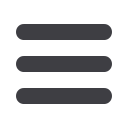

INFORMS Nashville – 2016
189
2 - Supporting The Decision Making Process In Safety Review
Taxiarchis Botsis, FDA/CBER/OBE,
Taxiarchis.Botsis@fda.hhs.govMedical experts at the US Food and Drug Administration (FDA) conduct
surveillance of licensed medical products to assure continued safety. The review
process requires the thorough evaluation of multiple parameters and can be time-
consuming for the end users. To assist them in this process, the FDA has
developed a Decision Support Environment that extracts key clinical (and time)
information from the texts, normalizes it to medical codes, and visualizes it in
meaningful manners. It also allows for other analysis, case management and
report generation.
3 - The Impact Of Transfusing Newer Blood Versus Current Practice
On The US Blood Supply
Hussein Ezzeldin, FDA/CBER/OBE, Silver Spring, MD,
United States,
hussein.ezzeldin@fda.hhs.gov, Richard Forshee,
Arianna Simonetti
The prolonged storage of red blood cells (RBCs) may be associated with
transfusion adverse events. Mixed findings between observational and clinical
studies demand larger and better-designed studies. We enhanced the US blood
supply model by implementing an adaptive feedback-control mechanism, where
blood collection is dynamically adjusted, to maintain inventory collector’s levels
and overcome potential shocks. If any benefits in transfusion outcomes of
younger RBCs are proven, an increase in their demand may be expected. We
evaluate the impact of such changes on the US blood system with respect to
current blood transfusion practice.
4 - Optimal Resource Allocation For Adaptive Clinical Trials
Alba Rojas-Cordova, Virginia Tech, Blacksburg, VA, 24060,
United States,
albarc@vt.edu, Ebru Korular Bish
Certain adaptive designs allow decision-makers to alter the course of a clinical
trial based on revised estimates of a drug’s probability of technical success. We
develop a stochastic dynamic programming model to analyze the resource
allocation decision, of continuing or stopping a trial, based on frequent data
updates. We determine the structure of the optimal resource allocation strategy
and support our findings with a numerical analysis.
MC22
107B-MCC
Cost, Safety and Resource Allocation In Health
Systems
Invited: ORinformed Healthcare Policies
Invited Session
Chair: Retsef Levi, MIT, Cambridge, MA, United States,
retsef@mit.edu1 - Data-driven Approaches To Improve The U.S. Kidney
Allocation System
Nikolaos Trichakis, MIT, Cambridge, MA, United States,
ntrichakis@mit.edu, Chaithanya Bandi, Phebe Vayanos
We present a data-driven optimization approach to estimate wait times for
individual patients in the U.S. Kidney Allocation System, based on the very
limited system information that they possess in practice. To deal with this
information incompleteness, we develop a novel robust optimization analytical
framework for wait time estimation in multiclass, multiserver queuing systems.
We calibrate our model with highly detailed historical data and illustrate how it
can be used to inform medical decision making and improve patient welfare.
2 - Optimization-driven Framework To Understand Healthcare
Networks Cost And Resource Allocation
Fernanda Bravo, UCLA Anderson School of Management, Los
Angeles, CA, United States,
fernanda.bravo@anderson.ucla.eduMarcus Braun, Vivek Farias, Retsef Levi
Consolidation in the US healthcare industry has resulted in the formation of large
delivery networks. However, integration remains uncertain. In order for large
care providers to best utilize their growing networks, it will be critical to
understand not only system-wide demand and capacity, but also how the
deployment of limited resources can be improved. We develop an optimization-
driven framework, inspired by revenue management, to understand network
costs and provide solutions to strategic problems, such as access, resource
deployment, and case-mix in multi-site networks. In collaboration with a
network of hospitals, we demonstrate our framework applicability.
3 - New Data-driven Approach To Safety And Risk Management In
Health Systems
Retsef Levi, Professor of Operations Management, MIT, 100 Main
Street, BDG E62-562, Cambridge, MA, 02142, United States,
retsef@mit.edu, Patricia Folcarelli, Yiqun Hu, Daniel Talmor,
Jeffrey Adam Traina
We present an innovative system approach to safety in Health Systems. The
approach is based on the innovative concept of risk drivers, which are states of
the System, its environment and its staff that affect the likelihood of harms, as
well as an innovative aggregated measure of the ‘burden of harm’. Using large
scale data we develop statistical models that identify predictive risky states.
MC23
108-MCC
Healthcare Delivery Modeling
Sponsored: Health Applications
Sponsored Session
Chair: Bryan A Norman, University of Pittsburgh, 1006 Benedum Hall,
Pittsburgh, PA, 15261, United States,
banorman@pitt.edu1 - Modeling To Enhance The Nurse Handoff Process
Anna Svirsko, University of Pittsburgh,
ACS167@pitt.eduBryan A Norman, David Rausch, Emily Shawley
Nurses in emergency departments often rotate between different zones during
their 12 hour shift to balance nurse workload. However, this rotation significantly
increases the number of nurse handoffs which reduces the amount of available
nurse-patient time and can result in errors in patient care. This model reduces the
number of nurse handoffs while still allowing nurses to rotate during their shift
and balancing workload among nurses. Furthermore, we look to reduce long
chains and cycles that can occur that hinder the rotation and handoff processes.
The effectiveness of the model is demonstrated by applying the model to the
nursing schedule from a local hospital.
2 - Closed-Form Solutions For Periodic Review Inventory
Systemsin Healthcare
Nazanin Esmaili, University of Pittsburgh, Pittsburgh, PA, United
States,
nae22@pitt.edu, Bryan A Norman, Jayant Rajgopal
Most inventory management systems at points of use in hospitals are
characterized by stochastic demand, periodic reviews, fractional lead time,
expedited delivery, limited storage capacity, and service level requirements. We
develop discrete time Markov chain models for such systems to minimize the total
expected replenishment effort. We derive closed form expressions and propose an
exact algorithm to calculate the limiting probability distribution by locally
decomposing the state space. We investigate the structural results and the
tradeoffs of performance measures of interest for different policies and show that
the computational effort is less than other algorithms from the literature.
3 - Considering No-shows And Procedure Time Variability When
Scheduling Endoscopy Patients
Karmel Shehadeh, University of Michigan, Ann Arbor, MI,
United States,
ksheha@umich.edu, Amy Cohn, Sameer Saini,
Jacob Kurlander
We consider the problem of how to schedule patients for colonoscopy
appointments, recognizing both the high frequency of no-shows and the
significant variability in procedure time. We review the clinic process flow,
identify metrics for evaluating schedule quality, and simulate different scheduling
approaches.
4 - Improving Healthcare Resource Management Through Demand
Prediction And Staff Scheduling
Nazanin Zinouri, Clemson University, 269 Freeman Hall, Clemson,
SC, 29634, United States,
nzinour@g.clemson.edu,Kevin Taffe
Staff scheduling in healthcare is very challenging. Hospitals typically operate 24
hours a day, 7 days a week, and are faced with high fluctuations in demand. We
developed an ARIMA model to forecast daily patient volumes a month in
advance. This information was used to compute workload and solve staff
scheduling problems. We used a risk adverse approach to find a feasible nurse
assignment that minimizes labor costs and to avoid risky cases, i.e., highly
overstaffed or understaffed. The liabilities of overstaffing and understaffing are
many. Overstaffing increases payrolls and results in excessive idle times, while
understaffing will negatively impact patient outcomes and results in loss of
revenue.
MC23