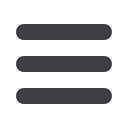

Single-Cell Biophysics: Measurement, Modulation, and Modeling
Poster Abstracts
65
41-POS
Board 21
The Correspondence between Raman Spectra and Total Omics
Koseki J. Kobayashi-Kirschvink
1
, Hidenori Nakaoka
1
, Arisa Oda
2
, Kunihiro Ohta
2,3
, Yuichi
Wakamoto
1
.
1
The University of Tokyo, Meguro-ku, Tokyo, Japan,
2
The University of Tokyo, Meguro-ku,
Tokyo, Japan,
3
The University of Tokyo, Bunkyo-ku, Tokyo, Japan.
Fluorescent reporters have been the de facto standard tool of
in vivo
research in the past two
decades, allowing us to monitor specific molecules in living cells with high precision. However,
the cell is a complex system with numerous molecules and interactions, and due to the specificity
of fluorescent reporters, they often fail to elucidate the cellular behavior as a whole. On the other
hand, omics techniques such as next generation sequencing or mass-spectrometry give us much
more comprehensive molecular information about the cell, but are inherently destructive. No
existing technique allows us to further analyze the dynamic molecular changes occurring at the
single-cell level.
In contrast, Raman micro-spectroscopy has advanced in recent years, and is one of the very few
imaging techniques that can potentially report on whole-cell molecular compositions at the
single-cell level in both comprehensive and non-destructive manners. However, the complexity
of Raman spectra has hampered its interpretation and thus its routine use. Previous attempts on
interpreting spectra mostly relied on preparing Raman spectral databases of purified materials,
which is usually time-consuming and laborious, especially when dealing with biological
samples. Here, we propose a method that can possibly circumvent the preparation of such
databases, and instead understand how Raman spectra and “total” omics correspond to each
other. Our method is based on the linear relationship between the two data sets that holds in
principle, and actively employs the intrinsic low-dimensionality of gene/protein expression
profiles. Example data of Raman spectra and mRNA-seq data of
Schizosaccharomyces
Pombe
under various stress conditions are obtained, and using supervised machine learning
algorithms such as the partial least squares regression, it is shown that if certain conditions are
met, mRNA-seq data can possibly be predicted from Raman spectra.