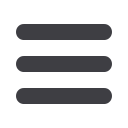

S864
ESTRO 36 2017
_______________________________________________________________________________________________
EP-1619 Determination of Lung Tumour Motion from
PET Raw Data used for Accelerometer Based Motion
Prediction
G. Hürtgen
1
, S. Von Werder
2
, V. Berneking
1
, K. Gester
1
,
O. Winz
3
, P. Hallen
4
, F. Büther
5
, C. Schubert
1
, N.
Escobar-Corral
1
, J. Hatakeyama Zeidler
6
, H. Arenbeck
6
,
C. Disselhorst-Klug
2
, A. Stahl
7
, M.J. Eble
1
1
RWTH Aachen University Hospital, Department of
Radiooncology and Radiotherapy, Aachen, Germany
2
Institute of Applied Medical Engineering RWTH Aachen
University, Department of Rehabilitation- & Prevention
Engineering, Aachen, Germany
3
RWTH Aachen University Hospital, Department of
Nuclear Medicine, Aachen, Germany
4
Institute for Experimental Molecular Imaging RWTH
Aachen University, Department of Physics of Molecular
Imaging Systems, Aachen, Germany
5
European Institute for Molecular Imaging EIMI,
University of Munster, Münster, Germany
6
Boll Automation GmbH, Research and Development,
Kleinwallstadt, Germany
7
RWTH Aachen University, III. Institute of Physics B,
Aachen, Germany
Purpose or Objective
For precise stereotactic radiation of lung tumours the
exact position of the tumour has to be known. A common
method for the detection of the tumour position is using
fluoroscopy during treatment. This leads to a very precise
tracking of the tumour position, but also causes additional
dose in the scanned region.
In this work an alternative solution to determine the
actual tumour position without additional radiation is
introduced. Combined information from FDG-PET scans
and an accelerometer based system are used for a patient
specific tumour movement prediction.
Material and Methods
We measured the breathing motions of ten patients in a
clinical trial by placing six tri-axial accelerometers on the
patient’s thorax and abdomen. Each patient is instructed
to breathe in up to five different breathing techniques:
‘free breathing’, ‘deep thoracic’, ‘flat thoracic’, ‘deep
abdominal’ and ‘flat abdominal’. Simultaneously, a FDG-
PET scan was performed to correlate the patient’s
respiratory states with the tumour positions afterwards.
Retrospectively the tumour trajectory was extracted from
the PET raw data and afterwards correlated with the
information obtained by the accelerometers. The
extraction of the respiratory motion was performed using
the
methods
described
in
[1]
and
[2].
A verification of the motion extraction algorithm was
performed with an in-house developed moving phantom.
Results
The measurements show a good agreement between real
and
reconstructed
phantom
motion.
An analysis of a 'deep abdominal' breathing is shown in
figure 1. The tumour trajectories are displayed in blue and
the low pass filter of the data in red. Combining the
information from the accelerometer system and the
tumour trajectories a model can be obtained to predict
the most likely tumour position for a given accelerometer
signal [3]. Figure 2 shows the tumour trajectory in
superior-inferior direction of a ‘free breathing’ instruction
in blue and the predicted trajectory in orange. The model
shows a good prediction of the real tumour trajectory.
Figure 1: Tumour trajectory (blue) and low pass filter (red)