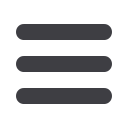

Time Blocks
Sunday - Tuesday
8:00am -9:30am
11:00am – 12:30pm
1:30pm – 3:00pm
4:30pm – 6:00pm
Wednesday
8:00am -9:30am
11:00am – 12:30pm
12:45pm -2:15pm
2:45pm – 4:15pm
4:30pm- 6:00pm
TA01
The day of
the week
Time Block.
Matches the time
blocks shown in the Program
Schedule.
Room number.
Room locations are
also indicated in the listing for each
session.
How to Navigate the
Technical Sessions
There are four primary resources to help you
understand and navigate the Technical Sessions:
• This Technical Session listing, which provides the
most detailed information. The listing is presented
chronologically by day/time, showing each session
and the papers/abstracts/authors within each
session.
• The Author and Session indices provide
cross-reference assistance (pages 518-560).
Quickest Way to Find Your Own Session
Use the Author Index (page 518) — the session code
for your presentation will be shown along with the room
location. You can also refer to the full session listing for
the room location of your session.
The Session Codes
17
Sunday, 8:00AM - 9:30AM
SA01
101A-MCC
Temporal Data Mining and Pattern Discovery
Sponsored: Data Mining
Sponsored Session
Chair: Mustafa Gokce Baydogan, Bogazici University, Bebek, Istanbul,
34342, Turkey,
baydoganmustafa@gmail.com1 - Discovering Distinct Features Using Deep Learning For
Arrhythmia Detection
Seho Kee, Arizona State University, Tempe, AZ, United States,
skee4@asu.edu, Phillip Howard, George Runger
Although domain knowledge-based features have been widely adopted in
anomaly detection studies, they still suffer from the limitations of the insufficient
known features or unavailability in practice. To address these problems, we
propose an autoencoder model that is able to discover useful features that identify
anomaly patterns in temporal heartbeat data without assuming any prior
knowledge. The results show that the discovered features obtained from just a
two-dimensional projection layer can effectively distinguish abnormal beats from
normal beats without training on pre-labeled data.
2 - Process Control For Time-varying Situations
Seoung Bum Kim, Korea University,
sbkim1@korea.ac.kr,
Seulki Lee
In modern manufacturing systems containing the complexity and variability of
processes, appropriate control chart techniques that can efficiently handle the
nonnormal and nonlinear processes are required. In this talk, I will present some
recently developed multivariate control charts to handle both nonnormal and
time-varying process situations.
3 - On The Use Of Support Vectors For Time Series
Pattern Discovery
Mustafa Gokce Baydogan, Bogazici University, Istanbul, Turkey,
mustafa.baydogan@boun.edu.tr,Mehmet R Kamber,
Erhun Kundakcioglu
Similarity search and classification on time series (TS) databases has received
great interest over the past decade. The definition of similarity between TS is a
major problem in this context. Nearest-neighbor (NN) classifers are widely used
for TS classification but these approaches compute the similarity over the whole
TS which might be problematic with the long TS and relatively short features of
interest. Moreover, these classifiers are not directly interpretable as they do not
describe why a TS is assigned to a certain class. This study utilizes margin
maximization to discover the regions of the time series that have potentially
representative patterns related to the classification task.
4 - Machine Learning For Predicting Heart Failure Readmission
Wei Jiang, Research Assistant, Johns Hopkins University,
3400 N Charles St, Baltimore, MD, 21218, United States,
wjiang1990@gmail.com,Scott R Levin, Lili Barouch,
Frederick Korley, Sauleh Ahmad Siddiqui, Diego A. Martinez,
Matthew Toerper, Sean Barnes, Eric Hamrock
Predicting risk of heart failure (HF) readmission has gained increasing attention,
with existing studies mainly using administrative data. We will focus on using
clinical data from EMR for predicting HF readmission by doing pattern
recognition with time series clinical data. We will then use classification models
for predicting the drivers of readmission.
T
E C H N I C A L
S
E S S I O N S
Rooms and Locations /Tracks
All tracks / technical sessions will be held in the Music
City Center and Omni Hotel. Room numbers are shown
on the Quick Reference and in the Technical session
listing.