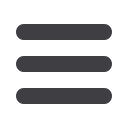

S918
ESTRO 36
_______________________________________________________________________________________________
were acquired using 3D or 4D gated PET(average image)
according to institutional settings. 14 SUV(mean) metrics
were obtained per acquisition varying VOI/ ROI shape and
location. Three ROIs and three VOIs with respective radii
of 0.5, 0.6 and 0.8cm were investigated. These ROIs/VOIs
were first centred on the maximum activity voxel; a
second analysis was made changing the location from the
voxel to the region (ROI5voxels) or the volume
(VOI7voxels) with the maximum value. Two additional
VOIs were defined as 3D isocontours respectively at 70%
and 50% of the maximum voxel value. The SUV metrics
were normalized by the corresponding 3D static SUV.
Converting to recovery coefficients (RC) allowed us to pool
data from all institutions, while maintaining focus solely
on motion. For each RC from each motion setting we
calculated the mean over institutions, we then looked at
the standard deviation (Sd) and spread of each averaged
RC over each motion setting.
Results
For the institutions visited we found that RCVOI70% and
RCVOI50%, yielded over the 14 metrics the lowest
variability to motion with Sd of 0.04 and 0.03 respectively.
The RCs based on ROIs/VOIs centered on a single voxel
were less impacted by motion (Sd: 0.08) compared to
region RCs (Sd: 0.14). The averaged Sd over the RCs based
on VOIs and ROIs was 0.12 and 0.11 respectively.
Conclusion
Quantification over breathing types depends on ROI/VOI
definition. Variables based on SUV max thresholds were
found the most robust against respiratory noise.
EP-1683 Fractals in Radiomics: implementation of new
features based on fractal analysis
D. Cusumano
1
, N. Dinapoli
2
, R. Gatta
2
, C. Masciocchi
2
, J.
Lenkowicz
2
, G. Chilorio
2
, L. Azario
1
, J. Van Soest
3
, A.
Dekker
3
, P. Lambin
3
, M. De Spirito
4
, V. Valentini
5
1
Fondazione Policlinico Universitario A.Gemelli, Unità
Complessa di Fisica Sanitaria, Roma, Italy
2
Fondazione Policlinico Universitario A.Gemelli,
Divisione di Radioterapia Oncologica- Gemelli ART,
Roma, Italy
3
Maastricht University Medical Center, Department of
Radiation Oncology, Maastricht, The Netherlands
4
Università Cattolica del Sacro Cuore, Istituto di Fisica,
Roma, Italy
5
Università Cattolica del Sacro Cuore, Department of
Radiotherapy - Gemelli ART, Roma, Italy
Purpose or Objective
A fractal object is characterized by a repeating pattern
that it displays at different size scales: this property,
known as self-similarity, is typical of many structures in
nature or inside human body (a snow flake and the neural
networks are just some examples).
The fractal self-similarity can be measured by Fractal
Dimension (FD), a parameter able to quantify the
geometric complexity of the object under analysis.
Aim of this study is to introduce in Radiomics new features
based on fractal analysis, in order to obtain new indicators
able to detect tumor spatial heterogeneity. These fractal
features have been used to develop a predictive model
able to calculate the probability of pathological complete
response (pCR) after neoadjuvant chemo-radiotherapy for
patients affected by locally advanced rectal cancer
(LARC).
Material and Methods
An home-made R software was developed to calculate the
FD of the Gross Tumor Volume (GTV) of 173 patients
affected by LARC. The software, validated by comparing
the obtained results with ImageJ, was implemented in
Moddicom, an open-source software developed in our
Institution to perform radiomic analysis.
Fractal analysis was performed applying the Box Counting
method on T2-weighted images of magnetic resonance.
The FD computation was carried out slice by slice, for each
patient of the study: values regarding mean, median,
standard deviation, maximum and minimum of the FD
distribution were considered as fractal features
characterizing the patient.
Fractal analysis was moreover extended on sub-
populations inside GTV, defined by considering the pixels
whose intensities were above a threshold calculated as
percentage of the maximum intensity value occurred
inside GTV. A logistic regression model was then
developed and its predictive performances were tested in
terms of ROC analysis. An external validation, based on 25
patients provided by MAASTRO clinic, was also performed.
The details on imaging parameters adopted are listed in
table 1.
Results
The predictive model developed is characterized by 3
features: the tumor clinical stage, the entropy of the GTV
histogram (calculated after the application of a Laplacian
of Gaussian filter with σ=0.34 mm) and the maximum FD
(maxFD) calculated for the sub-population whose
intensities are higher than 40% of the GTV maximum value.
MaxFD is the most significant parameter of the model:
higher maxFD value, typical of a more complex structure,
is correlated with less pCR probability. The model
developed showed an AUC of ROC equal to 0.77± 0.07. The
model reliability has been confirmed by the external
validation, providing an AUC equal to 0.80 ± 0.09.
Conclusion
Fractal analysis can play an important role in Radiomics:
the fractal features provide important spatial information
not only about the GTV structure, but also about its sub-
populations.Further investigations are needed to
investigate the spatial localization of these sub-
populations and their potential connection with biological
structures.