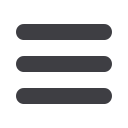

INFORMS Philadelphia – 2015
269
4 - On a Class of Reflected AR(1) Processes
Josh Reed, Associate Professor, NYU, 44 W. 4th St., New York, NY,
10012, United States of America,
jreed@stern.nyu.edu,Michel Mandjes, Onno Boxma
We study the the recursion Z(n+1) = max(aZ(n) + X(n),0) when X(n) is i.i.d. with
distribution the same as the difference of a positive random variable and an
independent, exponential random variable. We find the transform of Z(n) and,
when |a|<1, we perform a stationary analysis. In heavy-traffic, we show that the
process converges to a reflected Ornstein-Uhlenbeck process and the steady-state
distribution converges to the distribution of a normal random variable
conditioned to be positive.
TA33
33-Room 410, Marriott
Medical Decision Making in Chronic Disease
Screening and Treatment
Sponsor: Health Applications
Sponsored Session
Chair: John Silberholz, PhD Student, MIT, 77 Mass Ave, Bldg E40-130,
Cambridge, MA, 02139, United States of America,
josilber@mit.edu1 - An Analytics Approach to Designing Combination Chemotherapy
Regimens for Cancer
Dimitris Bertsimas, Professor, MIT, 77 Massachusetts Ave.,
Cambridge, MA, 02139, United States of America,
dbertsim@mit.edu,Allison O’hair, Stephen Relyea,
John Silberholz
We present a data-driven approach for designing new chemotherapy regimens for
advanced gastric and breast cancer. Our approach combines (i) construction of a
large-scale database of clinical trial results, (ii) statistical modeling to predict
outcomes of new drug combinations, and (iii) optimization models to select novel
treatments that strike a balance between maximizing patient outcomes
(exploitation) and learning new things about treatments that may be useful in the
future (exploration).
2 - On Estimating Optimization Model Parameters in Health
and Medicine
Thomas Trikalinos, Associate Professor, Brown University,
thomas_trikalinos@brown.eduCombining information from independent sources (meta-analysis) can increase
the likelihood of optimal actions in operational problems. Using as example the
optimization of breast cancer screening strategies, I will discuss methods for and
implications of synthesizing model parameter estimates from independent studies,
while accounting for biases (systematic errors) and nontransferability (differences
between the setting specified by the optimization and the settings of the data
sources).
3 - A Robust Approach to Designing Cancer Screening Strategies
John Silberholz, PhD Student, MIT, 77 Mass Ave, Bldg E40-130,
Cambridge, MA, 02139, United States of America,
josilber@mit.edu, Dimitris Bertsimas, Thomas Trikalinos
Many models have been proposed to evaluate screening strategies for detecting
cancer. Though each model for some cancer could be used to identify effective
screening strategies, models’ assumptions and structures can vary dramatically,
leading to differing conclusions about the most effective strategy. Using robust
and stochastic optimization, we identify screening strategies that are effective
across multiple models, which could increase confidence in the quality of the
identified strategies.
4 - Prioritizing Hepatitis C Treatment in United States Prisons
Can Zhang, Georgia Institute of Technology, 499 Northside Cir
NW, Apt. 315, Atlanta, GA, 30309, United States of America,
czhang2012@gatech.edu, Anthony Bonifonte, Turgay Ayer,
Jagpreet Chhatwal, Anne Spaulding
Correctional populations, which represent about 30% of the national Hepatitis C
virus (HCV) prevalence, offer a great opportunity to control the HCV epidemic.
New HCV treatments are very effective but also outrageously expensive.
Therefore, prisons are pressed to prioritize treatment decisions for HCV-infected
inmates. We propose a mathematical modeling framework for HCV treatment
prioritization decisions in prisons and present extensive numerical results based
on large datasets from US prisons.
TA34
34-Room 411, Marriott
Operations in Emergency Medicine
Sponsor: Health Applications
Sponsored Session
Chair: Yu Wang, PhD Student, Indiana University,
yw39@indiana.eduCo-Chair: Alex Mills, Assistant Professor, Indiana University, 1309 E.
10th Street, Bloomington, IN, 47405, United States of America,
millsaf@indiana.edu1 - Coordinated Response of Health Care Networks in Mass
Casualty Incidents
Mercedeh Tariverdi, PhD Student, University of Maryland,
mercedeh@umd.edu, Elise Miller-Hooks, Thomas Kirsch,
Scott Levin
A hybrid analytical-simulation and system-based approach is presented for
assessing the benefits of coordinated response of a health care network in a mass
casualty incident. The method accounts for incident-related operational
disruptions along with other sources of transient system behavior. Critical
resource management is included.
2 - An Empirical Study of Patient Discharge Decisions in
Emergency Departments
Eric Park, Postdoctoral Associate, The University of British
Columbia, 2053 Main Mall, Vancouver, BC, V6T1Z2, Canada,
eric.park@sauder.ubc.ca,Yichuan Ding, Mahesh Nagarajan
We analyze the physician’s patient discharge decision in EDs. We study how
inpatient wards play a role as additional resources to the ED in the discharge
process. We study over 530,000 patient discharges in five Canadian EDs.
3 - Allocation Models for Cooperation between Ambulance Services
Lavanya Marla, Assistant Professor, University of Illinois at
Urbana-Champaign, 104 S. Mathews Avenue, 216E, Urbana, IL,
61801, United States of America,
lavanyam@illinois.eduWe consider a setting where multiple ambulance service providers cooperate to
serve a population. Such settings have been observed in the case of large
casualties; and in emerging economies where 911-type services compete with
existing ad-hoc services. We first demonstrate the opportunity costs due to lack of
cooperation. Then we present a game-theoretic framework to model the
allocation of ambulances from competing service providers. We conclude with
results from a real-world case study.
4 - Surge: Smoothing Usage of Resources is Good for Emergencies
Yu Wang, PhD Student, Indiana University,
yw39@indiana.edu,Alex Mills, Jonathan Helm
Major hospitals often experience demand surges close to or above their capacity.
We study the interplay between reactive and proactive surge strategies and their
impacts on the hospital’s immediate response and recovery. We find that
immediate recourse actions at best sacrifice long-term recovery for short-term
capacity improvement, while proactive workload smoothing provides a Pareto-
improving response in both short- and long-term operational performance.
TA35
35-Room 412, Marriott
Panel Discussion: Infusing Learning from Hospitality
and Service Design to Healthcare: A Panel Discussion
Cluster: Hospitality, Tourism, and Healthcare
Invited Session
Chair: Rohit Verma, Professor, Cornell University, School of Hotel
Administration, 338 Statler Hall, Ithaca, NY, 14853-6902, United States
of America,
rohit.verma@cornell.edu1 - Infusing Learning from Hospitality and Service Design to
Healthcare: A Panel Discussion
Moderator: Rohit Verma, Professor, Cornell University, School of
Hotel Administration, 338 Statler Hall, Ithaca, NY, 14853-6902,
United States of America,
rohit.verma@cornell.edu, Panelists:
Craig Froehle, Nitin Joglekar
While fundamentally different from each other, the Healthcare and Hospitality
industries also share many common characteristics, challenges and constraints.
The purpose of this session is to discuss if and how lessons learnt from hospitality
can be infused to design better services within the context of healthcare, wellness
and senior living.
TA35