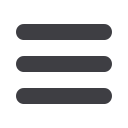

INFORMS Philadelphia – 2015
371
TD72
72-Room 203A, CC
Design and Analysis of Data with Complex Structure
Sponsor: Quality, Statistics and Reliability
Sponsored Session
Chair: Xinwei Deng, Assistant Professor, Department of Statistics,
Virginia Tech, 211 Hutcheson Hall, Blacksburg, VA, United States of
America,
xdeng@vt.eduCo-Chair: Ran Jin, Virginia Tech., Grado Department of Industrial and,
Systems Engineering, Blacksburg, VA, 24061, United States of America,
jran5@vt.edu1 - Markov Switching Autoregressive Models with Applications in
Cell Biology
Ying Hung, Rutgers University, Piscataway, NJ,
United States of America,
yhung@stat.rutgers.eduWe will introduce a new framework based on Markov switching autoregressive
models for the analysis of experiments in cell biology.
2 - Sparse Particle Filtering
Yun Chen, University of South Florida, 4202 E. Fowler Ave.
ENB118, Tampa, FL, United States of America,
yunchen@mail.usf.edu,Hui Yang
Wireless sensor network has emerged as a key technology for monitoring space-
time dynamics of complex systems. Distributed sensing gives rise to
spatially-temporally big data. Realizing the full potentials of distributed sensing
calls upon the development of space-time modeling of measured signals in
dynamically-evolving physical environment. This paper will present a new
approach of sparse particle filtering to model spatiotemporal dynamics of big data
in distributed sensor network.
3 - Graphical Modeling with Functional Variables
Ran Jin, Virginia Tech., Grado Department of Industrial and,
Systems Engineering, Blacksburg, VA, 24061, United States of
America,
jran5@vt.edu, Hongyue Sun, Shuai Huang
Graphical models are widely used to model variable relationship. Traditional
graphical models are mainly used to model scalar variables. In this paper, a
graphical model with functional variables is proposed. Functional regression
models, combined with sparsity-inducing norms, are applied for the graphical
modeling. A case study and simulation will be used to evaluate the proposed
method.
4 - Optimal Design of Experiments for Generalized Linear Models
Abhyuday Mandal, University of Georgia, Department of
Statistics, 101 Cedar Street, Athens, GA, 30602-7952, United
States of America,
amandal@stat.uga.edu, Liping Tong, Jie Yang,
Dibyen Majumdar
Generalized linear models have been used widely for modeling the mean
response both for discrete and continuous random variables with an emphasis on
categorical response. Here we find efficient designs in the context of several
optimality criteria, namely D-optimality, EW-optimality and Bayesian optimality.
Regular fractional factorials with uniform replications are often used in practice.
We show that these popular designs are often not optimal for binomial, Poission
and multinomial cases.
TD73
73-Room 203B, CC
Recent Advances in Analyzing Experiments
Sponsor: Quality, Statistics and Reliability
Sponsored Session
Chair: Rong Pan, Associate Professor, Arizona State University, P.O. Box
878809, Tempe, AZ, United States of America,
rong.pan@asu.edu1 - Performance of Standard Mixture Designs in Modeling
Ordinal Responses
Mickey Mancenido, Arizona State University, 699 S. Mill Ave.,
Tempe, AZ, United States of America,
mmanceni@asu.edu,
Douglas Montgomery, Rong Pan
Mixture designs for the proportional odds model — the widely used model for
ordinal data — are lacking in literature. A viable surrogate are the standard
mixture designs for linear models with normal errors. We are interested in the
performance of the simplex-lattice with axial runs, simplex-centroid, computer-
generated I-optimal, and the uniform space-filling designs when used in a
mixture study with an ordinal response.
2 - Generating and Comparing Pareto Fronts of Experiment Designs
to Account for Multiple Objectives
Byran Smucker, Assistant Professor, Miami University,
100 Bishop Circle, 311 Upham Hall, Oxford, OH, 45056,
United States of America,
smuckerb@miamiOH.edu,
Yongtao Cao, Tim Robinson
In many design scenarios the experimenter entertains multiple, conflicting
objectives. The Pareto approach to experiment design is to construct a set of
designs while explicitly considering trade-offs between criteria. The true Pareto
front is not known, which creates problems in assessing front quality, and existing
algorithms are inefficient, ineffective, or both. Here, we introduce an improved
measure of front assessment, and present a new algorithm to generate Pareto
fronts of designs.
3 - Cost Constrained ALT with Exponentially Changing
Stress Durations
David Han, University of Texas, One UTSA Circle, San Antonio,
TX, United States of America,
David.Han@utsa.eduWhen designing ALT, several variables such as the allocation proportions and
stress durations must be determined carefully because of constrained resources.
This talk discusses the optimal decision variables based on the popular optimality
criteria under the constraint that the total cost does not exceed a pre-specified
budget. A general scale family of distributions is considered to accommodate
different lifetime models for flexible modeling with exponentially decreasing
stress durations.
4 - Integration of Computer and Physical Experiments for Improving
Predictive Inference
Arda Vanli, Associate Professor, Florida State University,
Tallahassee, FL,
avanli@fsu.edu, Spandan Mishra
A Bayesian predictive approach is developed to combine data from designed
experiments on physical process and computer predictions. Predictive distribution
of a regression model is used for inference on the outcome variable and issues
including predictive capability, model adequacy and sensitivity to prior
specifications are discussed. Applications from structural loss prediction and
quality control are presented for illustrations.
TD74
74-Room 204A, CC
Bayesian Applications in Industrial Statistics
Sponsor: Quality, Statistics and Reliability
Sponsored Session
Chair: Refik Soyer, The George Washington University, 2201 G St NW,
Washington, DC, United States of America,
soyer@gwu.edu1 - What do Coin Tosses, Vessel Traffic Risk Assessment and Return
Time Uncertainty Have in Common?
Johan Rene Van Dorp, Professor, The George Washington
University, 800 22nd Street NW, Suite 2800, Washington, DC,
United States of America,
dorpjr@gwu.edu,Jason Merrick
Via a coin toss argument we will advocate decision making under uncertainty in
vessel traffic risk assessment to be informed by relative risk comparisons by
highlighting the analogy of an accident potentially occurring in a traffic situation
with the toss of a biased coin. That same analogy is next used to demonstrate the
large uncertainty bands that result for average return times of accidents in this
context.
2 - Integrating Expert Judgement and Bayesian Analysis
Thomas A. Mazzuchi, Professor And Chairman,
George Washington University, Washington DC, DC, 20052,
United States of America,
mazzu@gwu.eduThere is a growing need for marrying the fields of expert judgement and Bayesian
Analysis that is, using the Expert Judgement approach to define prior
distributions and for understanding the effects of the elicitation, codification and
combination on the prior distribution and subsequent posterior analysis. This
paper presents an investigation of the above for a simple model using the Classical
Model for Expert Judgment by Cooke (2001).
3 - An Augmented Simulation Approach for Bayesian Design of
Life Tests
Refik Soyer, The George Washington University, 2201 G St. NW,
Washington, DC, United States of America,
soyer@gwu.eduIn this talk we consider a Bayesian decision theoretic setup for optimal design of
life tests. More specifically, we consider use of augmented probability simulation
with a conjugate class of utility functions for design of life tests. We illustrate the
implementation of the approach in one and two stage designs.
TD74