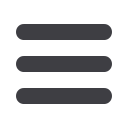

INFORMS Nashville – 2016
54
2 - A Model For Scheduling Practical Lessons And Selecting
Teaching Assistants At Universities
Cristian D Palma, Assistant Professor, Universidad del Desarrollo,
Avda. Sanhueza 1750, Pedro de Valdivia, Concepcion, 4040418,
Chile,
cristianpalma@ingenieros.udd.cl,Pablo Gonzalez,
Pamela Riffo
Most of the courses at universities includes practical sessions taught by teaching
assistants (TA), which are also students. These sessions are usually scheduled as
part of the courses, so the day and time when they are taught are known when
students register their courses. Since TAs have to attend their own courses, they
apply for teaching only in courses that match their own schedules rather than
courses they are good at. We propose a framework in which practical sessions are
scheduled after course registration, and show a model that schedules practical
sessions and simultaneously selects the TAs for each course. We discuss the
advantages of using this approach and present results of its application.
3 - Scheduling Virtual Network Embeddings
Frank Fischer, University of Kassel, Heinrich-Plett-Str. 40,
Kassel, 34132, Germany,
frank.fischer@mathematik.uni-kassel.de,
Andreas Bley
The virtual network embedding problem aims to embed several virtual network
(VN) requests, each consisting of several node and connection services that
require certain CPU, memory and bandwidth resources, into a shared physical
substrate network in such a way that the resources available in the substrate
network are not exceeded.
We consider the dynamic version of this problem, where VN requests have time
windows and durations specifying when and how long they should be embedded.
We discuss several mixed integer and constraint programming approaches for this
combined embedding and scheduling problem. Our computational results show
that a combination of both techniques performs best.
4 - An Improved Genetic Algorithm For Job-shop Scheduling
Mauricio G. C. Resende, Principal Research Scientist,
Amazon.com, Inc., 333 Boren Ave N, Seattle, WA, 98109,
United States,
resendem@amazon.comJosé F. Gonçalves
We present a local search, based on a new neighborhood for the job-shop
scheduling problem, and its application within a biased random-key genetic
algorithm. Schedules are constructed by decoding the chromosome supplied by
the genetic algorithm with a procedure that generates active schedules. After an
initial schedule is obtained, a local search heuristic, based on an extension of the
1956 graphical method of Akers, is applied to improve the solution. The new
heuristic is tested on a set of 205 standard instances taken from the job-shop
scheduling literature and compared with results obtained by other approaches.
The new algorithm improved the best-known solution values for 57 instances.
SB33
203B-MCC
Simulation and Optimization II
Contributed Session
Chair: Ryan Lawhead, Research Assistant to Dr. Gosavi, Missouri
University of Science and Technology, 223 Engineering Management
Building, Rolla, MO, 65409, United States,
rjlm97@mst.edu1 - A Study on The Operations Analysis Using Big Data Developed
by Simulations
Hongseon Park, University of Central Florida, Orlando, FL,
United States,
gauss1211@naver.com, Won Il Jung, Yong Bok Lee,
Gakgyu Kim, Gene Lee, Rabelo Luis
The operations analysis is analyzed under simulation circumstances. A lot of
assumptions and limited environments are included and, thus, the results have a
bias. To overcome this problem, we proposed a new methodology for the
operations analysis using Big Data developed by Virtual and Constructive (VC)
simulations. The VC simulations can produce a large volume and variety of data
since many variables are used for analyzing the operations close to reality by
using 6 Degrees of Freedom models. More than terabytes of data including
structured and unstructured types are applied to the current techniques of Big
Data. Then we will build the probability map and index to help the commanders
make decisions.
2 - Cloud Based Collaborative Information Sharing In Supply Chains
Cigdem Kochan, Assistant Professor, Ohio Northern University,
Dicke College of Business Administration, 525 S Main St, Ada, OH,
45810, United States,
cigdem.kochan@gmail.com,
David R. Nowicki
This research develops system dynamics models to simulate the effect of cloud
based collaborative information sharing in a supply chain. The results suggest that
the use of the cloud based information sharing in supply chain reduces inventory
levels, reduces actual lead time through demand and inventory visibility, and
reduces delivery delays while increasing overall performance of the supply chain.
3 - A Fully Exploratory Reinforcement Learning Algorithm For Solving
Semi-Markov Decision Processes
Angelo Encapera, Research Assistant, Missouri University of
Science & Technology, Rolla, MO, 65409,
United States,
amet3b@mst.edu,Abhijit Gosavi
We study the development of a fully exploratory Reinforcement Learning (RL)
algorithm for solving Semi-Markov Decision Processes (SMDPs). Existing RL
algorithms, such as R-SMART, for solving SMDPs require gradual decay of
exploration. The latter adds a tuning parameter to the algorithm, and indeed its
success depends on how the exploration-decay parameter is tuned. Our algorithm
uses a “reflective” update that accompanies the main update, based on relative Q-
Learning, to estimate the average reward without decaying the exploration. Our
algorithm delivers encouraging empirical behavior.
4 - A Bounded Actor Critic Algorithm For Reinforcement Learning
Ryan Lawhead, Research Assistant to Dr. Gosavi, Missouri
University of Science and Technology, 223 Engineering
Management Building, Rolla, MO, 65409, United States,
rjlm97@mst.edu,Abhijit Gosavi, Susan Murray
Actor-critic algorithms are amongst the oldest reinforcement learning algorithms
that can be used to solve Markov decision processes (MDPs) via simulation.
Unfortunately, the values of the “actor” in the classical version of this algorithm
get unbounded in practice. In practice, the actor’s values are artificially
constrained to obtain solutions. Boltzmann action selection is used for this
algorithm in which a temperature is used, but the convergence of the algorithm is
guaranteed only when the temperature equals 1. We propose a new actor-critic
algorithm in which the actor’s values are bounded even when the temperature is
set to 1. Our algorithm delivers encouraging numerical behavior.
SB34
204-MCC
Data-Driven Decisions in Healthcare
Sponsored: Manufacturing & Service Oper Mgmt, Healthcare
Operations
Sponsored Session
Chair: Vishal Ahuja, Southern Methodist University, Dallas, TX,
United States,
vahuja@smu.edu1 - Optimizing Cancer Prevention Strategies For BRCA1/2
Mutation Carriers
Elisa Frances Long, Assistant Professor, UCLA Anderson School of
Management, Los Angeles, CA, United States,
elisa.long@anderson.ucla.edu, Eike Nohdurft, Stefan Spinler
BRCA1/2 mutation carriers face significantly elevated lifetime risks for breast and
ovarian cancer. Prophylactic surgery (bilateral mastectomy, oophorectomy, or
both) can reduce the risk of cancer but may impact health utility. We developed a
Markov decision process model to determine the optimal age to undergo surgery
to maximize quality-adjusted life years or survival. Key state variables include
age, mutation type, breast cancer stage and sub-type, ovarian cancer stage, and
preventive surgery status. We solve the model with linear programming and
compute the optimal policy for different parameter settings reflecting varying
mutation carrier’s preferences.
2 - Predictive Models For Making Patient Screening Decisions
Michael Hahsler, Southern Methodist University, Dallas, TX,
United States,
mhahsler@lyle.smu.edu,Vishal Ahuja,
Michael Bowen
A critical dilemma that clinicians face is when and how often to screen patients
who may suffer from a disease. The stakes are heightened in the case of chronic
diseases that impose a substantial cost burden. We investigate the use of
predictive modeling to develop optimal screening conditions and assist with
clinical decision-making. We use electronic health data from a major U.S. Hospital
and apply our models in the context of screening patients for type-2 diabetes, one
of the most prevalent diseases in the U.S. and worldwide.
3 - Belief Perseverance And Experience
Bradley R Staats, University of North Carolina at Chapel Hill,
bstaats@unc.edu,Diwas S KC, Francesca Gino
Many models in operations management involve dynamic decision making that
assumes optimal updating in response to information revelation. However,
behavioral theory suggests that rather than updating their beliefs, individuals may
persevere in their prior beliefs. We examine how individuals’ prior experiences
and the experiences of those around them alter their belief perseverance in
operational decisions after the revelation of negative news using a field
experiment and two lab experiments.
SB33