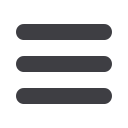

INFORMS Philadelphia – 2015
363
2 - Long-Term Partnership for Achieving Efficient Capacity Allocation
Fang Liu, Assistant Professor, Nanyang Business School, Nanyang
Technological University, 50 Nanyang Avenue, South Spine
S3-B2A-13, Singapore, 639798, Singapore,
liu_fang@ntu.edu.sg,Tracy Lewis, Nataliya Kuribko, Jeannette Song
We consider a manufacturer and a group of buyers who share a scarce but
expensive-to-build capacity over a finite period. Each member has private history-
dependent demand information and makes unverifiable investments. Because of
the high uncertainty, achieving supply chain efficiency while sustaining under a
dynamic environment is challenging for the partnership. We construct a
membership agreement that enforces efficient capacity allocation and investments
by introducing a novel breach remedy.
3 - The Perils of Sharing Information in a Trade-association
Noam Shamir, Assistant Professor, Tel-Aviv University, Haim
Levanon, Tel-Aviv, Israel,
nshamir@post.tau.ac.il, Hyoduk Shin
Studying the incentives of a group of retailers, organized as a trade association, to
exchange forecast information, we compare between two industry policies:
exclusionary and non-exclusionary information sharing. Although non-
exclusionary policy has been advocated to promote information sharing, we show
the opposite can happen and explain the reason.
4 - Aligning Incentives in Omni-channel Sale
Elnaz Jalilipour Alishah, PhD Candidate, University of
Washington, Seattle, Foster School of Business, Mackenzie Hall
358, Seattle, WA, 98195-3200, United States of America,
jalilipo@uw.edu, Yong-Pin Zhou, Jingqi Wang
We consider a retailer with both online and offline channels. While the online
store exerts costly effort to attract customers, the offline store handles inventory
for both locations – including fulfillment of online orders. We study how the
retailer should appropriately credit both channels to align their incentives.
TD51
51-Room 106B, CC
Innovative and Entrepreneurial OM
Sponsor: Manufacturing & Service Operations Management
Sponsored Session
Chair: Onesun Steve Yoo, University College London, Gower Street,
London, WC1E 6BT, United Kingdom,
o.yoo@ucl.ac.uk1 - Pricing and Capacity Planning for Flexible Consumption
Sanjiv Erat, UCSD, Gilman Drive, La Jolla, CA,
United States of America,
serat@ucsd.edu,Sreekumar Bhaskaran,
Rajiv Mukherjee
Motivated by the emergence of flexible consumption opportunities - such as the
rollover cellphone plans offered by many mobile providers - we study a firm’s
pricing and capacity planning decision when the timing of consumption is a
choice variable for consumers. Subsequently, we explore the effect of
heterogeneity in consumer preferences and its effect on a firm’s decision of how
much flexibility to offer.
2 - Improving Supply Chain Compliance using Buyer Consortiums
Prashant Chintapalli, Anderson School of Management,
University of California, Los Angeles, CA, 90095, United States of
America,
prashant.chintapalli.1@anderson.ucla.edu,
Kumar Rajaram, Felipe Caro, Chris Tang
Motivated by the Accord on Fire and Building Safety in Bangladesh we study the
effectiveness of buyer consortiums. We show that a consortium can increase
factory compliance and improve the buyers’ profits, though possibly at the
expense of the supplier. We also study the conditions under which a buyer should
join the consortium and characterize the settings in which the whole supply chain
is better off.
3 - Startup as a Process: Increase Your Chances of Success via a
Just-in-time Approach
Christophe Pennetier, PhD Student, INSEAD,
1 Ayer Rajah Avenue, Singapore, 138676, Singapore,
Christophe.Pennetier@insead.edu, Karan Girotra,
Serguei Netessine
Using a unique and novel dataset, we study the success of startups modeled as a
process: what is the best configuration for batches of funding cash –in terms of
size and frequency– to exit successfully? Our results suggest that founders should
not be obsessed by the amount of money they raise in any single round. It is
better to raise small batches more often than the other way around.
4 - Selling Fashionable Products: Change Price or
Facilitate Learning?
Yufei Huang, PhD Student, University College London, Gower
Street, London, United Kingdom,
yufei.huang.10@ucl.ac.uk,Onesun Steve Yoo, Bilal Gokpinar, Chris Tang
Firms selling new fashionable products are shifting their focus away from pricing
and towards facilitating the learning process for customers. To understand this
phenomenon, we present a stylized model with pricing and three channels
through which customers learn. We find that for new fashionable products,
facilitating learning can lead to greater profit than variable pricing. Moreover
when firms facilitate learning, variable pricing has only a marginal effect on firm
profits.
TD52
52-Room 107A, CC
Social Media and Internet Marketing
Sponsor: Marketing Science
Sponsored Session
Chair: Michael Trusov, University of Maryland,
3454 Van Munching Hall, College Park, MD, United States of America,
mtrusov@rhsmith.umd.edu1 - Attribution Metrics and Return on Keyword Investment in Paid
Search Advertising
Hongshuang Li, Indiana University, Bloomington, IN,
United States of America,
lhshruc@gmail.com,Siva Viswanathan,
Abhishek Pani, P.k. Kannan
In this paper, we analyze the impact of the attribution metric used for imputing
conversion credit to search keywords on the overall effectiveness of keyword
investments in search campaigns. We model the relationship among the
advertiser’s bidding decision for keywords, the search engine’s ranking decision
for these keywords, and the consumer’s click-through rate and conversion rate on
each keyword, and analyze the impact of the attribution metric used on the
overall return-on-investment of paid search advertising.
2 - Controlling for Self Selection Bias in Customer Reviews
Leif Brandes, University of Warwick, Coventry, CV4 7AL,
United Kingdom,
Leif.Brandes@wbs.ac.uk, David Godes,
Dina Mayzlin
Customers frequently use user online reviews as a valuable information resource
before making a purchase. This observation has motivated a large number of
empirical studies, and it is now a well-established finding that customer online
reviews impact product sales. However, one possible criticism of online user
reviews as a source of information is the self-selection inherent in the review
process. That is, consumers self-select into choosing whether to review a product,
which suggests that reviews may be prone to the extremity bias: the distribution
of reviews may be more polarized than the true preference distribution . This of
course implies that posted review valence may not always provide an unbiased
representation of customers’ true product experiences. We provide survey
evidence that demonstrates that customers who post an online review tend to
have more extreme opinions than customers who never post a review. We
hypothesize that the consumers who have more extreme opinions post their
reviews quicker, while the consumers with more moderate opinions may take
longer to post a review, which implies that in the limit some consumers with
moderate opinions may never post a review. One implication of this is that
reviews that arrive after a long time lapse are more similar to the opinions of the
non-responders. Hence, a firm that is able to observe the time lapse between the
experience and the review should be able to calculate the valence of reviews in a
way that corrects for the non-response bias. That is, we suggest how to correct for
the extremity bias by taking into account the latency of response data. To test our
hypotheses, we use a new dataset from a large online travel portal. Overall, we
have detailed information on 1.26 million bookings and 2.75 million reviews over
the complete history of the firm (twelve years). Because we observe hotel
bookings and review provision behavior at the individual customer level, we
know for each customer the exact duration between her last travel day and the
day that she provided the review. Based on our empirical results, we show how
customer self-selection across time impacts her review behavior and suggest a
method for controlling for this bias.
TD52