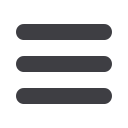

INFORMS Philadelphia – 2015
255
4 - Generalized Bounded Rationality and Robust Multi-Commodity
Network Design
Changhyun Kwon, Associate Professor, University of South
Florida, 4202 East Fowler Avenue, ENB 118, Tampa, FL, 33620,
United States of America,
chkwon@usf.edu,Longsheng Sun,
Mark Karwan
When the route-choice behavior of network users are uncertain, the notion of
bounded rationality has been used to allow users to choose sub-optimal routes
whose length is within a certain bound. In this paper, we provide another
framework to explain such bounded rationality assuming that network users
make perfectly rational route decisions, but with perception error in link costs. By
showing that some cases of the perception error model are equivalent to the
bounded rationality models, we establish the notion of generalized bounded
rationality. We demonstrate how the notion of generalized bounded rationality
can be used for robust multi-commodity network design problems and provide
computable optimization frameworks based on both links and paths. We illustrate
our approaches in the context of hazardous materials transportation.
MD72
72-Room 203A, CC
Panel Discussion on Big Data Science –
Opportunities and Challenges
Sponsor: Quality, Statistics and Reliability
Sponsored Session
Chair: Hui Yang, Associate Professor, Pennsylvania State University, 310
Leonhard Building, Industrial and Manufacturing Eng., State College,
PA, 16801, United States of America,
huy25@psu.edu1 - Panel Discussion on Big Data Science - Opportunities and
Challenges
Moderator:Hui Yang, Associate Professor, Pennsylvania State
University, 310 Leonhard Building, Industrial and Manufacturing
Eng., State College, PA, 16801, United States of America,
huy25@psu.edu,Panelists: Soundar Kumara, Liying Cui, Yan Xu,
Andrew Kusiak
This panel brings experts from academia and industry to discuss the opportunities
and challenges in big data science. The panelists are: Dr. Andrew Kusiak,
Professor and Chair, The University of Iowa; Dr. Soundar Kumara, Professor, The
Pennsylvania State University; Dr. Yan Xu, Senior Manager, Big data optimization
group, SAS Institue; Dr. Liying Cui, network improvement manager, Starbucks; ...
MD73
73-Room 203B, CC
Data Analytics in Manufacturing and
Service Industries
Sponsor: Quality, Statistics and Reliability
Sponsored Session
Chair: Nan Chen, National University of Singapore, 117576, Singapore,
isecn@nus.edu.sgCo-Chair: Kaibo Wang, Associate Professor, Tsinghua University,
Department of Industrial Engineering, Beijing, China,
kbwang@tsinghua.edu.cn1 - Modeling Air Quality Data based on Physical
Dispersion Processes
Xiao Liu, IBM, Singapore,
liuxiao@sg.ibm.comIn this paper, we investigate a statistical modeling approach based on a commonly
used physical dispersion model, called the scalar transport equation. The
relationship between the proposed spatial-temporal model and the physical model
is well established. The model describes the pollutant concentration by a non-
stationary random field with a space-time non-separable and anisotropic
covariance structure.
2 - Remaining Useful Life Prediction using Mixed Effects Model with
Mixture Prior Distributions
Raed Al Kontar, UW Madison, Eagle Heights 301J, Madison, WI,
United States of America,
alkontar@wisc.edu, Junbo Son,
Shiyu Zhou
In Modern engineering systems, pre-mature failure has become quite rare. Thus,
degradation signals used for prognosis are often imbalanced. Such imbalanced
data may hinder accurate remaining useful life prediction especially in terms of
detecting pre-mature failures as early as possible. We propose a degradation signal
based RUL prediction method to address the imbalance in data. This method
captures the characteristics of different groups and provides real time updating of
an in-service unit
3 - An OSA Detection Approach using a Discriminative Hidden
Markov Model
Xi Zhang, Assistant Professor, Peking University, 5 Yiheyuan Rd.,
Beijing, 100871, China,
xi.zhang@pku.edu.cn, Changyue Song,
Kaibo Liu
We proposed a novel detection approach for obstructive sleep apnea (OSA) based
on ECG signals by considering the temporal dependency. A discriminative hiddern
Markov model (HMM) and corresponding parameter estimation algorithms are
provided, and a real case study shows that a competitive performance including
accuracies of 94.3% for per-recording classification and 86.2% for per-segment
OSA detection with satisfactory sensitivity and specificity were achieved.
4 - Quantification and Monitoring on Ecommerce Reviews Dataset
Suoyuan Song, HKUST, Dept. of IELM, HKUST, Clear Water Bay,
Kowloon, Hong Kong - PRC,
songsuoyuan@gmail.com,
Fugee Tsung
Recently, the boom of e-merchants have attracted researchers on analyzing those
text-rich data. Unfortunately, these technologies have drawn little attention in
statistics and quality area. In this article, we aim to (1) use text mining
technologies to first quantify customer reviews, and (2) build statistical model to
monitor those text-rich reviews data.
MD74
74-Room 204A, CC
Advanced Maintenance Modeling
Sponsor: Quality, Statistics and Reliability
Sponsored Session
Chair: Yisha Xiang, Assistant Professor, Lamar University, 2626 Cherry
Engineering Building, Beaumont,, TX, 77710, United States of America,
yxiang@lamar.eduCo-Chair: David Coit, Professor, Rutgers University, Piscataway, NJ,
United States of America,
coit@rci.rutgers.edu1 - A Gaming Model for Outsourcing Maintenance under Uncertain
Fleet Expansion
Tongdan Jin, Texas State University, 601 University Drive,
San Marcos, TX, United States of America,
tj17@txstate.edu,
Shuying Li, Hong-zhong Huang
We propose a multi-criteria, performance-based maintenance contract to
maximize the utilities of the customer and the supplier under principal-agent
model. We prove that supplier’s decision on maintenance time, spares stocking
and repair capacity are fully observable to the customer, hence ensuring fully
efficient service delivery with no moral hazards. We further show that customers
are incentivized to share advance demand information with the supplier for new
product acquisition.
2 - A Survey of Condition-based Maintenance Policies for
Deteriorating Systems
Suzan Alaswad, Assistant Professor, Zayed University, Kalifa City
B, Abu Dhabi, United Arab Emirates,
Suzan.Alaswad@zu.ac.ae,Yisha Xiang
This paper reviews CBM literature highlighting the various stochastic modeling
approaches. This paper classifies the CBM models based on the system stochastic
degradation model (i.e. whether the degradation state is discrete or continuous)
into three deterioration models: discrete, proportional hazard model (PHM), and
continuous, and surveys existing CBM models based on this classification for both
single and multi unit systems.
3 - Markov Additive Processes for Degradation with Jumps under
Dynamic Environments
Yin Shu, University of Houston, E206 Engineering Bldg.2,
Houston, TX, 77204, United States of America,
yinshulx@gmail.com, Qianmei Feng, Edward Kao, Hao Liu,
David Coit
We use Markov additive processes to integrally handle the complexity of
degradation including internally- and externally-induced stochastic properties
with complex jump mechanisms. We derive the Fokker-Planck equations for such
processes, based on which we derive explicit results for life characteristics
represented by infinitesimal generator matrices and Levy measures. The
superiority of our models is their flexibility in modeling degradation data with
fluctuation under dynamic environments.
MD74