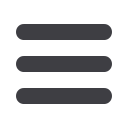

INFORMS Nashville – 2016
348
TD42
207D-MCC
RM in Online Markets
Sponsored: Revenue Management & Pricing
Sponsored Session
Chair: Dragos Florin Ciocan, INSEAD, Fontainebleau, France,
florin.ciocan@insead.edu1 - Learning Demand Curves In B2b Pricing: A Case Application Of
Optimal Learning
Ilya Ryzhov, Robert H. Smith School of Business, University of
Maryland,
iryzhov@rhsmith.umd.edu,Huashuai Qu, Michael Fu
We consider a sequence of B2B transactions involving a wide variety of buyers,
products, and other characteristics, where the seller only observes whether buyers
accept or reject the offered prices. The seller must adapt to this uncertain
environment and learn quickly from new deals as they take place. We propose a
new framework for statistical and optimal learning in this problem, based on
approximate Bayesian inference, that has the ability to measure and update the
seller’s uncertainty about the demand curve based on new deals. A case study
demonstrates the practical potential of this approach.
2 - Pricing Of Conditional Upgrades In The Presence Of Strategic
Consumers
Yao Cui, Cornell University, Ithaca, NY, United States,
yao.cui@cornell.edu, Izak Duenyas, Ozge Sahin
We study a conditional upgrade strategy that is recently used by the travel
industry. A consumer can accept an upgrade offer in advance and pay the
upgrade fee at check-in if higher-quality products are still available. Consumer
make forward-looking decisions regarding which product type to book. We
characterize the firm’s optimal upgrade pricing strategy and identify multiple
benefits of conditional upgrades. We also evaluate the revenue performance of
conditional upgrades by comparing to other policies.
3 - Revenue Management With Repeated Interactions
Dragos Florin Ciocan, INSEAD,
florin.ciocan@insead.edu,Andre
Du Pin Calmon, Gonzalo Romero
We consider an RM problem where a seller of heterogeneous goods interacts with
a dynamically evolving population of buyers over multiple periods. The basic
trade-off we explore is between myopically optimizing the seller’s revenues over
one period versus optimizing buyer surplus in the hope of increasing buyer
participation in future periods. We exhibit a simple policy that is asymptotically
optimal.
4 - Joint Pricing And Inventory Management With Strategic
Customers
Yiwei Chen, Singapore University of Technology and Design,
stevenyiweichen@gmail.com,Cong Shi
We consider a joint pricing and inventory management problem wherein a seller
sells a single product over an infinite horizon via dynamically determining
anonymous posted prices and inventory replenishment quantities. Customers
have a deterministic arrival rate but heterogeneous product valuations.
Customers are forward-looking, who can strategize their times of purchases. A
customer incurs waiting and monitoring cost if he delays his time of purchase.
The seller seeks a joint pricing and inventory policy that maximizes her long-run
average profit. We show that the optimal policy is cyclic. Under the optimal
policy, strategic customer equilibrium behaviors are proven to be myopic.
TD43
208A-MCC
Portfolio Decision Analysis
Sponsored: Decision Analysis
Sponsored Session
Chair: Janne Kettunen, The George Washington University, The George
Washington University, Washington, DC, 00000, United States,
jkettune@gwu.edu1 - Systematic Bias, Selection Bias, And Post-decision
Disappointment
Eeva Vilkkumaa, Aalto University, Helsinki, Finland,
eeva.vilkkumaa@aalto.fi,Juuso Liesiö
Based on empirical studies, the realized values of highest-ranked decision
alternatives tend to be lower than estimated. This phenomenon has been
explained by a systematic bias in the alternatives’ value estimates, or selection
bias. We develop models for estimating the relative magnitudes of these biases
using data on the estimated values of all alternatives but the realized values of
selected alternatives only. Results obtained from real data on 5,610 transportation
infrastructure projects suggest that out of the total cost overrun of $2.77 billion,
25% is due to systematic bias and 75% due to selection bias.
2 - Scheduling Public Procurement Contracting
Janne Kettunen, Assistant Professor, The George Washington
University, Washington, DC, United States,
jkettune@gwu.edu,
Young Hoon Kwak
We show that the schedule according to which procurements are contracted can
impact the number of proposals and thereby the cost of procured services or
products. To help the owner of the procurements to schedule the contracts, we
develop an optimization framework and apply it to the Florida Department of
Transportation’s procurement contracts scheduling problem. Our results show
that the optimal schedule yields about 2% ($15 million) cost savings annually.
3 - Multi-period, Multi-objective Portfolio Optimization
Ernest H Forman, George Washington University,
forman@gwu.eduMulti-Period, Multi-Objective and Multi-Perspective portfolio optimization
requires synthesis of hard data as well as judgment. The Analytic Hierarch Process
and extensions are increasingly being used to facilitate the portfolio optimization
process in a variety of applications, ranging from project portfolio optimization to
capital budgeting.
TD44
208B-MCC
Applications of Multiattribute Preferences
Sponsored: Decision Analysis
Sponsored Session
Chair: Jay Simon, American University, 4400 Massachusetts Ave NW,
Washington, DC, 20016, United States,
jaysimon@american.edu1 - Preference Programming For Spatial Multiattribute Decision
Analysis
Mikko Harju, Aalto University, Espoo, Finland,
mikko.harju@aalto.fi, Kai Virtanen, Juuso Ilari Liesio
The additive spatial value function models preferences among decision
alternatives with spatially varying multiattribute consequences. Use of this value
function can be challenging as it requires assessing a weighting function across
the (uncountably infinite) set of spatial locations. To overcome this challenge, we
develop (i) methods for capturing incomplete preference information about
relative importance of locations, and (ii) models for identifying the resulting
dominance relations among the alternatives. The use of these models is
demonstrated with a military planning application. Finally, we provide some new
insights about the axiomatic basis of spatial value functions.
2 - Multiattribute Preference Models For Computational Creativity
Debarun Bhattacharjya, IBM T. J. Watson Research Center,
Yorktown Heights, NY, United States,
debarunb@us.ibm.com, Lav
Varshney
There is vigorous debate around definitions of creativity, yet there is general
consensus that creativity inherently involves a subjective value judgment by an
evaluator. In this talk, I will present evaluation of creative artifacts and
computational creativity systems through a multiattribute preference modeling
lens. Various implications are illustrated with the help of examples from and
inspired by the creativity literature.
3 - Multilinear Utility Functions For Multiattribute Portfolio Decision
Analysis
Juuso Ilari Liesio, Aalto University, Helsinki, Finland,
juuso.liesio@aalto.fi, Eeva Vilkkumaa
In project portfolio selection applications, portfolio utility is often modeled as the
sum of the projects’ multi-attribute utilities. We establish the preference
assumption underlying this linear portfolio utility function. Furthermore, we
show how relaxing some of these assumption leads to a more general class of
multilinear portfolio utility functions, which can capture risk preferences on the
portfolio level. We also develop techniques to elicit these utility functions, and
optimization models to identify the project portfolio which maximizes the
expected utility subject to resource and other portfolio feasibility constraints.
4 - Multiattribute Procurement Auctions With Unknown Buyer
Preferences
Jay Simon, American University,
jaysimon@american.eduIn procurement auctions for new large-scale products or services, the length of
time between the request for bids and the selection of the winning bid can be
extremely long. During this time, the specific needs of the buyer may change.
Additionally, the new product or service being procured may involve technology
that is not fully understood. Thus, the buyer may not know what her eventual
preferences will be when the bid selection decision is made. However, the buyer
must tell the bidders how their bids will be scored at the start of the process. This
work explores optimal strategies for the buyer in the case where preferences at
the time of bid selection are uncertain when the scoring rule is chosen.
TD42