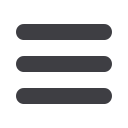

INFORMS Philadelphia – 2015
65
3 - Capacity Allocation with Demand Competition: Uniform,
Proportional, and Lexicographic Mechanisms
Niu Yu, Huazhong University of Science & Technology, 1037
Luoyu Road, Wuhan, China,
nyu@hust.edu.cn, Jianbin Li,
Zhixin Liu
We examine capacity allocation mechanisms in a two-echelon supply chain
comprising a monopoly supplier and duopoly retailers with asymmetric market
powers and demand competition.The supplier allocates limited capacity to
retailers according to uniform, proportional, or lexicographic mechanisms.Our
results show that regardless of whether retailer market powers are symmetric or
asymmetric, lexicographic allocation, regardless of order priority by retailer, is the
best choice for the supplier.
4 - Approximate Dynamic Programming and Real Options
Approaches for Sourcing Strategies under Risks
Purushottam Meena, Assistant Professor, NYIT, School of
Management, Old Westbury NY 11568, United States of America,
pmeena@nyit.eduThis paper demonstrates the use of real options for the valuation of different
sourcing strategies for supply disruptions risk management. The Approximate
Dynamic Programming (ADP) approach is used to solve the problem. The results
of ADP are compared with backward recursion to benchmark the performance of
ADP.
SA78
78-Room 301, CC
Managing Supply Chain Disruptions
Contributed Session
Chair: Hsin-Tsz Kuo, National Taiwan University, No.1, Sec. 4,
Roosevelt Road, Taipei, 10617, Taiwan - ROC,
d01741003@ntu.edu.tw1 - Topological Resilience Analysis of Supply Networks under
Random Disruptions and Targeted Attacks
Wenjun Wang, University of Iowa, S283 Pappajohn Business
Building, Iowa City, IA, 52242, United States of America,
wenjun-wang@uiowa.edu, Nick Street, Renato De Matta
We exploit the resilience embedded in the supply-network topology by
investigating the multiple-path reachability of each demand node to other nodes,
and propose a novel network resilience metric. We also develop new supply-
network growth strategies that reflect the heterogeneous roles of different types
of nodes in the network. We demonstrate the validity of our resilience metric and
experimentally show the effectiveness of our growth model.
2 - Post-disaster Disruptive Crop Supply Chain Resilience with
Stockpile Hoarding
Hsin-Tsz Kuo, National Taiwan University, No.1, Sec. 4, Roosevelt
Road, Taipei, 10617, Taiwan - ROC,
d01741003@ntu.edu.tw,Jiuh-biing Sheu
This paper presents a conceptual model to analyze the members’ opportunistic
behavior on post-disaster disruptive crop supply chain resilience. In this study, a
post-disaster supplier psychology theory is proposed to investigate the
antecedents of stockpile hoarding that may be revealed in a disruptive supply
chain. Moreover, we examine the decisions of the suppliers with government
intervention by selling stockpiled crops to the crop supply chain members for crop
market stabilization.
3 - Empirical Research on Supply Chain Resilience Factors
Jingjing Li, Student, Huazhng University of Science and
Technology, Luoyu Road 1037, Hongshan District, Wuhan, China,
1471058151@qq.comSupply chain resilience is an effective index to measure the ability of the supply
chain to deal with emergencies , including the ability to resist interruptions and
self-healing after emergencies . The conceptual model of supply chain resilience is
established on the basis of theory , including five factors. According to the
empirical study using SEM . The impact of various factors on the supply chain
resilience is quantized. It has substantial significance in improving supply chain
resilience.
SA79
79-Room 302, CC
Software Demonstration
Cluster: Software Demonstrations
Invited Session
1 - Microsoft Power BI – Power BI: Bring your Data to Life
Jen Underwood, Microsoft Senior Program Manager, Microsoft
In this tutorial session, you will explore Power BI with Microsoft engineering
product team members. Feel free to bring a sample of your own data to create
your own personalized dashboards. You will learn: • Data preparation: Discover,
transform and combine data from various sources. • Data models: Create
relationships, categorize your data and author business calculations. • Interactive
visual reports: Author professional reports to slice/dice data to discover insights.
• Power BI Dashboards: Ask language questions, create real-time dashboards and
share insights. • Power BI Mobile Apps: Interact with dashboards from iOS,
Android or Windows devices.
Sunday, 11:00am - 12:30pm
SB01
01-Room 301, Marriott
Network Mapping
Sponsor: Military Applications
Sponsored Session
Chair: Andrew Hall, COL, U.S. Army, 4760 40th St N, Arlington, VA,
United States of America,
AndrewOscarH@aol.com1 - Network Inference from Grouped Data
Charles Weko, Senior Reserve Manpower Analyst, US Army,
2812 Nobel Fir Court, Woodbridge, VA, 22192,
United States of America,
charles.w.weko.mil@mail.milIn many fields, network structure is not directly observed. Inferring implicit
network structure requires a probabilistic model of grouped data. Grouped data
records the manner in which a population forms subsets. In the existing
literature, network inference from grouped data is performed using descriptive
statistics. This presentation defines stochastic models for modeling group
formation and applies the parameters to the famous 18th century Chinese novel,
Dream of the Red Chamber.
2 - A Graph Comparison Approach to Network Attack and Defense
Jonathan Roginski, Applied Mathematics, NPS, Monterey,
United States of America,
jwrogins1@nps.eduThe promise of network science is to provide a foundation that supports
understanding the large, dynamic, complex networks that characterize life today.
In the Department of Defense, we create, manage, and defend against change in
these complex networks. However, the scientists and analysts providing decision
support are constrained by an acknowledged lack of tools for proper modelling of
network topology and quantification of network change. In this research, we
begin with the well-known problem of graph isomorphism and abstract it to the
idea of similarity between graphs, rather than graph “sameness.” We use structure
rather than statistics to quantify similarity between graphs by introducing a
matrix that captures more graph topological information than existing metrics or
descriptive statistics. We show that where current structural metrics fail, the
newly introduced distance-k matrix enables discrimination between graphs. We
also show a methodology through which a network may be “triaged” to identify
vertices which are potentially influential on the network’s topology. Those
candidate vertices are then analyzed using the distance-k matrix to determine the
“distance between graphs,” thereby quantifying the change in the network under
vertex removal. The result is a “target set” of vertices a decision maker can choose
from, based upon mission requirements, desired effect, and resources available-
supporting attack, defense, and stability operations.
3 - A Framework for Comparing Networks
Ralucca Gera, Naval Postgraduate School, Department of Applied
Mathematics, Monterey, CA, United States of America,
rgera@nps.eduA challenging problem in studying large networks is that networks data is
generally incomplete and often impossible to observe all members and
interactions within the network. Researches try to infer as much as possible of a
network and study its structure and function. Our approach is to create a ground
truth topology, infer the topology using several inference algorithms, and then
compare the inferred to the true topology, using synthetic and real networks.
SB01