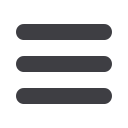

INFORMS Philadelphia – 2015
397
3 - Optimization of Active Traffic Management Strategies using Mixed
Integer Linear Programming
Joseph Trask, North Carolina State University, 909 Capability Dr,
Raleigh, NC, 27606, United States of America,
jltrask@ncsu.edu,
John Baugh, Nagui Rouphail, Behzad Aghdashi
In order to reduce congestion and improve travel times Active Traffic
Management (ATM) strategies are often used to better control flow on freeways.
This work presents a Mixed Integer Linear Programming formulation for the
oversaturated method of the Highway Capacity Manual. The model is then used
to optimize and provide insight into the usage of ATM strategies such as hard
shoulder running and ramp metering.
4 - Constrained Optimization for the Morning Commute Problem with
Flat Toll and Nonidentical Commuters
Xiaolei Guo, Associate Professor, University of Windsor, 834
Hacienda Court, Odette School of Business, Windsor, Canada,
guoxl@uwindsor.ca,Da Xu
We study the morning commute problem with a peak period flat toll on a single
bottleneck. Commuters’ values of time are assumed to be continuously
distributed. We consider that, for public acceptance reason, the toll has a
maximum acceptable toll level and a maximum acceptable length of tolling
period, both exogenously given. Under such a constrained optimization setup, we
investigate the problems of system cost minimization, Pareto improvement, and
revenue maximization.
5 - Research on the Decision Method of Concession Period for Bot
Highway Projects
Yinhua Yang, Huazhng University of Science and Technology,
Luoyu Road 1037,Hongshan District, Wuhan, China,
1143185408@qq.comPrivate provision of public roads through build–operate-transfer (BOT) contracts
is increasing around the world. In this paper, based on a flexible BOT contact with
demand updating, We build a decision model of Concession Period for Highway
BOT Projects to maximize social welfare and allow the private sector an
acceptable profit. Finally, we selects a BOT proiect of Guangfu highway for
concession period decision-making analysis.
WA72
72-Room 203A, CC
Design and Analysis of Computer Experiments
Sponsor: Quality, Statistics and Reliability
Sponsored Session
Chair: Ying Hung, Department of Statistics. Rutgers University,
yhung@stat.rutgers.edu1 - Modeling an Augmented Lagrangian for Improved Blackbox
Constrained Optimization
Robert Gramacy, The University of Chicago, 5807 S Woodlawn
Ave, Chicago, IL, 60605, United States of America,
rbgramacy@chicagobooth.eduWe propose a combination of response surface modeling, expected improvement
(EI), and the augmented Lagrangian (AL) numerical optimization framework,
allowing the statistical model to think globally and the AL to act locally. Our
hybridization presents a simple yet effective solution that allows existing
objective-oriented statistical approaches, like those based on Gaussian process
surrogates and EI, to be applied to the constrained setting with minor
modification.
2 - Empirical Orthogonal Function Calibration with
Simulator Uncertainty
Matthew Pratola, Assistant Professor, The Ohio State University,
1958 Neil Avenue, Columbus, OH, 43210,
United States of America,
mpratola@stat.osu.eduModel-assisted inference has become increasingly popular when predicting real-
world processes. Computer model calibration is a statistical framework combining
mathematical model simulators of the process with statistical techniques to
improve predictions by treating the simulator as deterministic. However, these
simulators are often uncertain. We develop a Bayesian approach to incorporate
such uncertainty using EOF’s, and map simulator uncertainty into inferences for
parameters and predictions.
3 - Challenges in Solar Power Forecasting Based on Multiple
Computer Models
Youngdeok Hwang, Research Staff Member, IBM Research,
1101 Kitchawan Road, Route 134, Rm 34-224, NY, 10603,
United States of America,
yhwang@us.ibm.comSolar energy forecasting based on large scale computer model needs to address
some unique challenges. Development of a forecasting system requires both the
accuracy and stability for large scale operation. Statistics has an important role in
quantifying uncertainty and providing guidance to decision-makers in the
market. I will discuss some of these unique challenges and research opportunities
in using complex computer models in solar energy industry.
4 - Multivariate Gaussian Process Model with Sparse
Covariance Estimation
Qiong Zhang, Virginia Commonwealth University, 1015 Floyd
Avenue, Richmond, VA, 23294, United States of America,
qzhang4@vcu.eduWe propose a method to obtain a sparse estimation of the covariance matrix for
multivariate Gaussian process model. We formulate the optimization problem as
the L1 penalized log-likelihood function. To solve the optimization problem, we
applied the majorization-minimization approach and the generalized gradient
descent algorithm. Numerical experiments are provided to show the
computational efficiency of the proposed method.
WA73
73-Room 203B, CC
Reliability Analysis of Complex Engineering Systems
Sponsor: Quality, Statistics and Reliability
Sponsored Session
Chair: Rong Pan, Associate Professor, Arizona State University, P.O. Box
878809, Tempe, AZ, United States of America,
rong.pan@asu.edu1 - Start-up Demonstration Tests with Sparse Connection
David Coit, Professor, Rutgers University,
coit@rci.rutgers.edu,Xiaoyue Wang, Xian Zhao
A start-up demonstration test is important for selecting equipment with high
start-up reliability. Based on the concept of sparse connection, three tests with
sparse connection are introduced which are called CSTF with sparse d1, TSCF
with sparse d2 and CSCF with sparse d3 and d4. By finite Markov chain
imbedding approach, probabilistic indexes are given. Besides, the procedure for
obtaining optimal parameters of tests is proposed and new tests have higher
efficiency than traditional ones.
2 - Bayesian Melding Methods for System Reliability Inference using
Multilevel Information
Zhaojun Li, Assistant Professor, Western New England University,
1215 Wilbraham Rd, Springfield, MA, 01119,
United States of America,
zhaojun.li@wne.edu,Jian Guo
This paper investigates Bayesian melding methods for the system reliability
analysis using various sources of test data and expert knowledge at both
subsystem and system levels. The adaptive Sample Importance Resampling (SIR)
method is proposed to address the computational challenges for updating the
subsystem and system level posterior reliability. System posterior reliability
assessments are compared under three scenarios of available subsystem and
system data and prior information.
3 - Early System Reliability Analysis for New Products using
Existing Components
Xiaoping Du, Professor, Missouri Science and Technology
University, 400 West 13th Street, Toomey Hall, Rolla, MO, 65409,
United States of America,
dux@mst.eduA new product may use existing components, and predicting its reliability is
difficult due to dependent components. This presentation discusses how to
address this issue. One way is to provide a narrow bound of the system reliability
by a physics-based approach. The other way is to require component designers
provide the relationship between the component reliability and the component
load. This allows for an accurate prediction of the system reliability.
4 - A Computational Bayesian Approach to Dependency Assessment
in System Reliability
Petek Yontay, Research Associate, Arizona State University,
699 S. Mill Ave., Tempe, AZ, 85281, United States of America,
pyontay@asu.edu, Rong Pan
Due to the increasing complexity of engineered products, it is of great importance
to use a model that can handle the complex reliability dependency among
components, subsystems and systems. We propose a Bayesian network approach
for assessing conditional dependencies within a complex system, using a
hierarchical setting. We estimate the posterior distributions of these conditional
probabilities in the Bayesian network by combining failure information at
component, subsystem and system levels.
WA73