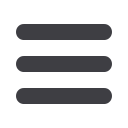

S126
ESTRO 36 2017
_______________________________________________________________________________________________
Purpose or Objective
In order to develop a more efficient workflow and to
achieve RTT-independent plans for a portion of breast
cancer treatments (whole breast radiotherapy with
optional boost irradiation), a fully automated planning
process has been clinically introduced. The process offers
a number of alternative treatment plans to the RTT, who
can choose to either select the optimal clinical plan, or
improve one of the candidate plans to a clinically
acceptable level. We investigated the acceptance rate of
automatically created plans and the motivations for
rejection and/or adaptation of these plans.
Material and Methods
From November 2015 to September 2016 657 treatments
have been planned using the automatic procedure. The
plans consist of medial and lateral tangential beams, with
an optional IMRT beam to deliver a boost dose to the
primary tumour (bed) (Fig.1). The tangential beams
consist of an open segment, delivering 75% of the dose,
and a limited number of IMRT segments, delivering 25% of
the dose. The open segments target the PTV (blocks on
the heart when applicable), but are open outside the
patient contour to allow for anatomical changes. 6MV and
10MV medial and lateral beams are offered. A heart
clearance choice of 0 mm or 5 mm is also offered. This
results in a total of 4 (right-sided breasts) or 8 (left-sided
breasts) candidate plans. The in-house automatic planning
software (FAST), controlling the Pinnacle
3
TPS, generates
the plans and corresponding dose distributions
automatically without any intervention from the RTT. This
procedure commences as soon as the radiation oncologist
has delineated the target volume.
Results
Automatically generated plans were selected by the RTT
without any adaptation in 54% of non-boost treatments
and in 41% of boost treatments (Fig.2). For both classes,
reasons for not selecting an automatically generated plan
were very similar: in 45% of the cases, optimization goals
were modified in order to change trade-offs between PTV
coverage and OAR doses (at the discretion of the RTT). Our
study found that in most of these cases the plan only
marginally differed from the automatic plan. In another
40% of the cases a new plan was manually created, e.g. to
replace the automatic tangential beam set-up with a more
favourable set-up. The final 15% comprised cases in which
automatic delineation was erroneous or other technical
issues. In the majority of left-sided cases, the 5mm heart
clearance plan was preferred. The variety in chosen beam
energies is related to patient geometry.
Conclusion
Considering that in close to 50% of all cases one of these
plans was accepted for clinical use, a significant time
saving is apparent (pre-clinical evaluation predicted an
acceptance rate of 60%). This saving is estimated to be
1000 hours/year based on the projected 800
patients/year. In 45% of the cases in which an automatic
plan was not chosen, only minor modifications were made
to the plan, still resulting in a time reduction close to 450
hours/year.
OC-0253 Machine Learning-Based Enables Data-driven
Radiotherapy Treatment Planning Decision Support.
G. Valdes
1
, L. Wojtowicz
2
, A.J. Pattison
3
, C. Carpenter
4
,
C. Simone
2
, A. Lin
2
, T. Solberg
1
1
University of California UCSF, Radiation Oncology, San
Francisco CA, USA
2
University of Pennsylvania, Radiation Oncology,
Philadelphia, USA
3
Siris Medical, CTO, Mountain View, USA
4
Siris Medical, CEO, Mountain View, USA
Purpose or Objective
Due to the complexity of dose deposition and variety of
treatment delivery technology, plan outcomes remain
non-intuitive. The ability to predict radiotherapy
treatment discrete plan outcomes before planning enables
the clinician to more accurately guide therapy decisions
before engaging in the time-consuming plan creation
process. We demonstrate the ability to accurately predict
plans for lung photon and for head and neck proton and
photon therapy using machine learning.
Material and Methods
100 patients with early stage lung cancer who received
stereotactic body radiation therapy (SBRT) and 36 patients
with head and neck cancer who received postoperative
proton radiotherapy were identified. Each head and neck
patient also had corresponding photon-based volumetric
modulated arc therapy plan (VMAT). DICOM-RT datasets
were processed using commercial plan-prediction
software (QuickMatch™, Siris Medical Inc.) to predict dose
to Planning Target Volumes (PTVs) and Organs at Risk
(OARs). For lung SBRT plans, we predicted doses to the
lung, cord, brachial plexus, skin, esophagus, heart, great
vessels, trachea, rib, chestwall, and PTV. For H&N plans,
we predicted doses to the: parotid, submandibular[AL1] ,
brain stem, cord, optic nerve, mandible, constrictors,
esophagus, oral cavity, and larynx. We computed error
metrics and established guidelines for dataset size. In
addition, several deliverable plans were created to
demonstrate the advantages of predictive modeling.
Results
We were able to effectively predict dose distributions and
dataset sizes required for desired accuracy errors for the
OARs and PTVs for both photon and proton treatments. For
lung SBRT plans, a dataset size of at least 69 plans resulted
in all mean errors below 2.5Gy. For photon H&N plans, a
dataset size of at least 121 plans resulted in all mean