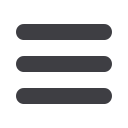

INFORMS Philadelphia – 2015
368
2 - Flexible Capacity Management with Advanced Information
Julian Kurz, Chair of Logistics and Quantitative Methods in
Business Administration, University of Wuerzburg,
Stephanstrasse 1, Wuerzburg, 97070, Germany,
julian.kurz1@uni-wuerzburg.deWe consider a maintenance service provider that overhauls technical equipment
for customers in a central facility. A flexible capacity control policy is developed
such that capacity costs and queue length-related holding costs are minimized.
We investigate three operating modes, each taking into account a different
amount of information (reactive/single-/multi-stage proactive modes). In the
proactive modes, advanced information regarding future jobs is utilized.
3 - How to Take Advantage of Crowdsourcing to Collect New Ideas
about Product Innovation?
Wanjiang Deng, Huazhong University of Science and Technology,
School of Management, Luoyu Road 1037, Hongshan District,
Wuhan, 430074, China,
dengwj01@foxmail.com, Shihua Ma
Crowdsourcing is gaining more and more attention in both practice and research
field. We stand on the position of the company who is going to propose a task
about product innovation on one online crowdsourcing platform, and investigate
its best strategies of both choice of platform and reward setting. We derive
solutions of the base model and then extend it to some detail aspects. Finally, we
discuss the managerial insights of our research.
4 - A Heuristic for Hospital Operating Theatre Scheduling
under Uncertainty
Milad Zafar Nezhad, Wayne State University, Industrial and
Systems Engineering Dep, Detroit, MI, 48202, United States of
America,
fq3963@wayne.edu,Hossein Badri, Kai Yang
Resource planning is one of the most important issues in healthcare operating
management. In this research a heuristic solution algorithm based on the shifting
bottleneck method is developed for hospital operating theatre scheduling when
some parameters are not deterministic. The developed algorithm is applied on
several instances to evaluate its applicability and performance.
5 - Contractual Coordination of Agricultural Cooperatives with
Quality Specifications
Xiaoyan Qian, PhD, The University of Auckland, 486 Parnell
Road, Auckland, New Zealand,
x.qian@auckland.ac.nzThis talk examines how agricultural cooperatives can motivate farmers’ effort
when the market price depends on the quantity of high quality produce. We
assume that a quality premium is offered to farmers and that their pay-outs are
made progressively. We propose a two-stage stochastic model. The main findings
are conditions for when the supply chain can be coordinated, that effort is
motivated by the quality requirement, and that the progressive payment is
needed for coordination.
TD64
64-Room 113A, CC
Optimization and Utility Theory
Sponsor: Decision Analysis
Sponsored Session
Chair: Hiba Baroud, Vanderbilt University, 400 24th Avenue South,
Nashville, TN, 37205, United States of America,
hiba.baroud@vanderbilt.edu1 - A Multi-criteria Decision Analysis Approach for Importance
Ranking of Network Components
Yasser Almoghathawi, PhD Candidate, University of Oklahoma,
202 W Boyd St., Norman, OK, 73019, United States of America,
moghathawi@ou.edu, Kash Barker
Analyzing network vulnerability is a key element of network planning and
preparing for a disruptive event that might impact the performance of the
network. Many importance measures have been proposed to identify and rank
the important components in a network to focus on preparedness efforts. We
integrate a number of flow-based importance measures with a multi-criteria
decision analysis technique, TOPSIS, highlighting how different weighting
schemes can lead to different rankings.
2 - Modeling Uncertainty in Risk-preference Elicitation
Dharmashankar Subramanian, Research Staff Member, IBM
Research, 1101 Kitchawan Rd, Rte 134, Yorktown Heights, NY,
10598, United States of America,
dharmash@us.ibm.com,
Debarun Bhattacharjya, Mengyang Gu
Utility functions are used to model a decision-maker’s risk-preferences. However,
interactive elicitation of a precise utility function is fraught with many practical
challenges such as noise, inconsistency and bias in the responses to questions. In
this work, we provide a flexible model along with Bayesian analysis to calibrate
random utility functions and to quantify different sources of uncertainty. We
present both theoretical and numerical results.
3 - Modeling Reference Dependence using
One-switch Independence
David Vairo, Virginia Commonwealth University, 2415 Krossridge
Road, N. Chesterfield, VA, 23236, United States of America,
vairodl@vcu.edu,Jason Merrick
We present an application of multi-attribute one-switch independence to single
attribute gambles by modeling chance as an attribute, which models reference
dependence and shows it is equivalent to one-switch independence. The resulting
form obeys stochastic dominance while incorporating probabilistic sensitivity,
utility curvature, reference dependence, and loss aversion. The approach connects
single-attribute behavioral and multi-attribute prescriptive decision analysis.
4 - Multiobjective Network Resilience Model with Parallel
Component Recovery
Nazanin Morshedlou, PhD Student, University of Oklahoma, 202
W. Boyd St., Room 424, Norman, OK, 73071, United States of
America,
nazanin.morshedlou@ou.edu, Kash Barker
This work introduces a multiobjective formulation that trades off investments to
enhance network resilience in the form of (i) strengthening link capacity
following a disruptive event to decrease vulnerability, and (ii) introducing
“parallel component at a time” recovery scheduling to improve recoverability.
Given the uncertainty associated with critical infrastructures, robust interval
optimization is used to solve the multiobjective formulation
TD65
65-Room 113B, CC
Near Miss and Threshold Events and Their Influence
on Risk Perception and Behavior
Sponsor: Decision Analysis
Sponsored Session
Chair: Florian Federspiel, IE Business School, Maria de Molina 12,
Bajo, Madrid, 28006, Spain,
ffederspiel.phd2014@student.ie.edu1 - Conceptualizing Perceptions of Near Misses
Richard John, Associate Professor, University of Southern
California, 3620 McClintock Ave., Dept. of Psychology, MC-1061,
Los Angeles, CA, 90266-1061, United States of America,
richardj@usc.edu, Jinshu Cui, Heather Rosoff
I will present a dynamic probabilistic model for describing and defining near miss
events. The model is useful for highlighting characteristics of a near miss that
determine the extent to which the event is perceived as a near miss.
Measurement of individual differences in perceptions of near misses will also be
discussed.
2 - Small Near-Misses: Too Weak of a Warning Signal
Robin Dillon-Merrill, McDonough School of Business,
Georgetown University, Washington, DC, 20057,
United States of America,
rld9@georgetown.eduThis research demonstrates that individuals too often evaluate near-misses as
successful events even when it was fortunate chance that prevented the same
event from resulting in a failed outcome. These weak signals of problems are then
overlooked as the warnings they could potentially be. This problem is exacerbated
as small near-misses accumulate over time, and decision makers increasing accept
more risk.
3 - Learning from Threshold Events
Wenjie Tang, IE Business School, Calle de Maria de Molina 12,
Piso 5, Madrid, MA, 28006, Spain,
Wenjie.Tang@ie.edu,
Steffen Keck, Matthias Seifert
Threshold events are events that are triggered when an observable underlying
random variable passes a known threshold. We suggest that when individuals
learn from past threshold events, their judgments depend strongly on whether
the realization of the underlying variable triggers the threshold event; the extent
to which the realization has been close to the threshold will be discounted.
Results from a laboratory experiment support our main hypothesis.
4 - The Experience of Near Miss Events under Ambiguity
Florian Federspiel, IE Business School, Maria de Molina 12,
Bajo, Madrid, 28006, Spain,
ffederspiel.phd2014@student.ie.edu,
Matthias Seifert
Near miss events are often clouded in ambiguity, allowing for hubris and
misattribution of what caused success or prevented failure. We investigate the
experience of near miss events, probabilistic events nearly resulting in loss
outcomes that do not materialize due to chance circumstances, and related
changes in risk perception and behavior under both ambiguity and unawareness.
We find that the near miss effect (an increase in risk taking behavior) principally
occurs under ambiguity.
TD64