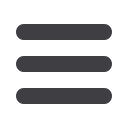

S228
ESTRO 36
_______________________________________________________________________________________________
by TME surgery and optional postoperative
chemotherapy and an experimental arm B:
short course 5
x 5 Gy radiation followed by six cycles of full-dose CAPOX
or nine cycles of FOLFOX and TME surgery.
Results
A total of 920 patients were included between June 2011
and June 2016. At randomisation, 302 were cT4 and 828
were cN+, of whom 621 were considered cN2 disease and
137 as extramesorectal pelvic lymphnodes. Based on MRI,
extramural vascular invasion was diagnosed in 275
patients, whereas the mesorectal fascia was threatened in
564 patients.
Preliminary data show that median time between
randomization and surgery was 15,9 weeks for arm A and
25,3 weeks for arm B. In arm B, 100% of the patients who
started, completed the radiotherapy and 72% of patients
completed all scheduled cycles of neoadjuvant
chemotherapy after 5x5 Gy. Another 9% of patients
completed the last course(s) without oxaliplatin. In arm A,
96% received all scheduled radiotherapy fractions and 94%
of the patients received 5 weeks of preoperative
capecitabine
combined
with
radiotherapy.
Open surgery was performed in 59% of the patients and
35% underwent an APR. In total, 19% of patients had a
ypT0N0. For 4% of all patients a wait & watch strategy was
applied. Of the operated patients, 89% had a negative
circumferential resection margin (> 1 mm).
Conclusion
Compliance for neoadjuvant treatment was good in both
treatment arms. Given the locally advanced state of most
tumors, the ypT0N0 rate can be considered satisfactory.
Final data and details concerning differences in pre-
treatment characteristics and treatments between the
two arms will be presented.
Joint Symposium: ESTRO-ESR: Radiomics and imaging
databases for precision radiation oncology
SP-0430 Radiomics in radiology, what are the
parameters of interest for different imaging
modalities?
H. Ahlström
1
1
Uppsala University, Dept of Radiology, Uppsala, Sweden
CT, MRI, PET, PET-CT and PET-MRI datasets contain huge
amounts of spatially detailed morphological, functional
and metabolic information. Today, when analysed, these
detailed datasets are typically heavily reduced to a few
measurements of a priori specified measurements of
interest
(e.g.
volumes,
areas,
diameters,
average/maximum tracer concentrations etc.) and/or
visually – and therefore inevitably subjectively – assessed
by a human operator. As a result, normality/non-normality
can only be assessed on these measurements and not on
the entire data collected, and statistical interaction with
non-imaging parameters can also be assessed only on
these a priori specified measurements. In order to utilise
the full potential of these image datasets, new analysis
tools included in the concept Radiomics, that allow
objective or quantitative assessment of all imaging data
(including e.g. previously discarded information about
texture), are needed. Radiomics can be divided into
distinct processes: (a) image acquisition and
reconstruction, (b) image segmentation and rendering, (c)
feature extraction and feature qualification and (d)
databases and data sharing with non-imaging data (e.g.
different “omics” and clinical data) for (e) informatics
analyses. Statistical knowledge of the normal range of
Radiomics features are needed for the analyses. These
analyses are anticipated to bring out new associations and
understandings that traditional approaches could not
achieve. Radiomics features can, together with non-
imaging data, be included in models that have shown to
provide valuable diagnostic, prognostic or predictive
information for oncological diseases. This information
aims at improving individual patients’ outcomes by a
better treatment selection.
SP-0431 Radiomics in radiotherapy. How is it used to
personalise treatment and to predict toxicity and/or
tumour control
C. Gani
1
1
University Hospital Tübingen Eberhard Karls University
Tübingen, Radiation Oncology Department, Tübingen,
Germany
Radiomics is defined as the automated or semi-automated
extraction of a large number of features from imaging
datasets resulting an individual “imaging phenotype”.
These features and the imaging phenotype can then be
correlated with a variety of other parameters: from
genetic phenotypes to oncological outcome data.
Radiomics as a non-invasive procedure is of particular
interest for the radiation oncologist in times of precision
radiation oncology: The radiomics phenotype might help
to identify patients at high risk for treatment failure and
therefore candidates for more aggressive treatment.
Furthermore radiomics can also be a helpful tool to
predict the risk for radiation-induced toxicities and guide
the dose distribution within normal tissues. This lecture
will give an overview about the existing data on radiomics
in the field of radiation oncology.
SP-0432 Uncertainties in imaging -how they should be
reported and propagated in prediction models using
radiomics
L. Muren
1
Aarhus University Hospital - Aarhus University, Medical
Physics, Aarhus, Denmark
Abstract not received
SP-0433 Imaging biobanks: challenges and
opportunities
A. Van der Lugt
1
1
Erasmus MC University Medical Center Rotterdam,
Department of Radiology, Rotterdam, The Netherlands
An imaging biobank can be defined as an organised
database of medical images and associated imaging
biomarkers (radiology and beyond) shared among multiple
researchers, and linked to other biorepositories. An
imaging biobank is designed for scientific use. Image data
are systematically analysed visually, manual, or (semi)-
automated with the main aim to extract imaging
biomarkers than can be related to patient characteristics
like medical history, genomic data, and outcome or
disease characteristics like genomic data, biomaterials or
response to treatment. The data storage is structured in a
way that the database can be queried and retrieved based
on available metadata. In order to exploit the available
information interactions with other databases are a
perquisite. General requirements with respect to the data
collection are therefore a database facilitating storage of
image data and metadata, storage of derived image-based
measurements, and storage of associated non-imaging
data, taking into account the need to deal with
longitudinal data, and to cope with multiple file formats.
Finally, automated retrieval is needed for image analysis
pipelines that extract image features for radiomics
signatures or for hypothesis free deep learning algorithms.