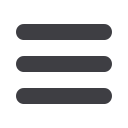

INFORMS Philadelphia – 2015
265
TA22
22-Franklin 12, Marriott
Two-Sided Matching Markets
Sponsor: Applied Probability
Sponsored Session
Chair: Peng Shi, MIT Operations Research Center, 1 Amherst Street,
E40-149, Cambridge, MA, 02139, United States of America,
pengshi@mit.eduCo-Chair: Yash Kanoria, Assistant Professor, Columbia University, New
York, United States of America,
ykanoria@columbia.eduCo-Chair: Itai Ashlagi, MIT, 100 Main St, Cambridge, MA, 02139,
United States of America,
iashlagi@mit.edu1 - On the Efficiency of Stable Matchings in Large Markets
Sangmok Lee, Univ of Pennsylvania, 3718 Locust Walk,
Philadelphia, PA, United States of America,
sangmok@sas.upenn.edu, Leeat Yariv
We study the wedge between stability and efficiency in large one-to-one
matching markets. We show stable matchings are efficient asymptotically for a
large class of preferences. In these environments, stability remains an appealing
objective even on efficiency grounds, and monetary transfers are not necessary
for efficiency purposes. Nonetheless, for severely imbalanced markets, when
preferences entail sufficient idiosyncrasies, stable outcomes may be inefficient
even asymptotically.
2 - Short Lists in Centralized Clearinghouses
Nick Arnosti, Stanford University, Stanford, CA,
United States of America,
narnosti@stanford.eduIn the presence of frictions, participants in centralized clearinghouses generally
fail to list all acceptable match partners. As a consequence, mutually acceptable
pairs are left unmatched. The number of unmatched agents (and the happiness of
matched agents) depends crucially on the structure of correlations in participants’
preferences. This work identifies a fundamental tradeoff between match quality
and quantity, and uses this to offer guidance for the design of school choice
mechanisms.
3 - How Much Choice is There in Two-sided Matching Markets?
Itai Ashlagi, MIT, 100 Main St, Cambridge, MA, 02139,
United States of America,
iashlagi@mit.eduWe study the structure of two-sided random matching markets with tiers. Our
results provide insights on the amount of choice agents have in the core.
TA23
23-Franklin 13, Marriott
Asymptotic Optimality in Processing Networks
Sponsor: Applied Probability
Sponsored Session
Chair: Itai Gurvich, Professor, Kellogg School of Management,
Northwestern, 2001 Sheridan Rd., Evanston, IL, 60201,
United States of America,
i-gurvich@kellogg.northwestern.edu1 - Approximations to Non-stationary Diffusion Processes
Harsha Honnappa, Perdue University, West Lafayette, IN, United
States of America,
honnappa@purdue.edu, Peter Glynn
Non-stationary diffusion processes emerge as limits to time inhomogeneous
queueing processes in appropriately defined ‘high intensity’ regimes. In general,
however, the transition densities of non-stationary diffusion processes are not
known in closed form. Thus, in this talk, we present analytical approximations to
expectations of these diffusion processes. This is joint work with Peter Glynn.
2 - On the Control of Fork-join Networks
Erhun Ozkan, University of Southern California, Marshall School
of Business, Los Angeles, CA, 90089, United States of America,
eozkan@usc.edu, Amy Ward
We study a prototypical fork-join network with two job classes and a shared
server that processes both job types. We show that a cmu-type static priority
policy is asymptotically optimal when the shared server is in some sense slow at
processing the more expensive jobs. Otherwise, a state-dependent slow departure
pacing control, under which the shared server sometimes gives priority to the less
expensive jobs, is asymptotically optimal.
3 - Insensitivity and Optimality of Load Balancing with Processor
Sharing Servers
Varun Gupta,
Varun.Gupta@chicagobooth.edu,Neil Walton
We present some recent results and ongoing work on near-optimality and
insensitivity properties of shortest queue load balancing under a carefully
constructed many-servers asymptotic regime, when all the servers use the
Processor Sharing scheduling rule.
4 - Capacity of Information Processing Systems
Kuang Xu, Stanford University, United States of America,
kuangxu@stanford.edu, Laurent Massoulie
We study an information processing system where jobs are to be inspected by a
set of experts. Inspections produce noisy results depending on the jobs’ hidden
labels and the expert types, and an inspection occupies an expert for one time
unit. The manager’s objective is to assign inspections so as to uncover the jobs’
hidden labels, using a minimum number of experts. Our main result is an
asymptotically optimal inspection policy as the probability of error tends to zero.
TA24
24-Room 401, Marriott
Intelligent Heuristics and Systems
Sponsor: Artificial Intelligence
Sponsored Session
Chair: Sam Thangiah, Professor/director, Slippery Rock University,
Artificial Intelligence and Robotics Lab, 250 ATS, Slippery Rock, PA,
16057, United States of America,
sam.thangiah@sru.edu1 - A New Mathematical Model for Pattern Recognition in the
Context of Feed Forward Neural Networks
Sam Findler, Slippery Rock University, 510 Campus Side Cir,
Slippery Rock, PA, 16057, United States of America,
srf5132@gmail.comThis paper sets out a new mathematical model for pattern recognition—in the
form of what I call Pattern Recognition Circuits (PRCs) and Pattern Recognition
Automata (PRAs). These new forms are given formal definitions and some
preliminary theorems are proven. Next, the model is applied to feed forward
neural networks, providing experimental grounding for the theory. Finally, the
place of this new mathematical model in the context of general computational
theory is discussed.
2 - Automatic Construction of Relational Features with Dataconda
Michele Samorani, Assistant Professor, University of Alberta,
3-20F Business Building, University of Alberta, Edmonton, AB,
T6G 2R6, Canada,
samorani@ualberta.caTraditional data mining and statistical techniques require a single table as input;
by contrast, I tackle the problem of findings patterns in a set of related tables
(Customers, Purchases, etc). This is made possible by Dataconda, a software freely
available to academics which automatically generates a large number of features
using information from all tables. In this talk, I will illustrate the benefits of this
approach through an example in retailing.
3 - Massively Parallel GPU Accelerated Genetic Algorithm for
Optimal Task Mapping in HPC Applications
Ramanan Sankaran, Computational Scientist, Oak Ridge National
Laboratory, P.O. Box 2008 MS 6008, Oak Ridge, TN, 37831,
United States of America,
sankaranr@ornl.govParallel applications on high performance computing (HPC) systems require
network and topology aware task placement to ensure performance and
scalability. We present a genetic algorithm for the quadratic assignment problem
(QAP) that utilizes thousands of GPU accelerated compute nodes allocated for the
application to compute an optimal task mapping in a few seconds. We show its
convergence characteristics and impact on real life physics simulations on Titan,
the most capable HPC system in the US.
4 - A Nao Humanoid Robot System for Interacting with
Autistic Children
Sam Thangiah, Professor/director, Slippery Rock University,
Artificial Intelligence and Robotics Lab, 250 ATS, Slippery Rock,
PA, 16057, United States of America,
sam.thangiah@sru.edu,
Daniel Martin, Michael Parnes, Mike Monfore, Stephen Fulton,
Zachary Kearney, Brian Atwell, Justin Cather, Andrew Rindt,
Sam Findler
The NAO humanoid robot is a two feet tall robot with two hands and two feet
with 25 degrees of freedom, cameras, microphones, sonar, infra-red and tactile
and pressure sensors. It has various communication devices and an Intel ATOM
processor. We describe a system implemented using the NAO robot to interact
with autistic children. The system is designed to interact with autistic children in
skill levels ranging from social to communication.
TA24