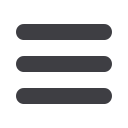

INFORMS Philadelphia – 2015
272
3 - Screening for Hepatocellular Carcinoma: A Restless Bandit Model
Elliot Lee, University of Michigan, 1205 Beal Ave, Ann Arbor, MI,
48109, United States of America,
elliotdl@umich.edu,
Mariel Lavieri, Michael Volk
Currently, all patients at risk for hepatocellular carcinoma (HCC) are screened
every six months. Recent medical discoveries have found a correlation between a
biomarker measured at each screening, and his/her risk of developing HCC. We
model the problem of simultaneously learning while allocating a limited number
of screening resources across a population as a restless bandit model. We prove
several structural properties of this problem, and ultimately derive a
corresponding optimal policy.
4 - Enhancing FDA’s Decision Making using Data Analytics
Vishal Ahuja, Southern Methodist University, P.O. Box 750333,
TX, United States of America,
vahuja@smu.edu, John Birge
Existing FDA surveillance methods are based on voluntary reporting or meta-
analysis primarily geared towards identifying new/unknown adverse events. We
propose a statistically robust and evidence-based empirical approach that focuses
on evaluating specific drug-related adverse outcomes to aid in the FDA decision-
making. We demonstrate our approach using a controversial black box warning.
Based on a large dataset from the Department of Veterans Affairs, we find that the
warning was not warranted.
TA43
43-Room 103A, CC
Measurement and Optimization in Online Advertising
Sponsor: Revenue Management and Pricing
Sponsored Session
Chair: Omar Besbes, Professor, Columbia University, Graduate School
of Business, New York, NY, 10027, United States of America,
ob2105@columbia.eduCo-Chair: Vineet Goyal, Associate Professor, Industrial Engineering and
Operations Research, Columbia University, 500 West 120th Street, 304
Mudd, New York, NY, 10027, United States of America,
vgoyal@ieor.columbia.eduCo-Chair: Garud Iyengar, Columbia University, S. W. Mudd 314,
500W 120th Street, New York, NY, United States of America,
garud@ieor.columbia.edu1 - Advertiser Revenue Versus Consumer Privacy in
Online Advertising
Vibhanshu Abhishek, Carnegie Mellon University, 5000 Forbes
Avenue, Pittsburgh, PA, 15213, United States of America,
vibs@andrew.cmu.edu, Arslan Aziz, Rahul Telang
Increasing concerns around consumer privacy have questioned the value of
targeted advertising. In this paper we quantify the value of privacy-intrusive
information in targeted advertising. Using individual level browsing/purchase
data we find that using more privacy-intrusive information increases the accuracy
of prediction of purchases, but at a decreasing rate. Targeted advertising is also
effective in increasing purchase probability. In our data, restricting cookies
reduces sales by 14%.
2 - Learning and Optimizing Reserve Prices in Repeated Auctions
Hamid Nazerzadeh, University of Southern California, Bridge
Memorial Hall, 3670 Trousdale Parkway, Los Angeles, 90089,
United States of America,
hamidnz@marshall.usc.edu,
Yash Kanoria, Renato Paes Leme, Afshin Rostamizadeh,
Umar Syed
A large fraction of online advertisements are sold via repeated second price
auctions. The reserve price is the main tool for the auctioneer to boost revenues.
However, the question of how to effectively set these reserves remains essentially
open from both theatrical and practical perspectives. The main challenge here is
that using previous bids to learn reserves could lead to shading of bids and loss of
revenue. I’ll present incentive compatible near-optimal leaning algorithms in this
context.
3 - Advertising on a Map
Sergei Vassilvitskii, Google, 111 8th Avenue, New York,
United States of America,
sergei@cs.stanford.eduWe study the mechanism design problem for advertising on a map. Unlike
traditional search advertising where there is a linear order on the slots, no such
structure exists in the case of a map. We begin with a model of the setting, noting
that the utility of an ad is discounted by the presence of competing businesses
nearby and its position in the set of ads ordered by distance from the user. We
present simple, approximately welfare maximizing allocation schemes with good
incentive properties.
4 - Attribution in Online Advertising under Markov Browsing Models
Antoine Desir, Columbia University IEOR department, 500 West
120th Street, Mudd 315, New York, NY, 10027, United States of
America,
ad2918@columbia.edu,Vineet Goyal, Garud Iyengar,
Omar Besbes
Web viewers are exposed to multiple ads across different websites before they
potentially make a purchase (leading to a conversion). In turn, a key question
facing the online advertising industry is that of attribution. We analyze attribution
based on Shapley values. While intractable in general, we provide
computationally tractable approximations to Shapley values under a general
Markov chain customer browsing behavior model and compare this attribution to
heuristics commonly used in practice.
TA44
44-Room 103B, CC
Pricing Issues in Revenue Management
Sponsor: Revenue Management and Pricing
Sponsored Session
Chair: Rene Caldentey, NYU, 44 W 4th St, New York, NY, 10012,
United States of America,
rcaldent@stern.nyu.eduCo-Chair: Ying Liu, Stern School of Business, New York University,
44 West 4th Street, KMC 8-154, New York, NY, 10012,
United States of America,
yliu2@stern.nyu.edu1 - Incorporating Online Customer Ratings in Pricing Decisions
Marie-claude Cote, Manager, Data Science, JDA Software -
Innovation Labs, 4200 Saint-Laurent #407, Montréal, QC, H2W
2R2, Canada,
Marie-Claude.Cote@jda.com,Philippe Tilly,
Nicolas Chapados
Research have demonstrated that online customer ratings have a huge impact on
the decision to choose a product. In hospitality, where the product is a hotel room
for a length of stay, customers consult an increasing number of reviews prior to
booking.Wewill describe an approach to automatically incorporate online user
rating impact in the pricing decisions of a hospitality revenue management
system.
2 - On the Equivalence of Quantity Pre-commitment and
Cournot Games
Amr Farahat, Washington University in St. Louis, One Brookings
Drive, St. Louis, MO, 63104, United States of America,
farahat@wustl.edu, Hongmin Li, Tim Huh
We establish sufficient conditions under which Cournot outcomes solve quantity-
followed-by-pricing games. Kreps and Scheinkman (1983) established this
connection for homogeneous product duopolies and Friedman (1988) for certain
differentiated product oligopolies under restrictive assumptions. Our research
provides conditions for more general differentiated product settings, including
multinomial logit models.
3 - Pricing Policies for Perishable Products with Demand Substitution
Ying Liu, Stern School of Business, New York University, 44 West
4th Street, KMC 8-154, New York, NY, 10012, United States of
America,
yliu2@stern.nyu.edu, Rene Caldentey
We study a monopolist’s optimal dynamic pricing policy for a family of substitute
perishable products. Customers arrive to the market according to an exogenous
stochastic process, each with a budget constraint. Upon arrival, each customer
first makes a decision among subfamilies that are differentiated by quality, and
then selects among horizontally differentiated products within the subfamily. We
characterize the optimal pricing policy and study the asymptotic approximation.
4 - Optimal Time and Price of Dynamic Upgrade
Xiao Zhang, PhD Candidate, University of Texas at Dallas,
Richardson, TX, United States of America,
xxz085020@utdallas.edu, Ozalp Ozer
Upgrade, a strategy used in travel industry to balance the supply-and-demand
mismatches among products of different quality levels, is usually offered either at
the booking time or the consumption time. We study a revenue management
problem of a firm which sells two products at fixed prices and offers upgrade
options anytime when necessary. The optimal policy specifies the time and price
of the upgrade option, and how many existing customers should be offered this
option.
TA43