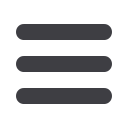

INFORMS Nashville – 2016
183
MC02
101B-MCC
Data Mining Innovations in Healthcare
Sponsored: Data Mining
Sponsored Session
Chair: Michael Lash, University of Iowa, S210 John Pappajohn
Business Building, Iowa City, IA, 52242-1000, United States,
michael-lash@uiowa.edu1 - Multi-site Evidence-based Best Practice Discovery – Finding
Factors That Influence Treatment Outcome
Eva Lee, Georgia Tech,
evakylee@isye.gatech.eduCody Wang
This work is joint with Care Coordination Institute. The work focuses on
establishing inter-operability among electronic-medical-records(EMRs) from 737
providers for large-scale data mining to identify discriminatory characteristics that
can predict the quality of treatment outcome. We demonstrate the system
usability by analyzing Type II diabetic patients. DAMIP establishes a classification
rule on a training set that results in greater than 80% predictive accuracy on a
blind set of patients. This facilitates evidence-based treatment and optimization of
site performance through best practice dissemination and knowledge transfer.
2 - Scalable Support Vector Machines For Massive
Healthcare Datasets
Talayeh Razzaghi, Clemson University,
trazzag@g.clemson.eduSolving the optimization model of support vector machines is often an expensive
computational task for massive healthcare training sets. We propose an efficient,
effective, multilevel algorithmic framework that scales to very large data sets. Our
multilevel framework substantially improves the computational time without
loosing the quality of classifiers for balanced and imbalanced datasets.
3 - Exploring Feasibility To Early Detect Alzheimer’s Disease (AD)
And Dementia Progression Using Big Data + Machine
Learning Approach
Chih-Lin Chi, University of Minnesota, Minneapolis, MN, United
States,
cchi@umn.edu, Wenjun Zeng, Wonsuk Oh, Soo Borson
We aim to develop a 3-step data strategy to develop a model to predict long-term
cognitive changes for AD. We show results from the first step: exploring feasibility
of long-term prediction by optimizing time-varying risk factors. The developed
model predicts cognitive scores and annual changes in up to 8 years when most
subjects were cognitive normal or mild cognitive impairment. This model
demonstrates accurate prediction of how dementia progress for cognitive stable,
mild deterioration, moderate deterioration, and sharp deterioration subgroups.
The presentation will also discuss the next steps that aim at converting the data-
research results into informatics tools.
MC03
101C-MCC
Health Care, Modeling I
Contributed Session
Chair: Sina Faridimehr, PhD Student, Wayne State University,
4815 Fourth Street, Detroit, MI, 48202, United States,
sina.faridimehr@wayne.edu1 - Measure And Predict Medication Adherence Behavior Using
Administrative Data
Shan Xie, Purdue University, 315 N Grant Street, W Lafayette, IN,
47907, United States,
xie34@purdue.edu,Yuehwern Yih
For patients with diabetes, poor adherence to medication has been associated
with suboptimal glycemic control, increased health care costs and adverse health
outcomes. Thus, improving medication adherence is important to realize the full
benefit of medication therapies. The existing measures of medication adherence
based on administrative data only provide an aggregate number, which lack the
ability to distinguish between different adherence behaviors. This study will
develop an analytic framework to quantify and predict medication adherence
patterns, and provide useful information to efficiently target patients at high risk
and customize adherence improvement interventions.
2 - Improving Access To Healthcare By Minimizing
Appointment Delays
Ashley N. Anhalt, PhD Student, University of Pittsburgh, 525 S.
Aiken Avenue, APT #3, Pittsburgh, PA, 15232, United States,
ana88@pitt.edu,Jeffrey P. Kharoufeh
Providing patients with timely access to healthcare is an important issue for major
healthcare providers. One major problem is that patients are often unable to
schedule appointments with specialists in a reasonable time. We present queueing
models and decision tools to find effective appointment scheduling strategies with
the objective of maximizing the likelihood that a majority of patients can be seen
within a time threshold.
3 - Managing Access To Primary Care Through Advanced Scheduling
Sina Faridimehr, PhD Student, Wayne State University,
4815 Fourth Street, Detroit, MI, 48202, United States,
sina.faridimehr@wayne.edu, Ratna Babu Chinnam,
Saravanan Venkatachalam
In this research, we develop a scheduling framework that employs stochastic
programming to improve access to care within primary care clinics. The model
leverages correlations between scheduling practice, continuity of care,
appointment utilization and access performance. Results from testing the models
at VA facilities are promising.
MC04
101D-MCC
Optimal Procurement, Tariff, and Cybersecurity in
Smart Grid
Sponsored: Energy, Natural Res & the Environment,
Energy I Electricity
Sponsored Session
Chair: Lawrence V Snyder, Lehigh University, 200 West Packer Avenue,
Bethlehem, PA, 18015, United States,
lvs2@lehigh.edu1 - Optimal Day-ahead Power Procurement With Renewable Energy,
Storage, And Demand Response
Soongeol Kwon, Texas A&M University, College Station, TX,
United States,
soongeol@tamu.edu, Natarajan Gautam,
Lewis Ntaimo
Motivation of this research stems from pressing issues related to reducing energy
cost, specifically focused on demand-side. From energy consumers perspective,
there exist opportunities to reduce energy cost by adjusting purchase and
consumption of energy in responding to time-varying electricity prices while
utilizing renewable energy with energy storage. Considering this scenario, the
main research objective is to develop a decision model to determine optimal day-
ahead purchase commitment while considering real-time adjustments in response
to variability and uncertainty in actual power demand, renewable supply, and
electricity price.
2 - A Game Theoretic Analysis Of Electricity Time-of-use (TOU) Tariff
For Residential Customers
Dong Gu Choi, Pohang University of Science and Technology,
Pohang, Korea, Republic of,
dgchoi@postech.ac.kr, Valerie Thomas
We properly formulate a game-theoretic model for analyzing not only the optimal
behaviors of both an electric utility and residential customers but also their
monetary gains or losses under a TOU tariff. With two heterogeneous customer
types in terms of consumption pattern, we identify that a win-win situation is not
possible. Also, we emphasize our analytic results by describing a numerical
example, and we discuss the implications of our results for electric utilities and
regulatory agencies.
3 - Risk Assessment And Network Optimization For
Smart Grid Cybersecurity
Lawrence V Snyder, Lehigh University,
lvs2@lehigh.edu,Jiyun Yao, Parv Venkitasubramaniam, Shalinee Kishore,
Rick Blum
Mesh communication networks are widely used to facilitate communication to
and between smart grid sensors such as advanced meters and demand response
devices. Despite this wide use, a comprehensive risk assessment of cyber attacks
on distributed networks has not been fully explored. In this work, we propose a
framework for connectivity analysis of smart grid sensor networks to ensure
robust communication when a given number of communication nodes are
compromised. We also propose an efficient algorithm to construct a graph to meet
given connectivity criteria by augmentation of communication links or strong
authentication on certain nodes.
4 - Electricity Market Clearing With Enhanced Dispatch Of Wind
Producers: Market Design And Environmental Implications
Ali Daraeepour, Duke University, Durham, NC, 27708,
United States,
a.daraeepour@duke.edu, Dalia Patino-Echeverri
This study explores the market design, operational, and environmental effects of
the stochastic electricity market clearing. We propose a framework that allows a
robust assessment of the relative advantages of the stochastic market clearing
with respect to the conventional deterministic mechanism under wind production
uncertainty. Using a stylized version of PJM, the two mechanisms are compared
in terms of air emissions, wind integration, prices and supply-side revenue
adequacy, and out-of-market adjustments.
MC04