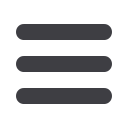

INFORMS Nashville – 2016
22
SA15
3 - Response Surface Methodology In Plant Breeding
Reka Howard, University of Nebraska – Lincoln, NE,
rekahoward@gmail.com, William Beavis, Alicia Carriquiry
We introduce Response Surface Methodology (RSM) as a strategy to find the
combination of attribute levels that results in accurate predictions for a given
genomic prediction (GP) method, and compare GP methods. We illustrate RSM
with a simulated example where the response we optimize is the difference
between prediction accuracy using the parametric best linear unbiased prediction
(BLUP) and the nonparametric support vector machine (SVM). The greatest
impact on the response is due to the genetic architecture of the population and
the heritability. When epistasis and heritability are highest, the advantage of using
the SVM versus the BLUP is greatest.
4 - A New Genomic Selection Approach
Lizhi Wang, Iowa State University,
lzwang@iastate.edu,Matthew Goiffon, Guiping Hu, Aaron Kusmec, Patrick Schnable
Conventionally, plant breeders make selection decisions based on phenotype
observations and intuitive judgement. The advent of genotyping techniques
provides breeders with much more informative genomic data. However, the
enormous volume and complexity of the genomic data also present great
challenges in extracting the useful information deeply buried in the mountains of
data. We present a new approach for genomic selection and demonstrate its
improvement over previous methods using computer simulation with realistic
genomic data.
SA15
104E-MCC
Big Data in the E-Commerce Deliveries
Invited: Modeling and Methodologies in Big Data
Invited Session
Chair: Chung-Yee Lee, HKUST, IELM Dept. HKUST, Clear Water Bay,
Hong Kong, 0000, Hong Kong,
cylee@ust.hk1 - The Benefits Of Randomization In Warehousing And Logistics
John Carlsson, University of Southern California,
jcarlsso@usc.eduA recent innovation in warehousing and logistics has been the use of
randomization, such as Amazon’s random stow, in which warehouse items are
scattered throughout the floor map as opposed to being concentrated in one area.
We use a continuous approximation model to describe how such a policy is
beneficial in the long run.
2 - Resource Allocation With Unmanned Aerial Vehicle
Siyuan Song, University of Southern California,
siyuanso@usc.edu,
John Gunnar Carlsson
Unmanned aerial vehicles, commonly known as drones, have become more
widely utilized in delivery nowadays. We study the efficiency of a so-called
‘horsefly’ delivery system, in which drones are used in conjunction with truck.
We propose a mathematical formulation of a ‘horsefly’ problem followed by some
general properties of optimal solutions. Then some approximation results,
including an approximation algorithm, are given to illustrate the benefit of
horsefly system on a large scale. Lastly, we compare some practical heuristic
algorithms in different scenarios for best choice in each case.
3 - The Last Mile Rush
Song Zheng, Cainiao Network, Hang Zhou, China,
zhengsong.zs@alibaba-inc.com, Lijun Zhu
As e-commerce keeps its impressive growth, a large percentage of express orders
are generated by e-commerce. In China, for example, it is over 60 percent.
Increasing investments rush into China’s express delivery industry, which now
has thousands of delivery companies and millions of delivery workers. Alibaba
group and Cainiao Network are building China Smart Logistics Network and
developing a huge ecosystem with all major logistics companies in China. We will
present an optimal solution to the last mile delivery, more specifically, arranging
thousands of courier to delivery all kinds of packages in cities including online e-
commerce packages and offline O2O packages.
SA16
105A-MCC
Inverse Optimization: Theory
Sponsored: Optimization, Optimization Under Uncertainty
Sponsored Session
Chair: Taewoo Lee, Rice University, #217, 7010 Staffordshire Street,
Houston, TX, 77030, United States,
taewoo.lee@utoronto.caCo-Chair: Timothy C.Y. Chan, University of Toronto, Toronto, ON,
Canada,
tcychan@mie.utoronto.ca1 - Goodness-of-fit In Multi-point Inverse Optimization Optimization
Rafid Mahmood, University of Toronto, Toronto, ON, Canada,
rafid.mahmood@mail.utoronto.ca, Timothy Chan, Taewoo Lee,
Daria Terekhov
Inverse optimization is a model fitting technique that uses observed points to
impute the cost function of an unknown optimization problem. Applications of
inverse optimization often rely on ad-hoc or informal methods to evaluate the fit
quality of the inverse solution to the data. A previous work introduced a general
formulation for inverse optimization with a single observation and a measure for
the goodness-of-fit. We extend both of these results to the case of multiple
observed points. Our techniques are capable of comparing different models and
identifying outliers that do not fit well with the remaining points.
2 - Inverse Optimization For Determining Constraint Parameters
Neal Kaw, University of Toronto, Toronto, ON, Canada,
neal.kaw@mail.utoronto.ca,Timothy Chan
Most inverse optimization literature has focused on determining the objective
function of an optimization problem, given an observed solution. In this work, we
develop inverse optimization models that additionally determine unspecified
parameters of the feasible set. First, we propose an inverse linear programming
model to determine all problem data. Second, we propose inverse robust linear
programming models to determine a cost vector and unspecified parameters of
the uncertainty set, for two types of uncertainty: interval uncertainty and
cardinality constrained uncertainty.
3 - Robust Inverse Optimization
Kimia Ghobadi, MIT, Cambridge, MA, United States,
kimiag@mit.edu,Daria Terekhov, Houra Mahmoudzadeh,
Taewoo Lee
In this talk, we explore the robustification of inverse optimization. Our work is
motivated by problems in which the observation of the solution is partial, noisy,
or uncertain. We build an uncertainty set around the observation and derive an
inverse model that finds a cost vector that protects against the worst case scenario
in the given uncertainty set. Our model generalizes previous work on single-
observation inverse models. It can also be seen as more general than inverse
optimization with multiple points, since the points can be thought of as a sample
from the uncertainty set.
SA17
105B-MCC
Optimal Statistical Learning
Sponsored: Optimization, Optimization Under Uncertainty
Sponsored Session
Chair: Nana Kwabena Aboagye, Princeton University (ORFE), 1 Nassau
Hall, Princeton, NJ, 08544, United States,
aboagye@princeton.edu1 - Uncertain Date Envelopement Analysis
Allen Holder, Rose-Hulman Institute of Technology,
holder@rose-hulman.eduWe motivate an inverse optimization problem that calculates a decision making
unit’s maximum efficiency within the context of uncertain data envelope
analysis. One of the sub-problems is a robust linear program, but unlike a
traditional robust model that sacrifices the objective to hedge against uncertainty,
the data envelope model leverages uncertainty to promote efficiency. We apply
the method to a set of prostate radiotherapy treatments to help discern
appropriate treatments.
2 - Optimal Learning Of Expensive Quadratic Functions
Nana Kwabena Aboagye, Princeton University,
aboagye@princeton.eduWe study the problem of learning the unknown parameters of an expensive
function where the true underlying surface can be described by a quadratic
polynomial. We present a previously studied Bayesian optimization algorithm
known as the knowledge gradient for the parametric belief model. Originally
established in the limited context of drug discovery (see Negoescu et al. (2011),
the knowledge gradient for the parametric belief model remains under-studied
with regards to its behavior. We seek to understand the behavior of this algorithm
and exploit this understanding to derive a simple heuristic that performs just as
well as the knowledge gradient for the parametric belief model.