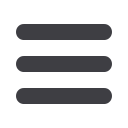

S906
ESTRO 36 2017
_______________________________________________________________________________________________
the obtained results with ImageJ, was implemented in
Moddicom, an open-source software developed in our
Institution to perform radiomic analysis.
Fractal analysis was performed applying the Box Counting
method on T2-weighted images of magnetic resonance.
The FD computation was carried out slice by slice, for each
patient of the study: values regarding mean, median,
standard deviation, maximum and minimum of the FD
distribution were considered as fractal features
characterizing the patient.
Fractal analysis was moreover extended on sub-
populations inside GTV, defined by considering the pixels
whose intensities were above a threshold calculated as
percentage of the maximum intensity value occurred
inside GTV. A logistic regression model was then
developed and its predictive performances were tested in
terms of ROC analysis. An external validation, based on 25
patients provided by MAASTRO clinic, was also performed.
The details on imaging parameters adopted are listed in
table 1.
Results
The predictive model developed is characterized by 3
features: the tumor clinical stage, the entropy of the GTV
histogram (calculated after the application of a Laplacian
of Gaussian filter with σ=0.34 mm) and the maximum FD
(maxFD) calculated for the sub-population whose
intensities are higher than 40% of the GTV maximum value.
MaxFD is the most significant parameter of the model:
higher maxFD value, typical of a more complex structure,
is correlated with less pCR probability. The model
developed showed an AUC of ROC equal to 0.77± 0.07. The
model reliability has been confirmed by the external
validation, providing an AUC equal to 0.80 ± 0.09.
Conclusion
Fractal analysis can play an important role in Radiomics:
the fractal features provide important spatial information
not only about the GTV structure, but also about its sub-
populations.Further investigations are needed to
investigate the spatial localization of these sub-
populations and their potential connection with biological
structures.
EP-1684 Optimal window for assessing treatment
responsiveness on repeated FDG-PET scans in NSCLC
patients
M. Lazzeroni
1
, J. Uhrdin
2
, S. Carvalho
3
, W. Van Elmpt
3
, P.
Lambin
3
, A. Dasu
4
, I. Toma-Dasu
5
1
Karolinska Institutet, Medical Radiation Physics-
Department of Oncology-Pathology, Stockholm, Swede
2
RaySearch Laboratories AB, RaySearch Laboratories AB,
Stockholm, Sweden
3
GROW-School for Oncology and Developmental Biology-
Maastricht University Medical Center, Department of
Radiation Oncology, Maastricht, The Netherlands
4
The Skandion Clinic, The Skandion Clinic, Uppsala,
Sweden
5
Stockholm University, Medical Radiation Physics-
Department of Physics, Stockholm, Sweden
Purpose or Objective
A previous study has shown that the early response to
treatment in NSCLC can be evaluated by stratifying the
patients in good and poor responders based on calculations
of the effective radiosensitivity, αeff, derived from two
FDG-PET scans taken before the treatment and during the
second week of radiotherapy [1]. However, the optimal
window during the treatment for assessing αeff was not
investigated. This study aims at assessing αeff of NSCLC
tumours on a new cohort of patients for which the second
scan was taken during the third week of treatment. The
optimal window for response assessment could be
determined by investigating the ability of the method to
predict treatment outcome through a comparison of the
results of a ROC analysis for the new cohort of patients,
imaged at three weeks, with the results of the previous
study in which patients were imaged at two weeks.
Material and Methods
Twenty-eight NSCLC patients were imaged with FDG-PET
before the treatment and during the third week of
radiotherapy. The patients received 45 Gy in 1.5 Gy
fractions twice-daily followed by a dose-escalation up to
maximum 69 Gy in daily fractions of 2 Gy. The outcome of
the treatment was reported as overall survival (OS) at two
years. αeff was determined at the voxel level taking into
account the voxel SUV in the two images and the dose
delivered until the second scan. Correlations were sought
between the average (a_αeff) or negative fraction
(nf_αeff) of αeff
values and the OS. The AUC and the p-
value resulting from the ROC analysis were compared to
the corresponding values reported for the case when the
second scan was taken during the second week of
treatment.
Results
The ROC curves in Figure 1 show the correlation between
a_αeff and OS and also the correlation between nf_αeff
and OS in the present and the earlier analysis. The results
expressed as AUC and p-value show the lack of correlation
between either a_αeff
(AUC=0.5, p=0.7) or nf_αeff
(AUC=0.5, p=0.8) and the OS for the scan at 3 weeks.
This
contrasts with the case when the second image was taken
during the second week of treatment (AUC=0.9,
p<0.0001). From the comparison of the ROC curves it
results that the values of αeff
can be used for predicting
the OS if the second scan is taken during the second week,
but not during the third week.
Conclusion