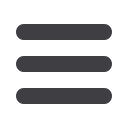

S442
ESTRO 36
_______________________________________________________________________________________________
Conclusion
RapidPlan model evolves when the sub-optimal
constituent training sets were replaced by the improved
plans that were re-optimized by the model. One iteration
is most cost-effective.
PO-0823 Hierarchical constrained optimization for
automated SBRT paraspinal IMRT planning
M. Zarepisheh
1
, L. Hong
1
, J.G. Mechalakos
1
, M.A. Hunt
1
,
G.S. Mageras
1
, J.O. Deasy
1
1
Memorial Sloan Kettering, Medical Physics, New York,
United States Minor Outlying Island
Purpose or Objective
To develop a fully automated approach to IMRT treatment
planning using hierarchical constrained optimization and
the Eclipse API for SBRT paraspinal cases.
Material and Methods
This study formulates the IMRT treatment planning
problem as a hierarchical constrained optimization (HCO)
problem (also known as prioritized optimization). HCO
prioritizes the clinical goals and optimizes them in ordered
steps. In this study, we maximize tumor coverage first and
then minimize critical organ doses in the subsequent steps
based on their clinical priorities (e.g., (1) maximize tumor
coverage, (2) minimize cord or cauda dose, (3) minimize
esophagus dose,...). For each organ we define an
objective function, based on the gEUD concept, which
correlates with the clinical criterion. At each step, we
preserve the results obtained in the prior steps by treating
them as hard constraints with a slight relaxation or ”slip”
to provide space for subsequent improvement. Maximum
dose criteria to the tumor and other organs is always
respected through hard constraints applied at all steps.
To solve the resultant large-scale constrained
optimization problems, we use two commerical solvers,
knitro
and
ampl
. The Eclipse API is used to pull patient
data needed for optimization (e.g., beam geometries,
influence matrix). After solving the optimization
problems, the optimal fluence map is imported back to
Eclipse for leaf sequencing and final dose calculation using
the Eclipse API. The entire workflow is automated,
requiring user interaction solely to prepare the contours
and beam arrangement prior to launching the HCO Eclipse
API plugin. Optimization requires ~1-3 hours, after which
the automated plan including final dose calculation is
ready in Eclipse.
Results
HCO IMRT automatic planning was tested for 10 patients
with spinal lesions who had previously been treated to 24
Gy in a single fraction using either VMAT (8 patients) or
multi-field IMRT (2 patients). All automated HCO plans
used multi-field IMRT. A typical automated and clinical
plan comparison is shown in Figure 1, demonstrating
improved PTV coverage, cord and esophagus sparing with
the automated plan. As shown in Table 1, on average, the
automated plan improved PTV coverage (V95%) by 1%,
cord maximum dose by 2%, cord D0.35cc by 12%, cauda
maximum dose by 15%, and esophagus V18Gy by 100%. All
HCO plans met all clinical planning criteria.
Table-1.
Comparison of clinical and HCO automated plans
for ten patients. For each criterion, the better score is
bolded.
Figure-1.
Comparison of the clinical and automated plans
for a patient. A1-A3 represent the automated plan and C1-
C3 represent the clinical plan.
Conclusion
Hierarchical constrained optimization shows promise as a
powerful tool to automate IMRT treatment planning. The
automated treatment plan meets all clinical criteria and
compares favorably in relevant metrics to the plan
generated by planners. Using Eclipse API, we developed a
plugin which fully automates the workflow and can be
implemented into clinical use after thorough testing.
Poster: Physics track: Treatment planning: applications
PO-0824 IMRT dose painting for prostate cancer using
PSMA-PET/CT: a planning study based on histology
K. Koubar
1,2
, C. Zamboglou
2,3
, I. Sachpazidis
1,2
, R.
Wiehle
1,2
, S. Kirste
2,3
, V. Drendel
2,4
, M. Mix
2,5
, F.
Schiller
2,5
, P. Mavroidis
6,7
, P.T. Meyer
2,5
, A.L. Grosu
2,3
, D.
Baltas
1,2
1
Medical Center University of Freiburg - Faculty of
Medicine - University of Freiburg, Division of Medical
Physics - Department of Radiation Oncology, Freiburg,