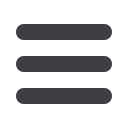

Emerging applications for AI will
depend on System-on-Chip devices
with configurable acceleration to satisfy
increasingly tough performance and
efficiency demands
As applications such as smart security,
robotics, or autonomous driving rely
increasingly on embedded Artificial
Intelligence (AI) to improve performance
and deliver new user experiences,
inference engines hosted on traditional
compute platforms can struggle to meet
real-world demands within tightening
constraints on power, latency, and
physical size. They suffer from rigidly
defined inferencing precision, bus
widths, and memory that cannot be
easily adapted to optimize for best
speed, efficiency, and silicon area. An
adaptable compute platform is needed to
meet the demands placed on embedded
AI running state-of-the-art convolutional
neural networks (CNN).
Looking further ahead, the flexibility to
adapt to more advanced neural networks
is a prime concern. CNNs that are popular
Inferencing precision
In conventional SoCs, performance-
defining features such as the memory
structure and compute precision are
fixed. The minimum is often eight bits,
defined by the core CPU, although
the optimum precision for any given
algorithm may be lower. An MPSoC
allows programmable logic to be
optimized right down to transistor level,
giving freedom to vary the inferencing
precision down to as little as 1-bit if
necessary. These devices also contain
many thousands of configurable DSP
slices to handle multiply-accumulate
(MAC) computations efficiently.
The freedom to optimize the inferencing
precision so exactly yields compute
efficiency in accordance with a square-
law: a single-bit operation executed in
a 1-bit core ultimately imposes only
1/64 of the logic needed to complete
the same operation in an 8-bit core.
Moreover, the MPSoC allows the
inferencing precision to be optimized
Adaptive Acceleration Holds the Key to Bringing AI
from the Cloud to the Edge
Dale K Hitt, Director Strategic Market Development, Xilinx Inc.
today are being superseded by new state-
of-the-art architectures at an accelerating
pace. However, traditional SoCs must be
designed using knowledge of current
neural network architectures, targeting
deployment typically about three years
in the future, from the time development
starts. New types of neural networks such
as RNNs or Capsule Networks are likely
to render traditional SoCs inefficient and
incapable of delivering the performance
required to remain competitive.
If embedded AI is to satisfy end-user
expectations, and – perhaps more
importantly – keep pace as demands
continue to evolve in the foreseeable
future, a more flexible and adaptive
compute platform is needed. This could
be achieved by taking advantage of user-
configurable multi-core System on Chip
(MPSoC) devices that integrate the main
application processor with a scalable
programmable logic fabric containing
configurable memory architecture and
signal processing suitable for variable-
precision inferencing.
26 l New-Tech Magazine Europe