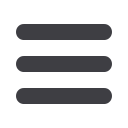

S324
ESTRO 36 2017
_______________________________________________________________________________________________
Despite higher symptoms’ burden after WBRT that are
attributed to the side effects of RT, like appetite loss,
drowsiness, and hair loss, QLQ-C30 global health status,
physical functioning and future uncertainty favored WBRT
in comparison with SRT-TB in our study. This may be
related to the compromised brain tumor control with
omission of WBRT; however, we should be aware that in
brain metastases patients many factors may influence
QoL.
PO-0627 Prediction of radiosurgery response of brain
metastases using convolutional neural networks
Y. Cha
1
, M.S. Kim
1
, C.K. Cho
1
, H. Yoo
1
, W.I. Jang
1
, Y.S.
Seo
1
, J.K. Kang
1
, E.K. Paik
1
1
Korea Institute of Radiological & Medical Sciences,
Department of Radiation Oncology, Seoul, Korea
Republic of
Purpose or Objective
A deep learning concept based on artificial convolutional
neural networks (CNN) is regarded as an emerging
radiomics methodology because it uses minimal amount of
image preprocessing. Metastatic brain tumor is presumed
an appropriate model for radiomics study because of
round shape and clear boundary of tumor. The purpose of
this study is to predict radiation response of metastatic
brain tumor receiving stereotactic radiosurgery (SRS) using
the radiomics model based on CNN.
Material and Methods
CNN is a kind of artificial neural network in which the
connectivity pattern between its neurons is inspired by the
organization of visual cortex. We implemented a CNN
system to process CT images using the numerical
computation library 'TensorFlow'. The 110 metastatic
brain lesions with longest diameter of 1-3.5 cm treated
with SRS between 2007 and 2015 were retrospectively
evaluated. Through the radiologic review within 3 months
after SRS, all lesions ware divided 2 groups: responder
(complete or partial response) and non-responder (stable
or progression) by Response Evaluation Criteria in Solid
Tumor (version 1.1). Responder and non-responder were
57 and 53 lesions, respectively. 110 data-sets which
composed of extracted images and matched response
classification were randomly assigned to 3 cohorts;
training, validation and evaluation cohort. And our CNN
system was trained by data-sets of training cohort. Then
the system was optimized by adjusting training
parameters using data-sets of validation cohort. After
sufficient training and optimization, a CNN system reliably
predicts classification of the arbitrarily inputted images.
We inputted images of evaluation cohort into the trained
CNN system. Then the system predicted the response
classification of inputted images. The above process was
repeatedly performed with changing the number ratio of
data-set in each cohort and the assignment of data-sets to
cohorts, respectively.
Results
The range of accuracy of prediction was elevated from 70%
to 83% as increasing the number of data-sets of training
cohort from 60 to 80. On 80 training data-sets, average
73% sensitivity and 83% specificity in predicting non-
responder were achieved.
Conclusion
CNN based metastatic brain tumor CT image training and
classification system was successfully implemented. The
prediction of early response after SRS to metastatic brain
tumor using the system was achieved effectively. To
improve the performance of CNN based prediction system,
the number of training data should be increased. This first
study of prediction of radiosensitivity using CNN provides
initial evidence of potential applicability of CNN based
radiomics method to clinical radiation oncology field.
PO-0628 Correlation between 18F-FDOPA uptake and
tumor relapse in recurrent high-grade gliomas
I. Chabert
1,2,3,4
, F. Dhermain
5
, S. Bibard
1
, S. Reuze
1,3,4
, A.
Schernberg
5
, F. Orlhac
4,6
, I. Buvat
6
, E. Deutsch
3,4,5
, C.
Robert
1,3,4
1
Gustave Roussy, Radiotherapy - Physics, Villejuif,
France
2
Institut Curie, Centre René Huguenin, St-Cloud, France
3
Univ. Paris-Sud, Université Paris-Saclay, Le Kremlin-
Bicêtre, France
4
INSERM, U1030, Villejuif, France
5
Gustave Roussy, Radiotherapy, Villejuif, France
6
IMIV, CEA- Inserm- CNRS- Univ. Paris-Sud- Université
Paris-Saclay- CEA-SHFJ, Orsay, France
Purpose or Objective
Patients suffering from high-grade gliomas have a median
survival time of 14 months despite various treatment
strategies. Our purpose was to investigate whether
18
F-
FDOPA PET imaging could predict tumor relapse areas and
improve tumor delineation in recurrent high-grade gliomas
treated by radio-chemotherapy.
Material and Methods
This prospective study started in 2015 included 8 patients
suffering from recurrent high grade gliomas (grade 4) who
received radiotherapy [from 40 Gy to 50 Gy in 2.5 or 4
Gy/fraction] associated with Bevacizumab chemotherapy.
Subjects underwent pre-treatment CT, T1-Gd, T2 FLAIR
acquisitions and a
18
F-FDOPA scan. All images were
registered to the planning CT using a rigid algorithm. One
senior radiotherapist delineated Gross Tumor Volumes
(GTV) on anatomical MR images. A large region of interest
was manually drawn around the first recurrence site on
the
18
F-FDOPA images and two thresholds t of 30% and 40%
of the maximum standardized uptake value (SUV
max
) were
applied to deduce the regions of high
18
F-FDOPA uptake
(V
PET,t
). Follow-up anatomical MR images were used to
localize second relapse areas (GTV’). Correlations
between all volumes were analyzed using five indexes. I
1,t
measures the percentage of V
PET,t
not included in the
anatomically-defined GTV. I
2
and I
3,t
respectively measure
the percentage of GTV’ included in GTV and V
PET,t
. I
4,t
measures the percentage of V
PET,t
included in GTV’. I
5,t
measures the percentage of V
PET,t
not included in the GTV
which was predictive of relapse. This index is meaningful
only
if
GTV’
and
GTV
are
different.
Results
Indexes obtained for each patient are presented in Table
1.
Six patients for whom relapse was confirmed anatomically
were included in the analysis. For 5 patients, I
2
was lower
than 40%, indicating a large progression of the tumor
outside the GTV. For 4 patients, I
1,30%
and I
1,40%
values were
between 69-82 % and 44-68 %, showing that additional
information was provided by
18
F-FDOPA images. I
3
values
rapidly decreased when the threshold t increased (30 % to
40%). For t = 30%, values were greater than 50 % for 3
patients. For these patients, I
4,30%
values were between 34