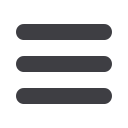

INFORMS Philadelphia – 2015
257
3 - Purchasing Postponement and SC Coordination in a
Decentralized N-V Model with Stochastic Demand
Sourabh Bhattacharya, Professor, Institute of Management
Technology, Hyderabad, India, 38, Cherlaguda Village
Shamshabad, Hyderabad, TS, 500048, India,
sbhattacharya@imthyderabad.edu.inWe determine the buyback price for a seller in a purchasing postponement
environment. Under stochastic demand a buyer postpones its purchasing decision
to reduce inventory cost. The seller on the other, offers a buck back rate to induce
higher orders from the buyer. Our model suggests that in a decentralized SC
under purchasing postponement, a buy back rate can be arrived at such that the
SC profits are maximized and SC coordination is established.
4 - Impact of Supply Relationship Dynamics on Firm Performance:
A Multilevel Empirical Analysis
Marcus Bellamy, Assistant Professor, Boston University Questrom
School of Business, 595 Commonwealth Avenue, Boston,
MA, 02215, United States of America,
bellamym@bu.edu,Soumen Ghosh, Manpreet Hora
We develop an empirical model to examine supply relationship dynamics as
drivers of firm performance. We use supply chain relationship and financial data
from the Bloomberg database. Our unique dataset allows us to investigate
manufacturing firms both as customers and suppliers. We use a multilevel mixed-
effects model combining firm and dyad level effects.
5 - Logistics Performance Improvement from Information Integration
Sung-tae Kim, Assistant Professor, SolBridge International School
of Business, 128 Uam-ro, Dong-gu, Daejeon, 300-814, Korea,
Republic of,
stkim1@solbridge.ac.kr,Gi-eyun Seo
This study examines the moderating effects of strategic and operational
information integration on the relationships between logistics performance and
organizational performance. This study measures logistics performance, in terms
of effectiveness, efficiency, and differentiation. Organizational performances are
classified as operational, financial, and market performances. The data from 321
manufacturing firms are evaluated using moderated hierarchical regression
analysis.
MD78
78-Room 301, CC
Optimization under Uncertainty with
Energy Applications
Contributed Session
Chair: Yu Zhang, University of Minnesota, 1033 29th Ave SE,
Apt B, Minneapolis, MN, 55414, United States of America,
zhan1220@umn.edu1 - Predicting and Mitigating Congestion for an Electric Power
System under Uncertainty
Dzung Phan, IBM T.J. Watson Research Center, 1101 Kitchawan
Road, P.O. Box 218, Yorktown Heights, NY, 10598, United States
of America,
phandu@us.ibm.com, Soumyadip Ghosh
Operation of a transmission grid has to handle increasing renewables uncertainty.
This necessitates probablistic modeling of the impact of uncertainty over the near-
future state of the grid. We propose a multi-period optimization model to estimate
the probability of the occurrence of a transmission line congestion event. The
model also helps to choose the best mitigation decisions to minimize the chances
of experiencing a congestion. A distributed algorithm is presented to efficiently
solve it.
2 - Optimal Operation and Services Scheduling for AA Electric
Vehicle Battery Swapping Station
Hrvoje Pandzic, Faculty of Electrical Engineering and Computing
University of Zagreb, Unska 3, Zagreb, Croatia,
Hrvoje.Pandzic@fer.hr, Mushfiqur Sarker, Miguel Ortega-vazquez
For a successful rollout of electric vehicles (EVs), it is required to establish an
adequate charging infrastructure. Battery swapping stations are poised as effective
means of eliminating the long waiting times associated with charging the EV
batteries. These stations are mediators between the power system and their
customers. This presentation describes an optimization framework for the
operating model of battery swapping stations.
3 - A Multi-period Energy-Aware Inventory Model with
CVAR Constraints
Niloofar Salahi, Graduate Research Assistant, Rutgers The State
University of New Jersey, 96 Frelinghuysen Road, Piscataway, NJ,
08854, United States of America,
niloofar.salahi@gmail.com,Mohsen Jafari
A risk-averse production planning with energy efficiency consideration is
introduced for an industrial process subject to stochastic demand. We present an
inventory model that minimizes expected costs while maintaining performance
requirements. The energy consumption is calculated using energy-performance
curves specific to the type of industrial process. We show that significant cost
saving is expected when adjusting the production plan according to time
dependent electricity pricing schemes.
4 - Robust Optimization vs. Stochastic Programming for Electricity
Generating Unit Commitment
Narges Kazemzadeh, Graduate Research Assistant, Iowa State
University, Industrial & Mfg. Sys. Engg., Ames, IA,
United States of America,
kazemzad@iastate.edu, Sarah Ryan
Unit commitment seeks the most cost effective generator commitment decisions to
meet net load while satisfying operational constraints. Stochastic programming
and robust optimization are the most widely studied approaches under
uncertainty in the load less variable generation. We investigate and compare the
performance of these approaches for a multi-bus power system in different aspects
including economic efficiency as well as the risk associated with the decisions.
5 - Real-time Energy Disaggregation using Online
Convex Optimization
Yu Zhang, University of Minnesota, 1033 29th Ave SE,
Apt B, Minneapolis, MN, 55414, United States of America,
zhan1220@umn.edu,Georgios Giannakis
By decomposing a whole electricity consumption into appliance-level signals,
energy disaggregation can induce end users’ saving behavior and significantly
improve energy efficiency. Capitalizing on underlying features of the sparse and
low-rank signals, an online convex optimization problem is formulated for the
real-time disaggregation task. An efficient online algorithm is developed with
provably sublinear regret. Numerical results corroborate the merits of the
proposed approach.
MD79
79-Room 302, CC
Software Demonstration
Cluster: Software Demonstrations
Invited Session
1 - LINDO Systems, Inc. - Optimization Modeling Made Easy
Mark Wiley, VP Marketing, LINDO Systems, Inc.
Come and learn how easy it is to: • Quickly build linear, nonlinear, quadratic,
conic and integer optimization models, • Incorporate uncertainty into
optimization models, • Easily access data from Excel and databases, • Seamlessly
embed a solver into your own application. Come and see a demonstration of the
power and flexibility of the new releases of: • LINDO API – a callable solver
engine, • LINGO – an integrated modeling language and solvers,• What’s Best! – a
large-scale solver for Excel.
2 - DO ANALYTICS - OPTEX Mathematical Modeling System:
The New Paradigm
Jesus Maria Velasquez, Chief Scientist, Do Analytics LLC
DO ANALYTICS presents OPTEX Mathematical Modeling System, a powerful
expert system that is changing the way to make large scale mathematical
programming models. OPTEX: * Generates programming codes in the most
powerful optimization technologies, including the SQL statements to connect any
DBMS. * Mixes the power of an optimization technology with the easiness of
EXCEL. * Works as a client & as an optimization server in the cloud. * Easy and
Fast, OPTEX represents the new generation to DO ANALYTICS
MD79