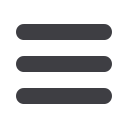

4
5.3. Huber’s method, which dynamically adjusts trimming to the empirical data distribution and
then follows a procedure similar to 5.2. This method is an M-estimator.
5.4. Use the median absolute deviation (‘MAD’) from the median and a normalizing factor of
1.4826, i.e., s = 1.4826 MAD.
5.5. Plot a Q-Q normal graph, select the range of data which is linear in the center, and compute s
as the slope of the line fit.
Note that all of methods 5.1)-5.5) will result in a lower bound for s, and are therefore maximally
liberal (in favor of the test method in question). These methods will generate comparable
estimates of s for typical datasets. These methods are heavily dependent upon the normal
distribution assumption, and are really only appropriate if it is known a priori that the data do, in
fact, follow a normal distribution, and any deviation from this must, in fact, be error.
It is the author’s opinion that methods 5.1) are due to an error in thinking
. What starts as a valid
‘robust’ theory for estimates of
location
is improperly twisted into a heavily biased estimate of
scale
. In the author’s opinion, method 3) is best compromise for the use of PT data to develop
estimates of reproducibility effects.
106