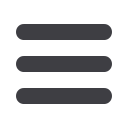

INFORMS Nashville – 2016
48
4 - Lockers Network: A Solution To Last Mile Delivery Problem
Guodong Lyu, National University of Singapore Business School,
#B1-01, BIZ2 Building, 1 Business Link, Singapore, 117592,
Singapre, 117592, Singapore,
guodong.lyu@u.nus.eduChung-Piaw Teo
Lockers (Parcel Collection Point) are convenient for parcels collection. To
maximize the coverage of failed delivery by using lockers, a novel locker location
model is introduced and customer choice is considered as an input. We provide
the mobile flow from stations to residence blocks, and use the mobility data to
locate lockers. The mobile delivery flow is calculated based on the transportation
data and failed delivery data. Customers’ parcel collection behavior is estimated
from locker usage data. Furthermore, lockers location data from an Express are
provided to validate our model. We demonstrate that the value of using mobility
date for failed delivery coverage maximization is significant.
SB16
105A-MCC
Algorithms for Stochastic Programming
Sponsored: Optimization, Optimization Under Uncertainty
Sponsored Session
Chair: Natashia L Boland, Georgia Institute of Technology,
755 Ferst Drive, NW, Atlanta, GA, 30332, United States,
natashia.boland@isye.gatech.edu1 - Scenario Set Partition Dual Bounds For Multi-stage
Stochastic Programming
Ilke Bakir, PhD Candidate, Georgia Institute of Technology,
Atlanta, GA, 30309, United States,
ilkebakir@gatech.eduNatashia Boland, Brian Dandurand, Alan Erera
We propose expected partition (EP) bounds, a hierarchy of bounds based on
partitions of the scenario set into subsets of (nearly) equal cardinality.
Additionally, using the fact that solution of group subproblems for all subsets in a
single partition also yields a dual bound, we establish that the best of even a very
small number of such single partition bounds is likely to be better than the
corresponding EP bound. By sampling partitions of the scenario set, we obtain an
algorithm that computes confidence intervals for an EP bound, while also
providing exact dual bounds. The practical value of these ideas is illustrated on
benchmark problems, and the approach is compared to Sample Average
Approximation.
2 - AMPL Representation And Solution Of Multiple Stochastic
Programming And Robust Optimization Formulations
Christian Valente, OptiRisk Systems, One Oxford Road, Uxbridge,
UB9 4DA, United Kingdom,
christian@optirisk-systems.com,
Gautam Mitra, Christiano Arbex Valle, Robert Fourer
Paradigms of ‘Uncertainty Models’, namely, recourse, chance constrained,
integrated chance constrained, and robust optimization models, are introduced
and represented through use case exemplars. Through our continued research
and close collaboration with AMPL Optimization we have now developed AMPL
templates and frameworks which can describe these classes of models and
connect them to respective solvers, making them readily available to industry-
based analysts and to the academic research community. We also describe an
E-book (in preparation) which captures the use cases that underpin our goal to
make these modelling and solving capabilities widely available to the OR
community.
3 - Combining Progressive Hedging And Frank-Wolfe To Solve The
Lagrangian Dual Of a Multistage Stochastic Integer Program
Natashia Boland, Georgia Institute of Technology,
natashia.boland@isye.gatech.edu, Jeffrey Christiansen,
Brian Dandurand, Andrew Eberhard, James Luedtke,
Jeffrey Linderoth, Fabricio Oliveira
We present a new primal-dual algorithm for computing the value of the
Lagrangian dual of a stochastic mixed-integer program (SMIP) formed by relaxing
its nonanticipativity constraints. The algorithm relies on the progressive hedging
method, but unlike previous progressive hedging approaches for SMIP, it
converges to the optimal Lagrangian dual value. The key improvement is an inner
loop of optimized linearization steps, as in the classical Frank-Wolfe method.
Numerical results show that the new algorithm outperforms the standard
progressive hedging approach.
4 - First Order Approximation Methods For Estimating Decision
Covariance In Stochastic Optimization
Sriram Sankaranarayanan, Johns Hopkins University,
3400, N. Charles St, Baltimore, MD, 21218, United States,
ssankar5@jhu.edu, Felipe A Feijoo, Sauleh Ahmad Siddiqui
We use first order methods to efficiently approximate the covariance matrix of the
optimal solution vector. The idea is extended for the covariance of the solution to
a complementarity problem by posing the complementarity problem as an
unconstrained minimization problem using the Fischer Burmeister merit
function. Having done this, we estimate the variability in the estimated Market
equilibrium of a Natural gas model, owing to uncertainty in the parameters of the
demand function.
SB17
105B-MCC
Optimization Challenges in Modeling
Sponsored: Optimization, Optimization Under Uncertainty
Sponsored Session
Chair: Leobardo Valera, University of Texas El Paso, 500 W. University
Ave, El Paso, TX, 79968, United States,
lvalera@miners.utep.edu1 - Problem Of Moments Over Log-concave Measures
Christopher Thomas Ryan, The University of Chicago, Chicago, IL,
United States,
chris.ryan@chicagobooth.edu,Simai He, Bo Jiang,
Teng Zhang
We explore the classical discrete problem of moments in a setting where the
underlying distribution are discrete log-concave. This situation arises in applied
problems such as reliabilit The challenge is that with log-concavity constraints,
the problem of moments becomes a non-convex optimization problem.
Nonetheless, we are able to characterize optimal extreme point solutions to such
problems. Our approach gives rise to improved bounds over the existing
literature, as well as analytical insight into the structure of extreme point optimal
solutions as geometric distributions.
2 - Interval Constraint Solving Techniques And Model Order
Reduction To Enhance The Solution Of Dynamic Systems
Leobardo Valera, The University of Texas at El Paso,
lvalera@utep.edu, Martine Ceberio
Many natural phenomena are dynamic systems, which can often be modeled as
nonlinear systems of equations. Major issues with solving such nonlinear systems
are that their dimension can be very large and that uncertainty, often present, is
tricky to handle. Reduced-Order Modeling (ROM) can address dimension issues,
via Proper Orthogonal Decomposition (POD) in particular. On the other hand,
interval constraint-solving techniques (ICST) allow to handle uncertainty and
ensure reliable results. In this presentation, we show how ICST can be embedded
into POD to address dimension and uncertainty.
SB18
106A-MCC
HPC in Optimization 1
Invited: High Performance Computing
Invited Session
Chair: Kibaek Kim, Argonne National Laboratory, 9700 South Cass
Avenue, Building 240, Lemont, IL, 60439, United States,
kimk@anl.govCo-Chair: Geoffrey Malcolm Oxberry, Lawrence Livermore National
Laboratory, P. O. Box 808, Livermore, CA, 94551, United States,
goxberry@gmail.com1 - Asynchronous Parallelization Of Decomposition Methods
Kibaek Kim, Argonne National Laboratory,
kimk@anl.govWe present an approximate and incremental bundle method based on dual
decomposition for stochastic mixed-integer programming (SMIP), where the
Lagrangian dual function is incrementally updated by approximate subgradients
as well as exact ones. We implemented the method in an open source parallel
solver DSP, taking advantage of asynchronous communications on HPC cluster
using MPI library. We present our computational results on several SMIP problem
instances.
SB16