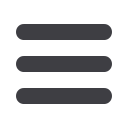

INFORMS Nashville – 2016
349
TD45
209A-MCC
Simulation Optimization and Ranking and Selection
Sponsored: Simulation
Sponsored Session
Chair: Demet Batur, University of Nebraska-Lincoln, CBA 209, Lincoln,
NE, 68588, United States,
dbatur@unl.edu1 - Optimization-based Learning Of Simulation Model Discrepancy
Henry Lam, University of Michigan, Ann Arbor, MI, United States,
khlam@umich.edu,Matthew Plumlee
The vast majority of stochastic simulation models are imperfect in that they fail to
fully emulate the entirety of real dynamics. Despite this, these imperfect models
are still useful in practice, so long as one knows how the model is inexact. We
propose a method to learn the amount of the model inexactness using data
collected from the system of interest. Our approach relies on a Bayesian
framework that addresses the requirements for estimation of probability measures
that are ubiquitous in stochastic simulation, and an embedded optimization to
enhance the involved computational efficiency.
2 - Quantile-based Ranking And Selection In A Bayesian Framework
Yijie Peng, Fudan University,
pengy10@fudan.edu.cn,Chun-Hung
Chen, Michael Fu, Jian-Qiang Hu, Ilya O Ryzhov
We propose two quantile-based ranking and selection schemes in a Bayesian
framework, i.e. myopic allocation policy (MAP) and optimal computing budget
allocation (OCBA). MAP has a superior small sample performance, while OCBA
shows a desirable asymptotic behavior. As a result, a switching strategy that that
switches from MAP to OCBA is provided to achieve balanced performances in
both small sample and large sample scenarios.
3 - Multi-information Source Optimization With Applications
Matthias Poloczek, Cornell University,
poloczek@cornell.edu, Jialei
Wang, Peter Frazier
We consider Bayesian optimization of an expensive-to-evaluate black-box
function, where we also have access to cheaper approximations of the objective
that are typically subject to varying unknown bias. Our novel algorithm
rigorously treats the involved uncertainties and uses the Knowledge Gradient to
maximize the predicted benefit per unit cost. We discuss applications and
demonstrate that the method consistently outperforms other state-of-the-art
techniques, finding designs of considerably higher objective value at lower cost.
4 - Tractable Dynamic Sampling Strategies For Quantile-based
Ordinal Optimization
Dongwook Shin, Columbia Business School,
dshin17@gsb.columbia.edu,Mark Nathan Broadie, Assaf Zeevi
Given a certain number of stochastic systems, the goal of our problem is to
dynamically allocate a finite sampling budget to minimize the probability of
falsely selecting non-best systems, where the selection is based on quantiles of
their performances. The key aspect is that the objective depends on underlying
probability distributions that are unknown. To formulate this problem in a
tractable form, we introduce a function closely associated with the
aforementioned objective. To derive sampling policies that are practically
implementable, we suggest a policy that combines sequential estimation and
myopic optimization, as well as certain variants of this policy for finite-time
improvement.
TD46
209B-MCC
Empirical Research on Pricing and Revenue
Management
Sponsored: Revenue Management & Pricing
Sponsored Session
Chair: Pnina Feldman, University of California-Berkeley, Berkeley, CA,
United States,
feldman@haas.berkeley.eduCo-Chair: Necati Tereyagoglu, Scheller College of Buss - Georgia
Institute of Technology, Atlanta, GA, United States,
necati.tereyagoglu@scheller.gatech.edu1 - Welfare Implications Of Congestion Pricing: Evidence from
SFpark
Hsin-Tien Tsai, University of California, Berkeley, 1822 Francisco
St., Apt 4, Berkeley, CA, 94703, United States,
hsintien@berkeley.edu, Pnina Feldman, Jun Li
SFpark is a congestion pricing program for street parking implemented in San
Francisco. We investigate whether consumers benefit from congestion pricing
using data from this program. We build a structural model of consumer search
and quantify the change in consumer welfare.
2 - Inventory Announcements And Customer Choice: Evidence From
The Air Travel Industry
Katherine Ashley, University of California-Berkeley,
kate_ashley@haas.berkeley.edu,Pnina Feldman, Jun Li
Does inventory announcement influence consumer decision-making in the
market for airline tickets? We estimate the impact of the firm’s announcement
policy on customer purchase timing and itinerary choice. In doing so, we measure
the information content of inventory announcements, and analyze the extent to
which customers treat these messages from the firm as cheap talk or credible
information.
3 - Designing Listing Policies For Online B2b Marketplaces
Wenchang Zhang, University of Maryland, COLLEGE PARK, MD,
CA, United States,
wzhang@rhsmith.umd.edu,Konstantinos
Bimpikis, Wedad Jasmine Elmaghraby, Kenneth Moon
Excess inventory amounts to $500 billion a year for big-box retailers. Much of
this inventory is sold through online B2B auctions. Based on a natural
experiment, we provide strong evidence that increasing the market thickness by
concentrating the auction ending times to just a couple of days of the week has a
significant positive effect on their final prices. We find that bidders’ monitoring
cost has a large impact on their auction entry choices and outweighs the
potentially negative effect of cannibalization among competing auctions. Our
findings may have implications for the design of online marketplaces beyond
liquidation auctions.
4 - Distribution Channel Relationships And Multimarket Competition
Necati Tereyagoglu, Assistant Professor of Operations
Management, Scheller College of Bussiness - Georgia Institute of
Technology, 800 W Peachtree St NW, Atlanta, GA, 30308, United
States,
necati.tereyagoglu@scheller.gatech.edu,O. Cem Ozturk
We study the role of the distribution channel relationships in determining
competitive intensity when manufacturers encounter in multiple markets. We
explore the manufacturers’ pricing decisions when they have asymmetric
distribution channel relationships with retailers across multiple markets. Using an
extensive scanner data set, we find that cross-market interdependence due to
shared ties with the retailers softens competition when manufacturers have
asymmetric distribution channel relationships across multiple markets.
TD47
209C-MCC
Cloud Computing in RM
Sponsored: Revenue Management & Pricing
Sponsored Session
Chair: Cinar Kilcioglu, Columbia Business School, Columbia Business
School, New York, NY, 10027, United States,
ckilcioglu16@gsb.columbia.eduCo-Chair: Costis Maglaras, Columbia University, New York, NY, United
States,
c.maglaras@columbia.edu1 - Optimal Resource Consumption via Data Driven Prophet
Inequalities With An Application To Cloud Infrastructure
Andrew A Li, MIT, Cambridge, MA, United States,
aali@mit.edu,Muhammad J Amjad, Vivek Farias, Devavrat Shah
Buyers of cloud compute resources are generally interested in completing
workloads by fixed deadlines as cheaply as possible. This entails purchasing
enough resources at the lowest prices possible, which is a challenge in today’s
market where the largest providers all use some form of demand-driven pricing.
We formulate this as a covering problem, and introduce the Data-Driven Prophet
Model, which uses historical price data to interpolate between stochastic
modeling and a fully adversarial model. We propose a simple, scalable threshold
policy that is order-optimal and has, in a real-world implementation, completed
workloads significantly cheaper than the current practice benchmark.
2 - Stochastic Optimal Control Of Time-varying Cloud Workloads
Mark S Squillante, IBM Thomas J. Watson Research Center,
mss@us.ibm.com,Yingdong Lu, Mayank Sharma, Bo Zhang
We consider a cloud computing system modeled as a GI/GI/1 queue under
workloads (arrival and service processes) that vary on one time scale and under
controls (server capacity) that vary on another time scale. Taking a stochastic
optimal control approach and formulating the corresponding optimal dynamic
control problem as a stochastic dynamic program, we derive structural properties
for the optimal dynamic control policy in general. We also derive fluid and
diffusion approximations for the problem and propose analytical and
computational approaches in these settings. Computational experiments
demonstrate the benefits of our approach.
TD47