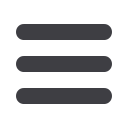

INFORMS Nashville – 2016
187
2 - Scheduling Commercial Spots Using Mixed Integer Programming
Vivek Vasudeva, Turner Broadcasting System, Inc.,
Atlanta, GA, United States,
vivek.vasudeva@turner.com,
Jose Antonio Carbajal Orozco, Wassim (Wes) Chaar
A broadcasting company sells airtime to advertisers to air their commercial spots
in accordance with certain agreed-upon rules. We examine a mixed integer
programming-based formulation that can be used to schedule spots such that a
desired measure can be optimized while honoring the deal rules. We also analyze
how the measure changes as we relax different sets of constraints, to gain insights
into the relative impact of these constraints on the optimization measure.
MC15
104E-MCC
Disaster and Emergency Management I
Contributed Session
1 - Apropos Resilient System Design
Henry Lester, University of South Alabama, P.O. Box 8172,
Mobile, AL, 36689, United States,
hlester@southalabama.eduSystem resiliency signifies the ability to resist and recover from an extreme event.
Critical measures of this resiliency are extreme event damages and disaster
recovery time. This paper presents an analytical approach to capturing extreme
event system behaviors with respect to system resiliency in order to predict
disaster recovery time. The approach isolates significant system factors to estimate
recovery function parameters to enhance a resilient system design. The resultant
resilient system design can provide situational awareness for future decision
analysis pertaining system deployment and operations while subject to extreme
events.
2 - A Follow-Up Sharing Method For Post-Event Response Resource
Distribution With Group Information Updates
Yong Ye, Wenzhou Medical University, Chashan Street, Ouhai
District, Wenzhou, 325035, China,
yong_ye@foxmail.com,
Guiling Liu, Lingle Pan
This paper addresses a Follow-up Sharing Character (FSC), which coordinates
resources between different phases. Based on FSC, this paper proposes a general
model by minimizing the RAEL (the losses caused by the mismatch between
supply and demand in impacted areas) of all phases, the RAEL of all affected areas
in the present phase, and the ELTL of the distribution plan in the present phase.
We also apply the Bayesian information updates approach to deal with
uncertainties of demand and traffic condition, by using historical and sample
information. Then, a solution algorithm is proposed to solve the model; and a
simulation study is presented. Insights derived from the model are provided in the
conclusion.
MC16
105A-MCC
Data-Driven Optimization Methods
Sponsored: Optimization, Optimization Under Uncertainty
Sponsored Session
Chair: Vishal Gupta, University of Southern California, University Park
Campus, Los Angeles, CA, 90089, United States,
guptavis@usc.edu1 - Machine Learning & Portfolio Optimization
Gah-Yi Ban, London Business School,
gban@london.edu,Noureddine El Karoui, Andrew Lim
We adapt two machine learning methods, regularization and cross-validation, for
portfolio optimization. First, we introduce performance-based regularization
(PBR), where the idea is to constrain the sample variances of the estimated
portfolio risk and return. We consider PBR for both mean-variance and mean-
CVaR problems. We show that the PBR models can be cast as robust optimization
problems with novel uncertainty sets and establish asymptotic optimality of both
Sample Average Approximation (SAA) and PBR solutions and the corresponding
efficient frontiers. We also develop new, performance-based k-fold cross-
validation algorithms.
2 - Smart Predict Then Optimize
Paul Grigas, UC Berkeley, 4177 Etcheverry Hall, University of
California, Berkeley, CA, 94720-1777, United States,
pgrigas@berkeley.edu, Adam Elmachtoub
We consider a class of optimization problems where the objective function is not
explicitly provided, but contextual information can be used to predict the
objective based on historical data. A traditional approach would be to simply
predict the objective based on minimizing prediction error, and then solve the
corresponding optimization problem. Instead, we propose a prediction framework
that leverages the structure of the optimization problem that will be solved given
the prediction. We provide theoretical, algorithmic, and computational results to
show the validity and practicality of our framework.
3 - Distribution Sensitivities For Quantile, Distortion Risk Measure,
And Inference
Yijie Peng, Fudan University,
pengy10@fudan.edu.cnMichael Fu
We treat quantile sensitivity, sensitivity of distortion risk measure, and statistical
inference under a single umbrella of distribution sensitivities. A new stochastic
derivative estimation technique called generalized likelihood ratio method is
proposed to address three applications in a uniform manner. We illustrate
advantages of the proposed method over existing stochastic derivative estimation
techniques for distribution sensitivities estimation, and provide supporting
numerical evidences.
4 - Small Data Optimization
Vishal Gupta, University of Southern California,
guptavis@usc.edu,
Paat Rusmevichientong
Notwithstanding press about “Big Data,” many real-world problems exhibit both a
large number of uncertain parameters and a small amount of data per parameter.
We propose a novel approach to linear optimization in this “small-data regime”
inspired by empirical Bayes methods. Our approach uses the large-scale structure
to circumvent the insufficient data; as the size of the optimization tends to infinity
while the amount of data remains fixed, our approach performs comparably to an
oracle best-in-class policy. Other popular methods do NOT enjoy this property.
Empirical evidence confirms that our approach significantly outperforms state-of-
the-art data-driven methods in this small-data regime.
MC17
105B-MCC
Robust Optimization
Sponsored: Optimization, Optimization Under Uncertainty
Sponsored Session
Chair: Anirudh Subramanyam, Carnegie Mellon University,
5000 Forbes Avenue, Pittsburgh, PA, 15213, United States,
asubramanyam@cmu.edu1 - Exploiting The Structure Of Two-stage Robust Optimization
Models With Integer Adversarial Variables
Seyed Hossein Hashemi Doulabi, Polytechnique Montréal,
Montreal, QC, Canada,
hashemi.doulabi@polymtl.caPatrick Jaillet, Gilles Pesant, Louis-Martin Rousseau
This paper addresses a class of two-stage robust optimization models with integer
variables in the adversary’s problem. We apply Dantzig-Wolfe decomposition to
exploit the structure of these models and show that the original problem reduces
to a single-stage robust problem. We propose a Benders algorithm for the
reformulated problem. Since the master problem and subproblem in the Benders
algorithm are mixed integer programs it is computationally burdensome to
optimally solve them in each iteration of the algorithm. Therefore, we develop
novel stopping conditions for these mixed integer. Some computational
experiments are performed on a two-stage nurse planning problem.
2 - A New Algorithmic Framework For Two-stage K-adaptable Robust
Optimization Problems With Mixed-integer Recourse
Anirudh Subramanyam, Carnegie Mellon University, Pittsburgh,
PA, 15213, United States,
asubramanyam@cmu.eduWolfram Wiesemann, Chrysanthos Gounaris
We present a new algorithm for solving K-adaptability versions of two-stage
robust mixed-integer linear programs (MILPs), in which we commit to K recourse
policies here-and-now and implement the best policy once the uncertain
parameters are observed. Viewing such problems as semi-infinite disjunctive
MILPs, our framework is able to address mixed-integer and random recourse in
K-adaptability problems for the first time. It is also able to incorporate tailored
solution approaches for the corresponding deterministic problems and
decomposition techniques widely used in stochastic programming. We conduct
extensive numerical experiments on benchmark data from a number of popular
applications.
3 - Weekly Two Stage Robust Generation Scheduling For
Hydrothermal Power Systems
Hossein Dashti, University of Arizona,
hdashti@email.arizona.edu,
Antonio J. Conejo, Ruiwei Jiang, Jianhui Wang
As compared to short-term forecasting, it is challenging to accurately forecast the
volume of precipitation in a medium-term horizon. As a result, fluctuations in
water inflow can trigger generation shortage and electricity price spikes in a
power system with major hydro resources. In this work, we study a two-stage
robust scheduling approach for a hydrothermal power system. We consider water
inflow uncertainty and employ a vector autoregressive (VAR) model to represent
its seasonality and construct an uncertainty set in the robust optimization
approach. We design a Benders’ decomposition algorithm to solve the problem.
Results are presented for the proposed approach on a real-world case study.
MC17