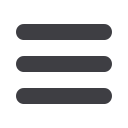

INFORMS Philadelphia – 2015
75
2 - Reliability of a Two Parallel K Out of N System with Removable
Repair Mechanism
George Mytalas, S. Lecturer, NJIT, University Heights, Newark,
NJ, United States of America,
mytalas@njit.eduWe study the reliability of a (k1,k2)-out-of-(n1,n2) system which consists of two
different types of components with finite populations and a single repair machine.
The system operates under the (N1,N2)-policy i.e. the server is activated for
exhaustive repairs. The repaired components are assumed to be as good as new.
Repair times of components and life times are assumed to be independent of each
other.
3 - Bullwhip Effect in a Pharmaceutical Supply Chain
Ming Jin, University of Utah, 1655 E Campus Center Dr, Salt
Lake City, UT, United States of America,
ming.jin@utah.edu,
Glen Schmidt, Nicole Dehoratius
We investigate the bullwhip effect in a pharmaceutical supply chain. Specifically,
we estimate the bullwhip effect at the stock keeping unit (SKU) level, analyze the
bias in aggregated measurement of the bullwhip effect, and examine various
driving factors of the bullwhip effect. Data aggregation across products or over
long time periods tends to mask the bullwhip effect in some cases. Price
promotion, order batching, and inventory are three main factors associated with
the bullwhip effect.
SB30
30-Room 407, Marriott
Model Building like a Boss
Sponsor: Analytics
Sponsored Session
Chair: Drew Pulvermacher, Director, Decision Sciences,
PerformanceG2, Inc., 3432 Sunset Dr, Madison, 53705, United States of
America,
drew@performanceG2.com1 - Descriptive, Predictive and Prescriptive Analysis for and by
Business Users
Alain Chabrier, IBM Spain, Santa Hortensia 26-28, Madrid, Spain,
achabrier@es.ibm.com,Xavier Ceugniet, Stéphane Michel
Visual analytics tools such as Watson Analytics provide easy ways for business
users to benefit of descriptive and predictive analytics. Using configuration and
elicitation of constraints and goals based on suggestions and natural language, we
show how prescriptive analytics can also be supported and more complex
business problems solved using the combinations of the 3 analytics area. We
illustrate with a campaign marketing optimization use case.
2 - The Five Minute Analyst
Harrison Schramm, Navy Headquarters Staff, 1507 22nd Street
South, Arlington, VA, 22202, United States of America,
Harrison.Schramm@gmail.comA little bit of analysis can go a long way – provided it’s both scoped appropriately
and presented in a way that is appealing to the layperson. The Five Minute
Analyst, appearing in INFORMS/Analytics Magazine, attempts to apply some
analysis to everyday problems with the goal of both improving decisions and
sharing the power of a little bit – five minutes’ worth, to be precise – of analysis
towards everyday problems. This session will be a ‘best of’, going further in depth
on the articles I’ve written, as well as discussing some ‘also rans’ – interesting
problems that did not make the column. The application areas contain (but are
not limited to) probability, statistics and game theory, although others may find
their way in. Problems addressed will include Star Wars and Downton Abbey.
There will be zombies.
SB31
31-Room 408, Marriott
Predictive Analytics for Health Care Decision Making
Sponsor: Data Mining
Sponsored Session
Chair: Nick Street, Professor And Departmental Executive Officer, The
University of Iowa, 108 Pappajohn Business Building, S210, Iowa City,
IA, 52242, United States of America,
nick-street@uiowa.edu1 - Sparse Logical Machine Learning Models
Cynthia Rudin, Associate Professor, Massachusetts Institute of
Technology, MIT, Cambridge, MA, United States of America,
rudin@mit.eduCART (Classification and Regression Trees) is possibly the most popular machine
learning method in industry. For the last few years, my research group has been
trying to build competitors for CART. I will overview our work on sparse logical
models that are direct competitors for CART. They are competitive in all ways:
accuracy, interpretability, and tractability.
2 - Using Machine Learning Approaches to Predict Patient Risk
from EHR Data
Alexander Cobian, Department of Computer Sciences, University
of Wisconsin-Madison, 1300 University Ave, Madison, WI,
53706-1532, United States of America,
cobian@cs.wisc.edu,
Mark Craven
We explore the task of learning from electronic health record (EHR) data to
predict patient risk levels for conditions of interest (asthma exacerbation and
post-operative deep venous thrombosis.) While standard risk questionnaires focus
on small numbers of known risk factors, we consider thousands of variables
elicited from the EHR in order to identify novel risk indicators. Further, our
approach attempts to discover latent variables that connect related, observed
variables.
3 - Reducing Patient Risk through Inverse Classification:
An SVM-based Method
Michael Lash, The University of Iowa, 318 MacLean Hall,
Iowa City, IA, 52242, United States of America,
michael-lash@uiowa.edu,Nick Street, Qihang Lin
In this work we propose a novel algorithm to address the problem of inverse
classification, and apply the result to a recommendation system for patient risk
minimization. We propose a mixed-integer nonlinear programming based on
SVMs that finds the optimal values of the attributes that achieve the targeted
probability of being in a desired class. The result is a flexible model that arrives at
a set of realistic recommendations to mitigate patient risk.
4 - Understanding Emergency Department 72-hour Revisits Among
Medicaid Patients
Kristin Bennett, Mathematical Sciences Dept., Rensselaer
Polytechnic Institute, 110 8th Street, AE 327, Troy, NY, 12180,
bennek@rpi.edu, James Hendler, James Ryan
We analyze emergency department (ED) usage at one hospital to understand ED
return visits within 72 hours. “Frequent flier” patients with multiple revisits
account for 47 percent of Medicaid patient revisits over a two year period.
Statistical and L1-logistic regression analysis reveals distinct patterns of ED usage
between frequent- and infrequent-patient encounters suggesting distinct
opportunities for interventions to improve care and streamline ED workflow.
SB32
32-Room 409, Marriott
Advances in Community Detection and Influence
Analysis in Social Networks
Sponsor: Data Mining
Sponsored Session
Chair: Wenjun Wang, University of Iowa, S283 Pappajohn Business
Building, Iowa City, IA, 52242, United States of America,
wenjun-wang@uiowa.edu1 - Finding Hierarchical Communities in Complex Networks
Wenjun Wang, University of Iowa, S283 Pappajohn Business
Building, Iowa City, IA, 52242, United States of America,
wenjun-wang@uiowa.edu, Nick Street
Based on a Shared-Influence-Neighbor (SIN) similarity measure, we propose two
novel influence-guided label propagation (IGLP) algorithms for finding
hierarchical communities in complex networks. One is IGLP-Weighted-Ensemble
(IGLP-WE), and the other is IGLP-Direct-Passing (IGLP-DP). Extensive tests
demonstrate superior performance of our methodology in terms of excellent
quality and high efficiency in both undirected/directed and unweighted/weighted
networks.
2 - Community Detection in Dynamic Networks with
Multiple Attributes
Xiang Li, University of Florida, Room 555, CSE Building U. of
Florida, Gainesville, FL, 32611, United States of America,
xixiang@cise.ufl.edu,My Thai
In this talk, we provide a mathematical model to qualify the relationship between
communities based on network structure and that based on the common node
attributes. We next present a dynamic and scalable algorithm to detect such
communities, based on the multiplex graph theory.
SB32