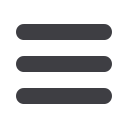

STATISTICAL ANALYSIS
Determining a One-Tailed Upper Limit for Future Sample
Relative Reproducibility Standard Deviations
F
OSTER
D. M
C
C
LURE
and J
UNG
K. L
EE
U.S. Food and Drug Administration, Center for Food Safety and Applied Nutrition, Office of Scientific Analysis and
Support, Division of Mathematics, Department of Health and Human Services, 5100 Paint Branch Pkwy, College Park, MD
20740-3835
A formula was developed to determine a one-tailed
100
p
% upper limit for future sample percent
relative reproducibility standard deviations
RSD
s
y
R
R
,%
100
, where
s
R
is the sample
reproducibility standard deviation, which is the
square root of a linear combination of the sample
repeatability variance
s
r
2
plus the sample
laboratory-to-laboratory variance
s
L
2
, i.e., s
R
=
s s
r
L
2
2
, and
y
is the sample mean. The future
RSD
R
,% is expected to arise from a population of
potential
RSD
R
,% values whose true mean is
R
R
,%
100
, where
R
and are the population
reproducibility standard deviation and mean,
respectively.
T
he sample relative reproducibility standard deviation
(
RSD
R
), usually expressed as a percent (
RSD
R
,%) is
obtained using a completely randomized model
(CRM; 1) and is defined as
RSD
s
y
R
R
,%
100
, where
s
R
is the
sample reproducibility standard deviation, which is the square
root of a linear combination of the sample repeatability
variance
s
r
2
plus the sample laboratory-to-laboratory
variance
s
L
2
, i.e.,
s
s s
R
r
L
2
2
, and
y
is the sample mean.
The sample
RSD
R
,% is an important method performance
indicator for validation organizations such as AOAC
INTERNATIONAL. Therefore, we reasoned that it might be
of great value to have a statistical procedure to determine a
one-tailed 100
p
% upper limit
P
for future sample
RSD
R
,%
values. A thorough literature search suggested that until now
no such procedure, based on a CRM, has existed. However,
we did note that Hald (2) had investigated the distribution of
the coefficient of variation for the single sample model, i.e.,
y
e
i
i
, where is an unknown constant and
e
i
is the
random error associated with
y
i
.
After considerable study of the problem, we came to the
conclusion that an exact limit for an
RSD
R
was unachievable,
primarily because the exact distributions of the sample
s
R
2
and
s
R
are very complicated, and possibly impossible to obtain.
Therefore, we sought to develop a formula to determine an
approximate one-tailed 100
p
% upper limit
p
for future
sample
RSD
R
values, obtained under a CRM model, by
extending Hald’s single sample approximation for
p
. In doing
so, we used a normal approximation and the delta-method
( -method; 1, 3, 4).
Collaborative Study Model
Here, we will review the CRM used by AOAC to establish
background notations. The model represents 2 sources of
variation: the first is often referred to as “among-laboratories”
and the other as “within-laboratory” variation. For the CRM,
an analytical result
y
ij
obtained by laboratory
i
on test
sample
j
is expressed as
y
ij
i
ij
,
i
= 1, 2, …,
L
and
j
= 1, 2, …,
n
, where is the grand mean of all potential
analyses for the material,
i
a constant associated with
laboratory
i
, and
ij
the random error associated with analysis
y
ij
. It is also assumed that
i
and
ij
are independent random
variables, such that
i
is normally distributed (~) with a mean
of 0 and variance of
L
2
, i.e.,
i
L
N
~ ,0
2
. Similarly,
ij
is
normally distributed with a mean of 0 and variance of
r
ij
r
N
2
2
0
,
i.e. ,
~ ,
.
Given the above model, we note that the expected value of
y
ij
equals the grand mean ( )
E y
ij
, the variance of
y
ij
equals the reproducibility variance var
y
ij
L
r
2
2
, the
covariance of
y
ij
and
y
ik
equals the “among-laboratories”
component of variation cov ,
y y
ij
ik
L
2
for
j k
, and the
correlation between
y
ij
and
y
ik
is
L
r
L
2
2
2
for
j k
, i.e., within a
given laboratory the
y
ij
are correlated under the CRM (5, 6).
M
C
C
LURE
& L
EE
: J
OURNAL OF
AOAC I
NTERNATIONAL
V
OL
. 89, N
O
. 3, 2006
797
Received May 17, 2005. Accepted by GL January 31, 2006.
Corresponding author's e-mail:
foster.mcclure@cfsan.fda.gov130