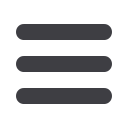

INFORMS Nashville – 2016
44
SB05
101E-MCC
Real Options in the Energy Sector
Sponsored: Energy, Natural Res & the Environment,
Energy I Electricity
Sponsored Session
Chair: Stein-Erik Fleten, Norwegian University of Science &
Technology, NO-7491 Trondheim, Trondheim, NO-7491,
Norway,
stein-erik.fleten@iot.ntnu.no1 - Switching From Oil To Gas Production – Decisions In A Field’s Tail
Production Phase
Kristian Støre, Norwegian University of Science and Technology,
Bodø, Norway,
krm@uin.no, Verena Hagspiel, Stein-Erik Fleten,
Claudia Nunes
We derive an optimal decision rule with regards to making an irreversible switch
from oil to gas production. Assuming that both the oil and gas prices follow a
geometric Brownian motion we derive a quasi-analytical solution for the exercise
threshold. We also derive the related abandonment option.
When comparing the use of a static decision rule to the proposed option approach
we show that the value loss can be substantial for the abandonment option. For
the switching option we find that with low gas prices the value loss can be more
substantial than for the abandonment option, while for high gas prices it may be
optimal to switch even as oil production is still generating positive cash flows.
2 - Resilience And Investment Valuation Of A Microgrid:
A Real Options Approach
Reinhard Madlener, Full Professor of Energy Economics and
Management, RWTH Aachen University,
E.ONEnergy Research
Center, FCN, Mathieustrasse 10, Aachen, Germany,
RMadlener@eonerc.rwth-aachen.de, Lisa Goebbels
In this study, microgrids are discussed as a possible decentralized system approach
to stabilize local power supply. Microgrids are a way to achieve a higher resilience
for a whole energy supply system. We introduce and empirically apply a
definition and quantification method for the resilience of a microgrid. Investment
feasibility of the installation of different combinations of components is evaluated
by adopting a real options approach for the optimal time to invest that takes the
uncertainty about future developments into account.
3 - Real Options In Renewable Portfolio Standards
Ryuta Takashima, Tokyo University of Science,
takashima@rs.tus.ac.jp, Makoto Goto
In order to promote renewable energy generation, the schemes as renewable
portfolio standards have been introduced in various countries. Thus the power
generators make investment decisions allowing not only for uncertain demands
and competitors’ strategies but also for the schemes. In this work we model an
equilibrium investment strategy of generators to analyze an effect of the schemes
on the investment in competitive electricity market. The market is composed of
non-renewable and renewable sectors. We show how the uncertainty affects the
investment timing for both generators with the scheme.
4 - Structural Empirical Analysis Of Hydropower Scheduling
Stein-Erik Fleten, Norwegian University of Science & Technology,
stein-erik.fleten@iot.ntnu.no, Maren Boger, Jussi Keppo,
Alois Pichler, Einar M Vestbøstad
Our goal is to study how price expectations are formed in an electricity market. In
the context of a single hydropower producer in the Nordic market, we expect the
forward curve to have a strong influence. The alternative we allow for is a
seasonal autoregressive joint inflow and spot price model that takes dry- and wet
year dynamics into account. Using observed time series of generation, reservoir
trajectories and technical plant data, and a structural model of optimal releases,
our initial findings indicate that forward prices have influence on price
expectations. An important byproduct of the proposed procedure is estimates of
marginal water values.
SB06
102A-MCC
Panel: What Industry Wants Analytics Graduates
to Know
Sponsored: Data Mining
Sponsored Session
Moderator: Thomas Tiahrt, The University of South Dakota, Beacom
School of Business, Rm 229, Vermillion, SD, 57069, United States,
Thomas.Tiahrt@usd.eduA panel discussion on What Industry Wants Analytics Graduates to Know
1 - What Industry Wants Analytics Graduates To Know
Panelist: Eric B Stephens, Vanderbilt University Medical Center,
eric.b.stephens@vanderbilt.edu2 - What Industry Wants Analytics Graduates To Know
Panelist: Sean T MacDermant, International Paper,
yorrie@macdart.com3 - What Industry Wants Analytics Graduates To Know
Panelist: Alkis Vazacopoulos, Optimization Direct, Inc.,
alkis@optimizationdirect.comSB07
102B-MCC
Data Mining in Decision Analytics:
Predictive Modeling in Theory and Applications
Sponsored: Data Mining
Sponsored Session
Chair: Ali Dag, Auburn University, Auburn, AL, United States,
azd0033@auburn.edu1 - A Novel Sentiment Analytic Methodology For Multinomial
Classification Of Product And Service Reviews
Ali Dag, Auburn University,
azd0033@auburn.eduThe objective of this study is to classify the customer reviews (on a five-star scale)
that were collected for 3 different product/service. To achieve this goal, a novel
classification framework is built by constructing a unique predictor, which
includes rich information gathered by using all of the extracted features. The
results indicate that the proposed method outperforms the alternatives.
2 - Probabilistic Decision Analytic Risk Level Prediction Model For
Kidney Transplants
Kazim Topuz, Wichita State University, Wichita, KS, United States,
mktopuz@gmail.com,Mehmet B Yildirim, Ferhat Zengul, Ali Dag
The objective of this study is to define risk levels and offer additional insights into
the factors affecting the short, medium and long-term success/failure of a kidney
transplant from deceased donor by using machine learning techniques. We
utilized an exhaustive variable selection algorithm to eliminate improper/noisy
variables by combining medical knowledge and mathematical models on large
pool of variables. Then we employed BBN to extract the hidden patterns and
relations between predictor variables as well as multi-class response variable.
3 - Ensemble Model With Cluster Analysis For Short-term
Stock Prediction
Bin Weng, Auburn University, Auburn, AL, 36849, United States,
bzw0018@auburn.edu, Fadel Mounir Megahed, Chen Li
The stock market is one of the most important ways for companies and
individuals to raise money due to the feature of publicity and high liquidity.
Accurately predicting stock market is extremely difficult due to the non-linear,
volatile and complex of the market. The purpose of this study is to develop a
model to predict stock’s short-term returns using disparate data sources from
online data, economic data, technical indicators, and traditional history data. This
study uses cluster analysis to cluster the trading days into different time periods
and ensemble machine learning methods to develop the models for each period.
As a result, the overall prediction performance has been increased.
SB05