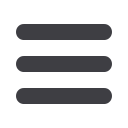

S816
ESTRO 36 2017
_______________________________________________________________________________________________
maximum dose to OAR located inside the brain PTV were
set to avoid hot spots. After that, the rest of the
optimization was carried out using the automatic
“Intermediate
Dose
Calculation”
option.
Various dosimetric parameters and indices were
employed: PTV mean dose; D
1cc
, D2% and D98%; mean and
maximum doses for OAR; V5 and V20 for lungs; conformity
(CI=Vol
95%
/Vol
PTV
) and homogeneity (HI=D
1cc
/D
prescr
)
indices; Normal Tissue mean and V5 dose.
Results
We found avg. values for CI, 1.02 (0.98-1.09) and for HI,
1.08 (1.05-1.10) regardless of D
prescr
. OAR mean dose
values along with average values reported by different
authors are shown in Fig. 1. A great reduction is observed
for almost all OAR for 23.4 Gy, while for 36 Gy our results
are favorable for eyes and lenses and similar or slightly
poorer to published ones for the rest of OAR, this may be
due to the fact that the majority of our cases with 36 Gy
were children which are precisely the more complex cases
to
optimize.
Dosimetric parameters dependent on D
prescr
are presented
in
Table 1.
Conclusion
A RapidArc planning process for craniospinal axis
irradiation has been implemented with significant
improvement on conformity, homogeneity, feasibility and
efficiency.
EP-1539 Parameters for estimating and controlling
small gaps in VMAT treatments
J. Saez Beltran
1
, V. Hernandez Masgrau
2
1
Hospital Clinic i Provincial, Radiation Oncology
Department, Barcelona, Spain
2
Hospital Sant Joan de Reus, Medical Physics
Department, Reus, Spain
Purpose or Objective
It is well established that dose modelling and calculation
of small fields and small MLC gaps is challenging. Indeed,
VMAT plans with a large fraction of small MLC gaps are
prone to present dosimetric discrepancies due to
limitation of the TPS and uncertainties in treatment
delivery. On the other hand, there is a growing interest in
developing tools to characterize robust class solutions for
plans. Our goal was to study leaf gap distributions in terms
of descriptive variables and complexity indices.
Material and Methods
A total of 276 RapidArc plans optimized with Eclipse v13
were exported in DICOM format for this study comprising
all locations (HN, prostate, gyne, brain, other). The
cumulative histogram of leaf gaps for all plans is shown in
figure 1. A set of MATLAB routines was developed to
perform the analysis. The following parameters were
computed: Total Modulation Index (MIt) (Park et, al),
Modulation Complexity Score (MCS) (McNiven et. al), Beam
Irregularity (BI) (Du et. al), mean gap, median gap and
MU/Gy. All these parameters were evaluated as predictors
of the fraction of small gaps, in particular fraction of gaps
smaller than 20, 10 and 5 mm.
Results
There was a very large variability in the distribution of MLC
gaps involved in VMAT plans. A strong exponential
relationship between the median gap and the fraction of
gaps smaller than 5, 10 and 20 mm was observed (r
2
=0.8,
0.92 and 0.95) (see figure 2). A similar but weaker
relationship was observed for the mean gap (r
2
=0.74, 0.87
and 0.94), the MCS (r
2
=0.55, 0.58 and 0.54) and MU/Gy
(r
2
=0.21, 0.26 and 0.25). Neither the MIt nor the BI
presented a relationship with any of the gap fractions
studied.
For median gaps > 30mm, the fraction of gaps lower than
5, 10 and 20 mm was estimated as 0.13 ± 0.07, 0.20 ± 0.06
and 0.36 ± 0.07, respectively. On the contrary, for median
gaps ~10mm, the fraction of gaps lower than 5, 10 and 20
mm was estimated as high as 0.33 ± 0.07, 0.50 ± 0.06 and
0.72 ± 0.07, respectively.