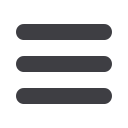

INFORMS Philadelphia – 2015
262
4 - Higher Rank-Order Semidefinite Cutting Planes for
Nonconvex QCQPs
Xiaofei Qi, PhD Student, Nanyang Technological University,
50 Nanyang Ave, Singapore, Singapore,
xqi001@e.ntu.edu.sg,Jitamitra Desai, Rupaj Nayak
We introduce a polynomial-time scheme to generate higher rank-order
semidefinite cutting planes that serve to tighten convex relaxations of nonconvex
quadratically constrained quadratic programs (QCQPs) and significantly improve
lower bounds. Suitably defined row-and-column based operations are used to
speed up the process of generating these cuts, and computational comparisons
across different types of relaxations shows the efficacy of these new cutting plane
strategies.
TA13
13-Franklin 3, Marriott
Optimizing Sharing Service/Economy Under
Uncertainty
Sponsor: Optimization/Optimization Under Uncertainty
Sponsored Session
Chair: Siqian Shen, Assistant Professor, University of Michigan,
1205 Beal Avenue, Ann Arbor, MI, 48105, United States of America,
siqian@umich.edu1 - Optimal Location Design of Carsharing Fleet under Uncertain
One-way and Round-trip Demands
Zhihao Chen,
czhihao@umich.edu, Siqian Shen
We allocate vehicles in a homogeneous carshare fleet to contracted locations, to
maximize the expected revenue from random demand for one-way and round
trip rentals. We use a spatial-temporal network and optimize both risk-neutral
and CVaR-based risk-averse two-stage stochastic programs with high demand
satisfaction rates. The two-stage problems are solved via branch-and-cut with
mixed integer rounding and we give insights on carsharing location design from
data reported by Zipcar in Boston.
2 - Online Resource Allocation with Limited Flexibility
Xuan Wang, New York University, 44 West 4th Street,
Suite 8-154, New York, NY, 10012, United States of America,
xwang3@stern.nyu.edu,Jiawei Zhang, Arash Asadpour
We consider a general class of online resource allocation problems with limited
flexibility, where a type j request can be fulfilled by resource j or resource j+1, and
we call this limited flexibility the long chain pattern. The long chain has been
studied in process flexibility and has been shown to be very effective in coping
with demand uncertainty under offline arrivals. We provide preliminary results
that show the effectiveness of the long chain when the arrivals are online.
3 - On-demand Staffing: Incentive Wage Contracts with
Guaranteed Fill Rates
Zhichao Zheng, Singapore Management University, Lee Kong
China School of Business, 50 Stamford Road, Singapore, 178899,
Singapore,
danielzheng@smu.edu.sg, Tao Lu, Yuanguang Zhong
We study the on-demand economy and its impact on labor market efficiency. We
consider n employers with uncertain and time-varying demands, and a platform
operator providing on-demand staffing services. We propose a novel fill rate-
based allocation policy enabling the on-demand workforce to be shared efficiently
among employers. We propose a form of incentive contracts based on fill rate
guarantees, and show that our contracts can induce the system-wise optimality in
decentralized systems.
TA14
14-Franklin 4, Marriott
Data-driven Optimization
Sponsor: Optimization/Optimization Under Uncertainty
Sponsored Session
Chair: Gah-Yi Vahn, Assistant Professor, London Business School,
Sussex Place, Regent’s Park, London, NW1 4SA, United Kingdom,
gvahn@london.edu1 - Data-driven Estimation of (s, S) Policy
Gah-Yi Vahn, Assistant Professor, London Business School,
Sussex Place, Regent’s Park, London, NW1 4SA, United Kingdom,
gvahn@london.eduI derive a tractable algorithm for computing the optimal (s,S) policy when the
decision maker has access to historical demand data. I show that this scheme
yields asymptotically optimal (s, S) policy and derive analytical characterisations
of confidence intervals, which is useful for operational decision-making.
2 - Near Optimal Ambiguity Sets in Distributionally
Robust Optimzation
Vishal Gupta, Assistant Professor, USC Marshall School of
Business, 3670 Trousdale Parkway, Bridge Hall 401 G,
Los Angeles, CA, 90089-0809, United States of America,
guptavis@usc.eduWe assess the strengths of data-driven ambiguity sets in distributionally robust
optimization (DRO) by bounding the relative size of a candidate set to a specific,
asymptotically optimal set. We find popular ambiguity sets are much larger than
this asymptotically optimal set, suggesting current DRO models are overly
conservative. We propose new “near-optimal” sets that are only a constant factor
larger than the optimal set and satisfy the usual robustness properties.
3 - A Time Based Choice Model
Tauhid Zaman, MIT Sloan School of Management, 50 Memorial
Drive, Cambridge, MA, 02139, United States of America,
zlisto@mit.eduWe present a choice model which incorporates the time it takes the user to make
a decision. Our model assumes that the further apart two items are in terms of
user preference, the faster a decision is made. We conduct a set of online polls and
find that this model captures actual human behavior. We also show that using
this time based choice model can learn user preferences with high accuracy than
standard choice models for a fixed sample size.
TA15
15-Franklin 5, Marriott
Patient Scheduling in Health Care
Sponsor: Optimization in Healthcare
Sponsored Session
Chair: Joseph Milner, Associate Professor Of Operations Management,
Rotman School of Management, University of Toronto,
105 St.George Street, Toronto, ON, M5S3E6, Canada,
Joseph.Milner@Rotman.Utoronto.Ca1 - Dynamic Patient Scheduling for Multi-Appointment
Health Care Programs
Adam Diamant, Assistant Professor Of Operations Management,
Schulich School of Business, York University,
111 Ian Macdonald Boulevard, Toronto, ON, M3J1P3, Canada,
adiamant@schulich.yorku.ca, Fayez Quereshy, Joseph Milner
We investigate the scheduling practices of a multidisciplinary, multistage,
outpatient health care program with no-shows. We formulate the problem as a
Markov Decision Process and use approximate dynamic programming to find
policies to schedule patients to appointments. We examine the quality of our
solutions via structural results and compare them to a simulation of the clinic.
Our results applied to the operation of a bariatric surgery program at a large
tertiary hospital in Toronto, Canada.
2 - Flexible Hospital-wide Patient Scheduling
Daniel Gartner, Carnegie Mellon University, 5000 Forbes Avenue,
Pittsburgh, PA, 15213, United States of America,
dgartner@andrew.cmu.edu,Rema Padman
We study a patient scheduling problem with admission decisions, clinical
pathways, day and overnight hospital resources, ward and surgical team
assignment flexibility, and overtime considerations. We model the problem using
Mixed-Integer Programming and embed it in a rolling horizon planning to take
into account uncertain recovery times of and remaining resource capacity for
patients. We analyze the impact of flexibility and uncertainty on several metrics.
3 - Coordinated Scheduling for a Multi-station Healthcare Network
Ester Dongyang Wang, PhD Candidate, University of Texas, IROM
Dept., Austin, TX, United States of America,
wdy@utexas.edu,
Douglas Morrice, Kumar Muthuraman
As the population ages, our healthcare industry must face the challenge of
increasing demand for care under constrained budget and resources. Our research
focuses on one of the central factors to the success of healthcare
reform–outpatient appointment scheduling. We develop a mechanism that
coordinates appointment scheduling among multiple services in a healthcare
network to improve access of care and reduce patient no-show rate. Our
approach has the potential to yield a global optimal solution.
4 - Appointment Scheduling and Walk-in Strategies with
Unpunctual Patients
Mohamad Soltani, University of Alberta, PhD Office, Business
Building,, University of Alberta, Edmonton, AB, T6G 2R3,
Canada,
soltani@ualberta.ca,Michele Samorani
It is commonly believed that clinics that schedule appointments have lower
patients’ waiting time and providers’ overtime than clinics that only allow walk-
ins. However, if we consider patient unpunctuality, walk-in-only clinics may
achieve a higher performance. In this research, we investigate the conditions
under which each strategy is preferable.
TA13