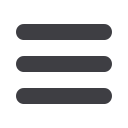

INFORMS Philadelphia – 2015
276
4 - When to Hire the First Employee? Behavioral Evidence
and Insights
Beatrice Boulu-reshef, Behavioral Research Associate, Darden
School of Business, 100 Darden Boulevard,
Charlottesville, VA, 22903, United States of America,
Boulu-ReshefB@darden.virginia.edu,Anton Ovchinnikov,
Charles Corbett
Effectively any entrepreneur shifts from doing all the work him/herself to hiring
someone to do part of that work. We use an analytical model and behavioral
experiments to study when entrepreneurs should and do hire their first
employee. Understanding both the optimal timing/conditions of hiring and the
deviations of the hiring patterns from optima have the potential to provide
insights to a very broad spectrum of entrepreneurs at the critical early stage of
their new venture formation process.
TA54
54-Room 108A, CC
Applying Machine Learning in Online Revenue
Management
Cluster: Tutorials
Invited Session
Chair: David Simchi-Levi, Professor, Massachusetts Institute of
Technology, 77 Massachusetts Avenue, Cambridge, MA, 02139,
United States of America,
dslevi@mit.edu1 - Tutorial: Applying Machine Learning in Online
Revenue Management
David Simchi-Levi, Professor, Massachusetts Institute of
Technology, 77 Massachusetts Avenue, Cambridge, MA, 02139,
United States of America,
dslevi@mit.eduIn a dynamic pricing problem where the demand function is unknown a priori,
price experimentation can be used for demand learning. In practice, however,
online sellers are faced with a few business constraints, including the inability to
conduct extensive experimentation, limited inventory and high demand
uncertainty. In this talk we discuss models and algorithms that combine machine
learning and price optimization that significantly improve revenue. We report
results from live implementations at companies such as Rue La La, Groupon and a
large European Airline carrier.
TA55
55-Room 108B, CC
Extensions of DEA
Cluster: Data Envelopment Analysis
Invited Session
Chair: Endre Bjorndal, Associate Professor, Norwegian School of
Economics, Helleveien 30, Bergen, 5045, Norway,
Endre.Bjorndal@nhh.no1 - Assessment of Alternative Approaches to Include Exogenous
Variables in DEA Estimates
Jose M. Cordero, Universidad de Extremadura, Av Elvas sn,
Badajoz, Spain,
jmcordero@unex.es,Daniel Santin
The aim of this paper is to compare the performance of some recent methods
developed in the literature to incorporate the effect of external variables into the
estimation of efficiency measures such as the conditional approach developed by
Daraio and Simar (2005, 2007) or the one-stage model proposed by Johnson and
Kuosmanen (2012). To do this, we conduct a Monte Carlo experiment using a
translog function to generate simulated data.
2 - Compensating for Exogenous Cost Drivers in the Regulation of
Electricity Networks
Endre Bjorndal, Associate Professor, Norwegian School of
Economics, Helleveien 30, Bergen, 5045, Norway,
Endre.Bjorndal@nhh.no,Maria Nieswand, Mette Bjørndal,
Astrid Cullmann
The present yardstick model used by the Norwegian regulator compensates, via
two-stage DEA efficiency analysis, for a number of environmental factors. These
factors are correlated with measured efficiency and company size. We compare
conditional nonpararametric methods to current benchmarking model, and we
discuss whether the choice of method affects the revenue caps of companies in a
systematic manner.
3 - Slacks-based Measure Variations Revisited
Kaoru Tone, Professor, National Graduate Inst. for Policy Studies,
7-22-1 Roppongi, Minato-ku, Tokyo, 106-8677, Japan,
tone@grips.ac.jpIn Tone (2010), I developed four variants of the SBM model where main concerns
are to search the nearest point on the efficient frontiers of the production
possibility set. However, in the worst case, a massive enumeration of facets of
polyhedron associated with the production possibility set is required. In this
paper, I will present a new scheme for this purpose which requires a limited
number of additional linear program solutions for each inefficient DMU.
TA56
56-Room 109A, CC
Execution Mode Choices for NPD
Cluster: New Product Development
Invited Session
Chair: Pascale Crama, Singapore Management University, 50 Stamford
Road, Singapore, 178899, Singapore,
pcrama@smu.edu.sg1 - Managing Exploration and Execution
Nittala Lakshminarayana, University of California San Diego,
9256 Regents Road Apt. G, La Jolla, CA, 92037, United States of
America,
Lakshminarayana.Nittala@rady.ucsd.edu,Sanjiv Erat,
Vish Krishnan
We model Innovation as a multi-stage activity consisting of Exploration and
Execution. Within this parsimonious model that mimics many contexts in
Innovation, we consider the effect of incentives and several institutional features
on the optimal idea generation and development strategy.
2 - Customer Co-design: The Role of Product Lines
Sreekumar Bhaskaran,
sbhaskar@mail.cox.smu.edu,Amit Basu
Involving customers in the new product design can be a powerful means to
achieve high levels of customer satisfaction and market success. However, the
“co-design” process may require participating customers to commit significant
time and effort, while facing the uncertainty that the firm may overprice the
custom product. Since this reduces a customers incentive to commit effort up-
front, co-design can be difficult to motivate. We develop analytical models that
capture these various effects.
3 - Flexibility and Knowledge Development in Product Development:
Insights from a Landscape Search Model
Mohsen Jafari Songhori, Jsps Research Fellow, Tokyo Institute of
Technology, J2 Bldg., Room 1704, 4259 Nagatsuta-cho,, Tokyo,
226-8502, Japan,
mj2417@gmail.com,Majid Abdi, Takao Terano
This study introduces a landscape model of Product Development (PD). The
model captures different PD performance aspects (e.g. development time, quality
and cost) and their trade-offs. Moreover, knowledge development dynamics and
flexibility are incorporated in the model to investigate how strategies toward
these, in PD process, are associated with the performance measures.
TA57
57-Room 109B, CC
Applications of Stochastic and Dynamic
Programming in Energy
Sponsor: ENRE – Energy II – Other (e.g., Policy, Natural Gas,
Climate Change)
Sponsored Session
Chair: Andrew Liu, Assistant Professor, Purdue University,
315 N. Grant Street, West Lafayette, IN, 47907,
United States of America,
andrewliu@purdue.edu1 - Approximate Dynamic Programming for Pricing-based Real-time
Demand Management
Ozgur Dalkilic, The Ohio State University, 205 Dreese Labs, 2015
Neil Ave, Columbus, OH, 43210, United States of America,
dalkilic.1@buckeyemail.osu.edu, Atilla Eryilmaz, Antonio Conejo
We consider the real-time demand management problem of a load aggregator that
coordinates the consumer demand to match a predetermined daily load. The
aggregator’s objective is to minimize its payment to the real-time market. Under
uncertainty of the market prices, we derive dynamic pricing algorithms that
approximate the optimal dynamic programming solution. We show via numerical
investigations that the proposed algorithms coordinate flexible demand and
achieve close to optimal allocation.
TA54