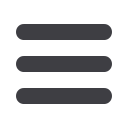

INFORMS Nashville – 2016
333
Tuesday, 3:10PM - 4:00PM
Keynote Tuesday
Davidson Ballroom A-MCC
Optimizing the Future – Supply Chain at Amazon
Keynote Session
Chair: Anne Robinson, Verizon Wireless, Basking Ridge, NJ,
anne.robinson@verizonwireless.com1 - Optimizing The Future - Supply Chain at Amazon
Jason Murray, Amazon, 410 Terry Avenue North, Seattle, WA,
89109, United States,
n.a@na.orgThe retail supply chain of the future will be built on massive data, advanced
analytics and innovative technology. At Amazon, we are constantly pushing the
frontier in each of these areas to help create that future. Our vision is to move
products at an unprecedented scale through the most technologically advanced
supply chain possible, where intelligent optimization algorithms drive efficiency.
To achieve this vision, we focus on three core pillars: research, technology, and
business ownership. We develop new research, implement it in technology, and
own the top- and bottom-line of the business. To be successful, we believe
business ownership, research, and development must be tightly coupled. During
this presentation, I will discuss our vision for the future of retail supply chain —
where we have been, where we are, and where we plan to go. I will share some
cases of research innovation and its integration with technology and business.
These include inter-disciplinary modeling and optimization (from machine
learning, statistics, simulation and optimization) to make Amazon’s supply chain
more efficient. Finally, I will provide examples of some challenges we will need to
overcome to make our vision a reality.
Keynote Tuesday
Davidson Ballroom B-MCC
Wagner Prize Winner Reprise
Invited: Plenary, Keynote
Invited Session
Chair: Allen Butler , Daniel H Wagner Associates, Inc., Hampton, VA,
allen.butler@va.wagner.com1 - Wagner Prize Winner Reprise
C. Allen Butler, Daniel H Wagner Associates, Inc., 2 Eaton Street,
Hampton, VA, 23669, United States,
Allen.Butler@va.wagner.comThe Daniel H. Wagner Prize for Excellence in Operations Research Practice
emphasizes the quality and coherence of the analysis used in practice. Dr. Wagner
strove for strong mathematics applied to practical problems, supported by clear
and intelligible writing. This prize recognizes those principles by emphasizing
good writing, strong analytical content, and verifiable practice successes
Keynote Tuesday
Davidson Ballroom C-MCC
Edelman Reprise-UPS
Keynote Session
Chair: Michael Trick, Carnegie Mellon University, Pittsburgh, PA,
(IFORS President)
trick@cmu.edu1 - UPS Optimizes Delivery Routes
Jack Levis, Director of Process Management, UPS, Timonium, MD,
United States,
jlevis@ups.com, Ranganeth Nuggehalli
UPS, the leading logistics provider in the world, and long known for its penchant
for efficiency, embarked on a journey to streamline and modernize its pickup and
delivery operations in 2003. This journey resulted in a suite of systems, including
an optimization system, which is called “On Road Integrated Optimization and
Navigation” (ORION). Every day, ORION provides an optimized route for each of
UPS’ 55,000 U.S. drivers based on the packages to be picked up and delivered on
that day. The innovative system creates routes that maintain the desired level of
consistency from day to day. To bring this transformational system from concept
to reality, UPS instituted extensive change management practices to ensure buy-
in from both users and executives. Costing more than $250 million to build and
deploy, ORION is expected to save UPS $300 to $400 million annually. ORION is
also contributing the sustainability efforts of UPS by reducing the CO2 emissions
by 100,000 tons annually. By providing a foundation for a new generation of
advanced planning systems, ORION is transforming the pickup and delivery
operations at UPS.
Tuesday, 4:30PM - 6:00PM
TD01
101A-MCC
New Methods in Data Mining
Sponsored: Data Mining
Sponsored Session
Chair: Mariya Naumova, Rutgers University, 640 Bartholomes Road,
Piscataway, NJ, 08854, United States,
mnaumova@rci.rutgers.edu1 - A Fast Algorithm For Discretization In A Big Data Space
Abdelaziz Berrado, Associate Professor, University Mohammed V
in Rabat, BP 765, Avenue Ibn Sina, Agdal, Rabat, 10080, Morocco,
berrado@emi.ac.maDiscretizing continuous attributes is a necessary preprocessing step before
association rules mining or using several inductive learning algorithms with a
heterogeneous big data space. This important task should be carried out with a
minimum information loss. We combine bagging and nonlinear optimization
techniques to build an automated supervised, global and dynamic discretization
algorithm that derives its ability in conserving the data properties from the
Random Forest algorithm. Empirical results indicate the performance of our
discretization algorithm.
2 - Orthogonal Tensor Decomposition
Cun Mu, PhD Student, Columbia University, 500 West 120th
Street, Room 315, New York, NY, 10027, United States,
cm3052@columbia.edu, Donald Goldfarb
Many idealized problems in signal processing, machine learning, and statistics can
be reduced to the problem of finding the symmetric canonical decomposition of
an underlying symmetric and orthogonally decomposable (SOD) tensor. In this
talk, we will address several practical issues arising from conducting this
orthogonal tensor decomposition.
3 - Distance-based Methods For Classification Of Groups Of Objects
Mariya Naumova, Rutgers University, 110 Frelinghuysen Rd.,
Piscataway, NJ, 08854, United States,
mnaumova@rci.rutgers.eduGiven a finite number of learning samples from several populations (groups) and
a collection of samples from the union of these populations, it is required to
classify the entire collection (not a single sample) to one of the groups. Such
problems often arise in medical, chemical, biological and technical diagnostics,
classification of signals, etc. We consider different methods of solving the problem
based on distance formulas and make comparison of their quality based on
numerical results. We give an illustrative example with real data to demonstrate
the effectiveness of the classification methods.
TD02
101B-MCC
Data Mining in Medical and Brain Informatics II
Sponsored: Data Mining
Sponsored Session
Chair: Chun-An Chou, SUNY Binghamton, 4400 Vestal Parkway East,
Binghamton, NY, 13902, United States,
cachou@binghamton.eduCo-Chair: Sina Khanmohammadi, SUNY Binghamton, 4400 Vestal
Parkway East, Binghamton, NY, 13902, United States,
skhanmo1@binghamton.edu1 - Statistical Learning Of Neuronal Functional Connectivity
Chunming Zhang, University of Wisconsin - Madison,
cmzhang@stat.wisc.eduIdentifying the network structure of a neuron ensemble is critical for
understanding how information is transferred within such a neural population.
We propose a SIE regularization method for estimating the conditional intensities
under the GLM framework to better capture the functional connectivity among
neurons. A new algorithm is developed to efficiently handle the complex penalty
in the SIE-GLM for large sparse data sets applicable to spike train data. Simulation
results indicate that our proposed method outperforms existing approaches. An
application of the proposed method to a real spike train data set provides some
insight into the neuronal network.
TD01