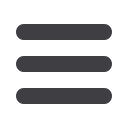

S236
ESTRO 36
_______________________________________________________________________________________________
Conclusion
Rapidplan DVH prediction is a useful tool to guide
treatment plan generation, although significant prediction
inaccuracies may occur, even when the training database
consists of as many as 114 treatment plans. Since all plans
to train and validate RapidPlan DVH prediction were
Pareto optimal, prediction errors for a database of
manually optimized treatment plans are likely larger than
presented here. For models based on a small number of
plans (N=20), prediction performance depends strongly on
the selected training patients, therefore larger models are
recommended.
OC-0445 Probabilistic optimization of the dose
coverage – applied to treatment planning of cervical
cancer
D. Tilly
1,2
, A. Holm
2
, E. Grusell
1
, A. Ahnesjö
1
1
Uppsala University Hospital, Department of
Immunology- Pathology and Genetics, Uppsala, Sweden
2
Elekta, R&D, Stockholm, Sweden
Purpose or Objective
Probabilistic optimization is an alternative to margins for
handling geometrical uncertainties in treatment planning
of radiotherapy where the uncertainties are explicitly
incorporated into the plan optimization through sampling
of treatment scenarios and thereby better exploit patient
specific geometry. In this work, a probabilistic method is
presented based on statistical measures of dose coverage,
similar to the basis for margin based planning. The idea is
that the dose planner requests a dose coverage to a
specified probability, which the algorithm then fulfils.
Material and Methods
The van Herk margin recipe is designed to deliver
sufficient target dose coverage in 90% of the treatments.
The probability is however rarely specified. We generalize
this prescription approach to include the probability
explicitly through the concept of
Percentile Dose
Coverage
(PDC), i.e. the dose coverage that is at least
fulfilled to a specified probability. The PDC used in this
work for target minimum dose criterion is the probability
for the dose-volume criteria
D
98%
to be fulfilled with 90%
probability
which
we
denote
as
D
98%,90%
.
For optimization, we make use of the
Expected Percentile
Dose Coverage
(EPDC), defined as the average dose
coverage below a given PDC. The EPDC is, in contrast to
PDC, a convex measure which allows for standard
optimization techniques to be used for finding an optimal
treatment plan. We propose an iterative method where a
treatment optimization is performed at each iteration and
the EPDC constraint tolerance is adjusted gradually until
a desired PDC is met.
We have tested our probabilistic planning method based
on datasets containing multiple imaging for four cervical
cancer patients treated with VMAT (2 Gy, 23fx). The
datasets formed the basis for a statistical shape model
(SSM) that provided the scenario specific sampled
deformations. A set of 100 scenarios sampled from the SSM
was included in the probabilistic optimization. A final
iteration using 400 scenarios was performed to increase
the resulting precision. A set of 1000 independent
scenarios not part of the optimization was used to verify
that the requested PDC was met.
Results
For all patients in this work, the iterative process of
finding the EPDC tolerance to fulfil the requested PDC
converged in less than 10 iterations to within 0.1 Gy of the
requested PDC (95% of 46 Gy = 43.7 Gy), see figure 1. The
verification calculations showed that the requested PDC
was met within 1.3%, see table 1.
Figure 1.
The convergence of
D
98%,90%
per iteration towards
the requested indicated by the dashed line. A full
probabilistic optimization is performed per iteration.
Table 1.
The D
98%,90%
after optimization and verification
calculations.
Patient
1
PDC optimization
[Gy]
PDC verification
[Gy]
1
43.4
43.2
2
43.8
43.8
3
43.4
43.4
4
43.1
43.1
Conclusion
We proved that a probabilistic planning algorithm can be
formulated such that the dose planner can request a PDC
which the algorithm attempts to fulfil. Results for datasets
of four cervical cancer patients indicate that the
requested PDC was fulfilled within 1.3%.
OC-0446 A Fully Automated VMAT Planning System
with Site-Configurable Algorithm
M. Chu
1
, R. Maggs
1
, M. Smyth
1
, R. Holmes
1
, D.G. Lewis
1
,
J. Staffurth
2
, E. Spezi
3
, A.E. Millin
1
, P.A. Wheeler
1
1
Velindre Cancer Centre, Medical Physics, Cardiff,
United Kingdom
2
Cardiff University, School of Medicine, Cardiff, United
Kingdom
3
Cardiff University, School of Engineering, Cardiff,
United Kingdom
Purpose or Objective
One of the key benefits to automation of the treatment
planning process is that consistency in plan quality can be
maintained, regardless of user experience. To ensure that
the plans are fully optimal, however, the system should
allow incorporation of clinical experience and knowledge
of the oncologist. This work presents an automated
planning system that can be configured via a novel Pareto
navigation process. A retrospective study was performed
with thirty patients across three sites: Prostate & Seminal