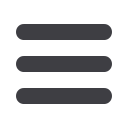

INFORMS Nashville – 2016
263
3 - System Optimal Transit Assignment With Flow-dependent Dwell
Times
Alireza Khani, University of Minnesota, 136 Civil Engineering
Building, 500 Pillsbury Drive S.E., Minneapolis, MN, 55455,
United States,
akhani@umn.eduTravel time of transit vehicles between stops is constant with respect to passenger
flow. However, dwell time at stops changes by more boarding and alighting of
passengers. This makes the transit assignment an asymmetric problem. To model
this phenomenon, an optimization problem is developed and its mathematical
properties are investigated.
TA90
Broadway D-Omni
Opt, Stochastic II
Contributed Session
Chair: Xiting Gong, Assistant Professor, The Chinese University of Hong
Kong, Room 506, William M. W. Mong Engineering Building, Shatin
N.T., Hong Kong,
xtgong@se.cuhk.edu.hk1 - Modeling AS/RS Travel In Order Picking Applications
Jingming Liu, Research Assistant, 1986, 920 N Leverett, Apt 824,
Fayatteville, AR, 72701, United States,
jl011@uark.edu,
John A White
An order picking operation is modeled as an M|G|1 queueing problem, with the
S/R machine being the server and order picking stations being customers. Service
time is the time required for an automated storage and retrieval machine to travel
from an order picking station to a random storage location and, then, to an order
picking station. To obtain the variance for service time, the density function is
derived. Because the two travel times are statistically dependent random
variables, results obtained previously for S/R travel do not apply. Random
demands for replenishment of order picking stations and Chebyshev travel by the
S/R machine are assumed.
2 - Stochastic Quasi-newton Methods For Non-strongly Convex
Problems: Convergence And Rate Analysis
Farzad Yousefian, Assistant Professor, Oklahoma State Univeristy,
317D Engineering North, School of Industrial Engineering &
Management, Stillwater, OK, 74074, United States,
farzad.yousefian@okstate.edu, Angelia Nedich, Uday Shanbhag
Motivated by applications in machine learning, we consider stochastic quasi-
Newton (SQN) methods. Traditionally, the convergence of SQN schemes relies on
strong convexity. To our knowledge, no rate statements exist in the absence of
this assumption. We consider merely convex problems and develop an SQN
scheme where both the gradient mapping and the Hessian approximation are
regularized and updated in a cyclic manner. Under suitable assumptions on the
stepsize and regularization parameters, the convergence is shown in both almost
sure and mean senses and the rate of convergence is derived in terms of function
value. The empirical results on a binary classification problem are promising.
3 - Optimization With Reference-based Almost
Stochastic Dominance
Jian Hu, Assistant Professor, University of Michigan - Dearborn,
4901 Evergreen Rd., Dept. of IMSE,, Dearborn, MI, 48128,
United States,
jianhu@umich.eduStochastic dominance is a preference relation of uncertain prospect defined over a
class of utility functions. While this utility class represents basic properties of risk
aversion, it includes some extreme utility functions rarely characterizing a
rational decision maker’s preference. We introduce reference-based almost
stochastic dominance (RSD) rules which well balance the general representation
of risk aversion and the individualization of the decision maker’s risk preference.
We also propose RSD constrained stochastic optimization model and develop an
approximation algorithm based on Bernstein polynomials.
4 - Balancing Flexibility And Inventory In Workforce Planning With
Learning And Uncertain Demand
Silviya Valeva, University of Iowa, 710 Carriage Hill, Apt 5,
Iowa City, IA, 52246, United States,
silviya-valeva@uiowa.edu,Barrett Thomas, Mike Hewitt
Explicitly modeling human learning in task assignment problems offers
opportunities for better decision making that can result in both increased revenue
and decreased lost sales. We present an assignment model incorporating both
individual learning and uncertainty in demand and compare its performance to
several myopic models. Our results demonstrate that cross training the workforce
can be a successful way to hedge against uncertain demand. We further explore
the use of practice assignments and inventory building as ways of creating
capacity and preparing to meet future demand.
5 - Approximation Algorithms For Capacitated Perishable Inventory
Systems With Positive Lead Times
Xiting Gong, Assistant Professor, The Chinese University of Hong
Kong, Room 506, William M. W. Mong Engineering Building,
Shatin N.T., Hong Kong,
xtgong@se.cuhk.edu.hk, Xiuli Chao,
Cong Shi, Chaolin Yang, Huanan Zhang, Sean Zhou
Perishable inventory system with positive lead time and finite ordering capacity is
an important but notoriously difficult class of problems in both analysis and
computation. Its optimal control policy is extremely complicated, and no effective
heuristic policy has been proposed in the existing literature. In this paper, we
develop an easy-to-compute approximation policy for this class of problems and
show that it admits a theoretical constant-factor worst-case performance
guarantee under most demand models of practical interest. Our numerical study
shows that the proposed policy performs consistently well.
TA94
5th Avenue Lobby-MCC
Technology Tutorial: Frontline/ SAS-GAP/EDU
Technology Tutorial
1 - Frontline:
AnalyticSolver.com:Data Mining, Simulation And
Optimization In Your Web Browser
Daniel Fylstra, Frontline Systems, Inc., Incline Village, NV,
Daniel@solver.com AnalyticSolver.comis the new, simple, point-and-click way to create and run
analytic models using only your web browser - that also works interchangeably
with your spreadsheet. Whether you need forecasting, data mining and text
mining, Monte Carlo simulation and risk analysis, and conventional and
stochastic optimization, you can “do it all” in the cloud. We’ll show how you can
upload and download Excel workbooks, pull data from SQL Server databases and
Apache Spark Big Data clusters, solve large-scale models, and visualize results -
without leaving your browser. If you’re more comfortable working on your own
laptop or server, we’ll show how you can do that, too.
2 - SAS: Analysis Of a Presidential Debate Using SAS Text Analytics
André de Waal, Global Academic Program, Cary, NC, 27513,
United States
During the last year of a presidential term in the United States of America, the
race to the White House has everybody excited. News channels and newspapers
provide “expert” analysis of day to day events. However, many of the expert
opinions are biased and reflect a commentator’s political viewpoint or affiliation.
Can text mining be used to look at the data objectively and cut through the
political rhetoric? In this talk, a script of one of the 2016 presidential debates is
analyzed with SAS Text Miner. An attempt is made to look at the data
“objectively” and to let the data speak. Words are counted and stemmed,
documents are grouped into clusters, topics are identified and candidates are
analyzed while trying to determine what separates one candidate from the rest of
the field. Although it is impossible to predict using text mining alone who will
win the presidential election, text mining could provide some insight into the
election process (of which the debates are an integral part) that is not generally
available to the general populace and might influence their choice of presidential
candidate.
TA94