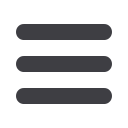

S304
ESTRO 36 2017
_______________________________________________________________________________________________
as identifying patients' care needs. With the increase in
long-term remote follow-up of patients PROMs play an
important role as they offer the opportunity to assess and
address the health concerns or health-related quality of
life (HRQOL) issues of individual patients [1]. Other
important clinical applications of PROMs include aiding
treatment choices as well as identifying high risk patients
who may have poorer long-term health-related outcomes
[2, 3]. These are all key challenges of modern oncology,
and PROMs play a strategic role in this as they enable the
tailoring of treatments according to the priorities, risks or
concerns of individual patients.
The successful application of PROMs requires a deeper
understanding of the methods for extracting information
carried within PROMs [4]. PROMs data are complex, with a
large number of variables (HRQOL, symptoms, function,
bother, performance or health concerns) measured on
different scales (with different levels, ratios or
frequencies). Symptom clusters are groups of 3 or more
correlated symptoms that occur together, and this is
stable over time [5, 6]. Symptom clusters can be easily
determined specifically to each dataset or clinical trial
[7]. This can be used as a method of grouping symptoms
for the purpose of summarising PROMs and extracting
meaningful information. The advantage of exploring
symptom clusters within a dataset is that it allows a study
specific method of grouping symptoms. Because of this
symptom clusters have the potential to improve sensitivity
and specificity to symptom grouping. Only items that are
strongly correlated, and so measure the same underlying
health concern, are included in summative scores. This
can be utilised in PROMs data modelling or clinical decision
making.
In clinical trials PROMs are often seen as a research tool
and it can be challenging to deliver real-time clinical
applications. PROMs can be difficult for patients to
complete, and missing data is another common problem
when analysing and interpreting PROMs [8]. Some of the
causes of missing data include the complexity of long and
multiple PROMs questionnaires, lack of feedback following
the delivery of PROMs, difficulty in understanding
questions or language issues (potentially associated with
migration), patients missing their appointments or
dropping-out of studies, and intermittent missingness
when patients fail to complete some of the questions. All
this contributes to the degree of missing data and in turn
a reduction in sample size, limited analytical applications
or even the risk of biased results. As treatments evolve
and the characteristics of patient populations change,
study specific approaches to analysing PROMs are
warranted. The correlation and grouping of items, missing
data, and the ceiling or floor effect in collected data
should all be investigated for each study when interpreting
and analysing PROMs. This may advance PROMs data
analysis and lead to the extraction of more relevant and
meaningful information.
1. Horwitz EM, Bae K, Hanks GE, Porter A, Grignon DJ,
Brereton HD et al. Ten-Year Follow-Up of Radiation
Therapy Oncology Group Protocol 92-02: A Phase III Trial
of the Duration of Elective Androgen Deprivation in Locally
Advanced Prostate Cancer. Journal of Clinical Oncology.
2008;26(15)
2. Weldring T, Smith SMS. Patient-Reported Outcomes
(PROs) and Patient-Reported Outcome Measures (PROMs).
Health Services Insights. 2013;6
3. Warrington L, Absolom K, Velikova G. Integrated care
pathways for cancer survivors - a role for patient-reported
outcome measures and health informatics. Acta Oncol.
2015;54(5)
4. Faithfull S, Lemanska A, Chen T. Patient-reported
Outcome Measures in Radiotherapy: Clinical Advances and
Research Opportunities in Measurement for Survivorship.
Clin Oncol. 2015;27(11)
5. Aktas A. Cancer symptom clusters: current concepts and
controversies. Curr Opin Support Palliat Care. 2013;7(1)
6. Dodd MJ, Miaskowski C, Paul SM. Symptom clusters and
their effect on the functional status of patients with
cancer. Oncol Nurs Forum. 2001;28(3)
7. Skerman HM, Yates PM, Battistutta D. Multivariate
methods to identify cancer-related symptom clusters. Res
Nurs Health. 2009;32(3)
8. Gomes M, Gutacker N, Bojke C, Street A. Addressing
Missing Data in Patient-Reported Outcome Measures
(PROMS): Implications for the Use of PROMS for Comparing
Provider Performance. Health Econ. 2016;25(5)
Symposium: Hypofractionation in prostate cancer
SP-0583 Moderate hypofractionation in prostate
cancer: what have we learnt from phase 3 trials
D.P. Dearnaley
1
1
Institute of Cancer Research, Academic Radiotherapy,
London, United Kingdom
Evidence has accumulated suggesting that prostate cancer
(PCa) may be particularly sensitive to radiation fraction
size. This has considerable implications for the delivery
of radical radiation treatments suggesting that shorter
treatments using higher dose/fraction schedules might
improve the therapeutic ratio and make treatment more
convenient for patients as well as using radiotherapy
resource more effectively. Four large randomised
controlled trials testing modest hypofractionation for
localised PCa have reported efficacy and side effect
outcomes within the last year
(1-4)
. The largest trial,
CHHiP, which included 3216 patients compared standard
fractionation (SFRT) using 2.0Gy daily fractions (f) (total
dose 74Gy) with two experimental hypofractionated
(HFRT) schedules using 3.0Gy/f (total doses of 60Gy and
57Gy)
(1)
. The trial used a non-inferiority design and
demonstrated that HFRT at 60 Gy was non–inferior to
SFRT. Five year disease control rates defined by
biochemical (PSA)/clinical failure free outcome were for
HFRT (60Gy) 90.6% (95% confidence intervals 88.5 - 92.3)
compared with SFRT 88.3% (86.0 - 90.2) (hazard ratio 0.84,
(95% CI: 0.65 – 1.07)); treatment related toxicities were
low and similar. A complementary study design was used
in the PROFIT trial
(2)
which included 1206 patients and
compared SFRT using 2.0Gy/f (total dose 78Gy) with the
same HFRT schedule of 3.0Gy/f (total dose 60Gy). HFRT
was again shown to be non-inferior to SFRT with identical
21% biochemical/clinical failure rates at 5 years. In
PROFIT gastro-intestinal side effects were increased in the
SFRT group compared with HFRT group probably due to
the higher SFRT dose given compared with
CHHiP. Intensity modulated radiotherapy methods (IMRT)
using either forward or inverse planning with a 3 part
simultaneous integrated boost were used in all patients in
the CHHiP trial. IMRT/IGRT methods were used in the
PROFIT trial. A key difference between the trials was the
use of 6 months neoadjuvant androgen deprivation
therapy (ADT) in CHHiP whilst RT alone was used in PROFIT
which probably explains the 11% higher biochemical
control rate in CHHiP. Both investigator groups suggested
that HFRT (60Gy/20f in 4 weeks) could be considered a
new standard of care. In contradistinction authors of the
HYPRO study
(3)
came to different conclusions testing dose
escalated HFRT. 804 patients received either SFRT 78Gy
in 2Gy daily fractions or HFRT giving 64Gy in 3·4Gy
fractions but importantly treating with three fractions per
week and therefore protracting overall treatment time
(OTT). The gain in tumour control was smaller than might
have been expected from radiobiological models (HFRT
80.5% vs. CFRT 77.1%) and not statistically significant. The
relatively unfavourable side effect profiles may be due to
the higher HFRT doses delivered. The trial failed to