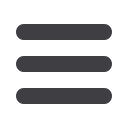

S150
ESTRO 36
_______________________________________________________________________________________________
Conclusion
We did show significant deviations between positioning
using the surface scanner and CBCT with the chosen ROI.
In this small patient cohort, 68% of the fractions would
have been out of tolerance using a threshold of 5mm and
3 degrees if positioned solely based on the surface
scanner. Therefore a surface scanner does not replace the
usual X-ray image guidance procedure. Furthermore, for
pelvic patients it does not seem possible to use the surface
scanner for reliable estimations of rotational deviations
which could have limited repeated x-ray imaging.
PV-0286 Quantifying registration uncertainties in
image-based data mining
E.M. Vasquez Osorio
1
, A. McWilliam
1,2
, J. Kennedy
3
, C.
Faivre-Finn
1,4
, M. Van Herk
1,2
1
The University of Manchester, Division of Molecular &
Clinical Cancer Studies- School of Medical Sciences-
Faculty of Biology- Medicine and Health, Manchester,
United Kingdom
2
The Christie NHS Foundation Trust, Christie Medical
Physics and Engineering, Manchester, United Kingdom
3
The Christie NHS Foundation Trust, Informatics,
Manchester, United Kingdom
4
The Christie NHS Foundation Trust, Clinical Oncology,
Manchester, United Kingdom
Purpose or Objective
Image based data mining relies on non-rigid registration to
bring image data on a common frame of reference.
Registration uncertainties will affect the analysis and must
be quantified and incorporated. We have developed a
method to quantify global and local random registration
uncertainties. Additionally, we evaluated the impact of
accounting for global random registration uncertainties on
the results of a recent lung data mining study that
identified the base of the heart as a dose sensitive region
affecting survival in lung cancer patients [1].
Material and Methods
CT data and heart delineations from 386 lung cancer
patients were used to quantify random registration
uncertainties. Inter-patient registration inclu ded an
affine and a non-rigid registration (NRR) using the first
patient in our database as reference. The affine re
gistration was initialized by scaling the clip-box that
encompassed both lungs to match the reference patient’s
clip-box and then an automatic intensity-based affine
registration was run. Subsequently a non-rigid registration
was performed using NiftyReg on the same region. Both
registrations ignored bony anatomy. Global random
registration uncertainty was estimated by assessing
standard deviation of all centres of mass of the
transformed organ of interest contours, here the
heart. Local random uncertainties on the heart surface
were estimated by calculating the standard deviation of
the distances of individual transformed delineations to the
median heart. To determine the impact of the random
registration uncertainty in our study, we compared the
results of the data mining analysis between the original
dose distributions and the Gaussian blurred dose
distributions using the global registration uncertainty
found, excluding outliers.
Results
Figure 1 summarizes the global and local random
uncertainties. The smaller local uncertainties were seen
on the lateral aspects of the heart close to the heart-lung
interface; conversely, the largest local uncertainties were
observed on the caudal regions of the heart close to the
lung-diaphragm-liver interface.
Including the random registration uncertainties in the data
mining analysis did not change the conclusions of the
study, mainly because significant regions exceeded the
registration accuracy in size (figure 2).