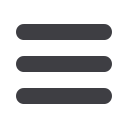

Conformational Ensembles from Experimental Data
and Computer Simulations
Poster Abstracts
86
51-POS
Board 11
Bayesian Ensemble Refinement by Replica Simulations and Reweighting
Juergen Koefinger
, Gerhard Hummer.
Max Planck Institute of Biophysics, Frankfurt am Main, Germany.
Ensemble refinement attempts to overcome the respective limitations of experiment and
simulations when it comes to the inference of structural ensembles of highly flexible and
dynamic macromolecules. Most experimental measurements provide averages over ensembles of
structures (NMR) and additionally often provide only low-resolution structural information
(SAXS/SANS, FRET, DEER). In contrast, bioinformatics and simulations can provide high-
resolution ensembles of structures but suffer from systematic and sampling uncertainties. In
replica simulations, experimental measurements can be used to steer simulations to explore
configurations space more efficiently. However, the number of replicas, and thus the maximum
size of the ensemble, is strongly limited by the available computational resources. In contrast,
reweighting allows us to adapt the statistical weights of large ensembles of structures efficiently
but it will fail if the overlap with the true ensemble is insufficient. By combining these two
approaches, we overcome their respective limitations. We show that replica simulations [Best
and Vendruscolo, J. Am. Chem. Soc. 2004] and the Ensemble Refinement of SAXS method
(EROS) [Rozycki et. al, Structure 2011] both aim to find the optimal solution of the same
underlying Bayesian formulation of ensemble refinement. In the Bayesian Inference of
ENsembles method (BioEn) [Hummer and Koefinger, J. Chem. Phys. 2015] we first perform
replica simulations to explore configurations space. From these simulations, we generate a
reference ensemble using a bin-free version of WHAM, which we then refine while striking a
balance between our prior knowledge, summarized in the reference ensemble, and the new
experimental data.