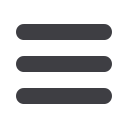

Conformational Ensembles from Experimental Data
and Computer Simulations
Poster Abstracts
110
75-POS
Board 35
Bridging the Gap Between Markov Stability Theory and Protein Dynamics Experiments at
All Timescales
Robert Peach
1
, David Klug
1
, Sophia Yaliraki
1
, Keith Willison
1
, Mauricio Barahona
2
, Liming
Ying
3
.
1
Imperial College, London, United Kingdom,
2
Imperial College, London, United Kingdom,
3
Imperial College, London, United Kingdom.
Objective: The hierarchy of timescales over which protein dynamics occurs is difficult to probe
both experimentally and computationally where motions cover approximately ten orders of
magnitude in timescale. We have developed Markov Stability, an atomistic graph theoretical
method that is able to explore protein dynamics across all of these temporal and spatial scales by
finding communities of atoms that are biologically relevant. Markov Stability is able to provide
information regarding the timescale of dynamics and has the ability to identify functionally and
dynamically important residues. We compare the computational results with single-molecule
FRET and Fluorescence correlation spectroscopy (FCS) to both test the computational
predictions and to calibrate a relationship between measured physical parameters and Markov
Stability.
Methods: The main methods used were Markov Stability (an atomistic graph theoretical
community detection method), single-molecule FRET and FCS on a confocal microscope. The
experimental system is Aquifex Adenylate Kinase, a well-studied and understood system and an
ideal proving ground for experimental tests of the Markov Stability method.
Results: Computational mutagenesis identified a number of residues that altered protein
dynamics and molecular stability. The key result was the straight line correlation between the
computational score of a mutation and the shift in population equilibrium as measured by single-
molecule FRET. Additionally we have found a correlation between Markov time and the
dynamical rates of subdomain motion obtained using FCS. Furthermore, a correlation between
melting temperatures and predicted scores were identified in the core domain.
Conclusions: We have experimentally validated the predictions from Markov Stability analysis
of Adenylate Kinase by showing a linear relationship between measured and calculated
parameters. In order to do this we successfully identified key functional residues through
computational mutagenesis providing a tool that can be used for protein engineering.