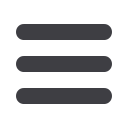

INFORMS Nashville – 2016
414
2 - IT Processes Improvement
Larisa Shwartz, IBM,
lshwart@us.ibm.comWith the advent of cognitive computing, new generations of cognitive systems
and services are being conceived. In IT service management, cognitive approaches
used for optimization and automation of IT Service management processes. We
discuss an integrated framework for problem resolution that enables an
automated discovery of informative phrases from IT incident tickets which are
later used to construct knowledge and facilitate automation of IT service
management. The effectiveness and efficiency of our framework are evaluated by
an extensive empirical study of a large scale real ticket data.
3 - Business-driven Optimization Of It Service Configuration In
Public And Hybrid Clouds Based On Performance Forecasting
Genady Grabarnik, St. John’s University, Queens, NY, Mauro
Tortonesi, Larisa Shwartz
Modern Cloud environments are rapidly evolving, leading to a growing adoption
of dynamic pricing for virtual resources and of speedier deployment tools and to
the emergence of hybrid Cloud scenarios. The need to address these challenges is
paving the way to a new generation of Cloud services, capable of adapting to
changes in their operating conditions and deployment environments by dynami-
cally realigning their configuration. However, their management will require
new and more sophisticated tools. This paper presents a new optimization solu-
tion for Cloud-based IT services, that tries to address these issues by using queu-
ing analysis of the services’ workflows and ILP optimization.
WB55
Music Row 3- Omni
Inventory Management VIII
Contributed Session
Chair: Yue Zhang, Duke University, 5507 Butterfly Ln Apt 207,
Durham, NC, 27707, United States,
yueyue.zhang@duke.edu1 - Deep Learning For Newsvendors
Afshin Oroojlooyjadid, Lehigh University, 200 West Packer Ave,
Bethlehem, PA, 18015, United States,
afo214@lehigh.edu,
Martin Taká , Lawrence Snyder
We study a newsvendor problem in which each demand observation also has a
set of features. We propose an algorithm based on deep learning that optimizes
the order quantity based on the features. It integrates the forecasting and
inventory-optimization steps, rather than solving them separately. The algorithm
does not require probability distributions. Numerical experiments on real-world
data suggest that our algorithm outperforms approaches from the literature,
including data-driven and SVM approaches, especially for volatile demands.
2 - Inventory Repositioning In Product Sharing Networks
Xiang Li, University of Minnesota, 2508 Delaware St SE,
Apt 473A, Minneapolis, MN, 55414, United States,
lixx1315@umn.edu, Saif Benjaafar, Xiaobo Li
We study a product sharing network in which customers can pick up a product
without reservation, and are allowed to keep the product for as long as they
want, without committing to a specific return time or location. We model the
periodic inventory re-positioning as a Markov decision process. We characterize
the qualitative properties of the optimal policy .
3 - Dynamic Inventory And Price Control In The Face Of
Unknown Demand
Tingting Zhou, Rutgers university, Newark, NJ, 07102, United
States,
tingzhou@rutgers.edu,Michael N Katehakis, Jian Yang
We study adaptive policies that combat unknown demand in a dynamic inventory
and price control setting. Inventory control is achieved by targeting newsvendor
ordering quantities for empirical demand distributions learned over time. On top
of that, demand-affecting prices are selected in a fashion that balances between
exploration and exploitation. When burdened with the task of selecting the most
profitable price, bounds for the regret can range between the orders of T1/2and
those of T2/3. Simulation studies are conducted as well.
4 - Approximating Optimal Inventory Policies For Assemble To Order
Manufacturing Systems
Levi DeValve, Duke University, 716 Turmeric Lane, Durham, NC,
27713, United States,
levi.devalve@duke.edu, Yehua Wei,
Sasa Pekec
We study the classical one-period assemble-to-order problem, modeled as a two
stage stochastic integer program with recourse. We leverage a primal-dual
approach to develop several approximation methods based on newsvendor
solutions. We identify co-monotone demand and symmetric hierarchy systems as
special cases where a component newsvendor solution is optimal under a
constant mark-up assumption, and provide closed-form bounds on sub-optimality
for more general cases. Further, we establish closed-form bounds for systems
where components serve many products and show asymptotic optimality.
5 - Serial Inventory Systems With Markov-modulated Demand:
Derivative Bounds, Asymptotic Analysis, And Insights
Yue Zhang, Duke University, 5507 Butterfly Ln Apt 207, Durham,
NC, 27707, United States,
yueyue.zhang@duke.edu, Li Chen,
Jing-Sheng Jeannette Song
We consider the inventory control problem for serial supply chains with
continuous, Markov-modulated demand. We perform a derivative analysis and
develop general, analytical solution bounds for the optimal policy. We further
derive a simple procedure for computing near-optimal heuristic solutions. We
next perform asymptotic analysis with long replenishment lead time. We show
that the relative errors between our heuristics and the optimal solutions converge
to zero as the lead time becomes sufficiently long, with the rate of convergence
being the square root of the lead time.
WB56
Music Row 4- Omni
Predictive Analytics in eBusiness
Sponsored: EBusiness
Sponsored Session
Chair: Ajit Sharma, Carnegie Mellon University, 5000 Forbes Ave,
Pittsburgh, PA, 15213, United States,
ajits1@andrew.cmu.edu1 - Online Assortment Optimization at Scale
Deeksha Sinha, MIT ORC,
deeksha@mit.edu, Theja Tulabandhula
We revisit the problem of assortment optimization in retail and consider a setting
where (a) the product universe is large, (b) there are time constraints in offering
an assortment, and (c) the user choice model can be personalized. In this setting,
we propose an offline algorithm based on the theory of locality sensitive hashing
(LSH) that computes approximately optimal assortments quickly. We also perform
a sensitivity analysis of the optimal assortment to the choice model used. We then
propose an online learning setup where we get feedback on the quality of
assortments offered in each round. We come up with new online algorithms
based on our offline solutions that can learn the user choice model quickly.
2 - Crowd-driven Competitive Intelligence: Understanding The
Relationship Between Local Market Structure And Online
Rating Distribution.
Dominik Gutt, Paderborn University,
Dominik.gutt@upb.de,
Philipp Herrmann, Mohammad Saifur Rahman
Crowdsourced information, such as online ratings, are increasingly viewed as a
critical source for understanding local market dynamics. A key aspect of utilizing
online ratings to derive competitive market intelligence is to delineate the
systematic relationship between local market structure and distributional
properties of online ratings. Using restaurant review data from
Yelp.comfor 372
isolated markets in the U.S., our empirical findings suggest that an increase in
competition leads to a broader range of ratings and to a decrease in the average
mean rating in a market. Moreover, we present evidence in support of both the
internal and external validity of Yelp’s crowdsourced online ratings.
3 - Networks And Income: Evidence From Individually Matched
Income And Mobile Phone Metadata
Guillaume Saint-Jacques, MIT Sloan School Of Management,
Cambridge, MA, 02142, United States,
gsaintja@mit.edu, Eaman
Jahani, Pål Roe Sundsøy, Johannes Bjelland, Bjørn-Atle Reme,
Sinan Aral, Alex “Sandy” Pentland
Measuring the relationship between income and various properties of one’s social
network has proven difficult because it requires data on income and social ties to
be matched at the individual level. We offer the first large-scale investigation of
this question using data that is both large scale and individually matched. How
are ego-networks different across income levels? Are there measurable differences
in degree, reciprocity, diversity and centrality? We use a dataset of Call Detail
Records from an Asian country of over 100M individuals and income surveys sent
to over 110,000 individuals. We use location data to control for location effects,
rather than rely on it to match incomes.
WB55