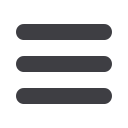

INFORMS Philadelphia – 2015
63
SA72
72-Room 203A, CC
Predictive Modeling and Control for
Additive Manufacturing
Sponsor: Quality, Statistics and Reliability
Sponsored Session
Chair: Qiang Huang, Associate Professor, University of Southern
California, GER 240, USC, Los Angeles, CA, United States of America,
qiang.huang@usc.eduCo-Chair: Arman Sabbaghi, Assistant Professor Of Statistics, Purdue
University, Department of Statistics, 150 N. University Street, West
Lafayette, IN, 47907, United States of America,
sabbaghi@purdue.edu1 - Bayesian Additive Modeling for Quality Control of
3D Printed Products
Arman Sabbaghi, Assistant Professor Of Statistics, Purdue
University, Department of Statistics, 150 N. University Street,
West Lafayette, IN, 47907, United States of America,
sabbaghi@purdue.edu, Tirthankar Dasgupta, Qiang Huang
Three-dimensional (3D) printing is a disruptive technology with the potential to
revolutionize manufacturing. However, control of product deformation remains a
major issue. Quality control requires a generic methodology that can predict
deformations for a wide range of designs based on data available for a few
previously manufactured products. We develop a Bayesian methodology to
update prior conceptions of deformation for a new design based on printed
products of different shapes.
2 - Predictive Modeling of in-plane Geometric Deviation for 3d
Printed Freeform Products
He Luan, University of Southern California, GER 236, USC,
Los Angeles, CA, United States of America,
hluan@usc.edu,
Qiang Huang
Although additive manufacturing holds great promise, dimensional geometric
accuracy remains a critical issue and lacks of generic solve method. Our work fills
the gap by establishing a general model predicting in-plane deviations of AM built
freeform products. Built upon our previous model for cylinder and polyhedron,
this work directly predicts freeform shape deviations from CAD design. SLA
experiments validated this method, indicating the prospect of optimal
compensation for freeform products.
SA73
73-Room 203B, CC
Data Analytics for Reliability Evaluation and
Maintenance Optimization I
Sponsor: Quality, Statistics and Reliability
Sponsored Session
Chair: Qingyu Yang, Assistant Professor, Wayne State University, 4815
4th street, Room 2167, Detroit, Mi, 48202, United States of America,
qyang@wayne.eduCo-Chair: Eunshin Byon, Assistant Professor, University of Michigan,
1205 Beal Avenue, Ann Arbor, MI, 48109, United States of America,
ebyon@umich.edu1 - Modeling of Degradation Data from Disjoint Time Intervals
Xiao Liu,
liuxiao@sg.ibm.comMotivated by real-life problems, this paper presents a statistical model for
degrdation data collected from disjoint time intervals (blocks). Within each block,
high-frequency degradation measurements are available. Of interest is the
extreme (i.e., maximum or minimum) of the degradation level within each
interval.
2 - A Generic Method for Analyzing Complex Data with Covariates
Haitao Liao, Associate Professor, The University of Arizona, The
University of Arizona, Tucson, AZ, 85716, United States of
America,
hliao@email.arizona.edu, Yiwen Xu, Neng Fan
In this research, we study an automated modeling approach to constructing
phase-type (PH) distributions via mathematical optimization and develop PH-
based models to analyze complex data with covariates.
.
3 - A Discrete Semi-markov Model to Determine Optimal Repair
Decisions for Trend-renewal Process
Ernie Love, Professor Emeritus, Simon Fraser University, 8888
University Drive, Burnaby, BC, v5a1r5, Canada,
love@sfu.ca,Qingyu Yang, Wujun Si
The failure and repair process of a repairable machine (system) is modeled as a
trend-renewal process permitting the modeling of imperfect repairs. The state of
such a system can be characterized by the real age of the system and the failure
count permitting the use of a two-state semi-Markov model to determine optimal
repair/replacement decisions. Threshold type policies are established. Failure data
from a cement kiln is used to demonstrate the approach.
4 - A Mixed Effect Kijima Model and Application in Optimal
Maintenance Analysis
Wujun Si, PhD Student, Wayne State University, Detroit, MI,
48202, United States of America,
wujun.si@wayne.edu,
Qingyu Yang
The Kijima model has been widely applied to analyzing repairable systems with
general repair efficiency. Most existing studies treat the repair efficiency as a fixed
value while it can vary among a series of repair actions. In this paper, we propose
a mixed effect Kijima model to characterize the variation of repair efficiency. An
SAEM algorithm is developed for model parameter estimation. Based on the
proposed model an optimal maintenance analysis is developed as a case study.
SA74
74-Room 204A, CC
IEEE T-ASE Invited Session: Manufacturing
Systems Automation
Sponsor: Quality, Statistics and Reliability
Sponsored Session
Chair: Jingshan Li, Professor, 1513 University Ave, Madison, WI,
53706, United States of America,
jli252@wisc.edu1 - A Quality Flow Model in Battery Manufacturing Systems for
Electric Vehicles
Feng Ju, Assistant Professor, Arizona State University, Tempe, AZ,
53705, United States of America,
jeffrey0930@gmail.comIn this paper, we present a flow model to analyze product quality in battery
assembly lines with 100% inspections and repairs for defective parts. A Markov
chain based model is introduced to analyze quality propagations along the battery
production line. Analytical expressions of final product quality are derived and
structural properties are investigated. A case study is presented to illustrate the
applicability of the method.
2 - Energy-efficient Production Systems through
Schedule-based Operations
Liang Zhang, University of Connecticut, 371 Fairfield Way UNIT
4157, Storrs, CT, 06269-4157, United States of America,
liang@engr.uconn.edu, Jorge Arinez, Stephan Biller,
Guorong Chen
Control of production operations is considered as one of the most economical
methods to improve energy efficiency in manufacturing systems. This paper
investigates energy consumption reduction in production systems through
effective scheduling of machine startup and shutdown. The theoretical methods
are applied through a case study in automotive paint shop operations.
3 - Adaptive Sensor Allocation Strategy for Process Monitoring and
Diagnosis in a Bayesian Network
Kaibo Liu, Assitant Professor, UW-Madison, 1513 University
Avenue, Madison, 53706, United States of America,
kliu8@wisc.edu, Xi Zhang, Jianjun Shi
This talk proposes a novel approach to adaptively reallocate sensor resources
based on online observations in a Bayesian Network model, which can enhance
both monitoring and diagnosis capabilities. The proposed method addresses two
fundamental issues in an integrated manner: when to reallocate sensors and how
to update sensor layout. Case studies are performed on a hot forming and a cap
alignment process to illustrate the performance of the proposed method under
different fault scenarios.
4 - Online Steady-state Detection for Process Control using Multiple
Change-point Models
Shiyu Zhou, Professor, University of Wisconsin-Madison,
Department of Industrial and Systems Eng, 1513 University
Avenue, Madison, WI, 53706, United States of America,
shiyuzhou@wisc.edu, Jianguo Wu, Yong Chen, Xiaochun Li
Steady-state detection is critical in process performance assessment, fault
detection and process automation and control. We proposed a robust on-line
steady-state detection algorithm using multiple change-point model and particle
filtering techniques. Extensive numerical analysis shows that the proposed new
method is more accurate and robust than the other existing methods.
SA74