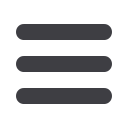

INFORMS Nashville – 2016
335
TD05
101E-MCC
Forest Management
Sponsored: Energy, Natural Res & the Environment II Forestry
Sponsored Session
Chair: Aaron Bradley Hoskins, United States Naval Research
Laboratory, 7220 Briarcliff Drive, Springfield, VA, 22153, United States,
abh318@msstate.eduCo-Chair: Sándor F. Tóth, University of Washington, School of
Environmental & Forest Sciences, Seattle, WA, 9, United States,
oths@uw.edu1 - Stochastic Forestry Planning Problem Using Progressive Hedging
Cristobal Pais, PhD Student, Instituto de Sistemas Complejos de
Ingeniería, Domeyko 2367, Santiago, 8370397, Chile,
cpais@ing.uchile.cl,Andres P Weintraub
The forest planning problem with road construction consists in managing the
production of a land divided into harvest cells. The goal is the maximization of
the expected NPV for a tactical plan subject to uncertainties. A method is applied
for generating scenario trees (price, demand and growth) with their probabilities.
Non-anticipativity constraints are needed in the model to link scenarios. This
problem is difficult to solve due to the number of scenarios. We have
implemented Progressive Hedging which separates the problem by scenarios, with
multiple adjustments to improve its solvability exploiting its parallel
implementation. With this approach, instances up to 1000 scenarios were solved.
2 - Using Critical Component Detection In Graphs For Wildfire
Fuel Management
Dmytro Matsypura, The University of Sydney, Business School,
Abercrombie Building H70, Sydney, 2006, Australia,
dmytro.matsypura@sydney.edu.auWe study the problem of wildfire fuel management. The problem is formulated as
a non-linear optimization problem. We apply the formulations and objectives
used for critical element detection in graphs. We present the results of simulations
and empirical study.
3 - A Stochastic Programming Approach To Satellite
Constellation Design
Aaron B Hoskins, PhD Candidate, Mississippi State University,
P.O. Box 9542, Starkville, MS, 39762, United States,
abh318@msstate.edu, Hugh Medal
A constellation of satellites is launched to collect data on wildfires. The time and
location of the wildfires is not known at the time the satellites are launched into
orbit. This research implements a stochastic programming algorithm to select the
initial constellation design that minimizes the expected cost of maneuvering the
satellites to collect data once the location of the wildfire has been realized.
4 - Optimal Carbon Sampling In Remote Forest Regions
Sándor F. Tóth, University of Washington, School of
Environmental & Forest Sciences, Seattle, WA, 9, United States,
oths@uw.edu,Hans Eric Andersen
We present a spatial optimization approach to find the best integrated ground and
air sampling strategy for carbon in remote boreal forests. We minimize the
expected variance on estimates of the mean carbon tonnage in six forest pools by
optimal flight path selection for remote sensing and by optimal vehicle routing for
ground calibration. We apply the model, which incorporates budgetary and
logistical constraints, to the Tanana District of the U.S. Forest Service in the
Interior of Alaska.
TD06
102A-MCC
Optimization for Large-Scale Learning
Sponsored: Data Mining
Sponsored Session
Chair: Dzung Phan, IBM Research, Yorktown Heights, NY,
United States,
phandu@us.ibm.com1 - Gradient Sliding For Structured Convex Optimization
Yuyuan Ouyang, Clemson University,
yuyuano@clemson.edu,
Guanghui Lan
We present a gradient sliding method for a class of structured convex
optimization. In particular, we assume that the optimization problem has the
structure of the sum of two components. The proposed method is capable of
skipping the evaluation of one of the components in the problem, will preserving
the overall iteration complexity. The proposed method can be applied to smooth
optimization, bilinear saddle point optimization, and variational inequalities.
2 - Storm: Stochastic Optimization Using Random Models
Matthew Joseph Menickelly, Lehigh University,
mjm412@lehigh.eduIn this talk, we will discuss work in developing an algorithmic framework
(STORM) for the unconstrained minimization of a stochastic function, f. The
framework is based on the class of derivative-free trust-region methods. It
essentially requires that both the quality of random models of f and the error in a
pair of point estimates - one at the current iterate and a second at the trial step -
scale with the square of the trust region radius. We compare this approach to
usual methods of stochastic optimization, e.g. stochastic gradient descent, and
discuss possible applications of STORM in hyperparameter tuning and AUC
optimization.
3 - Extended Gauss-Newton-Type Algorithms For Low-rank
Matrix Optimization
Quoc Tran-Dinh, University of North Carolina at Chapel Hill,
quoctd@email.unc.eduWe develop a Gauss-Newton framework for solving nonconvex optimization
problems involving low-rank matrix variables. The algorithm inherits advanced
features from classical Gauss-Newton method to this extension such as local
linear and quadratic convergence. Under mild assumptions, we prove the local
linear and quadratic convergence of our
method.Byincorporating with a
linesearch, the algorithm has a global convergence guarantee to a critical point of
problems. As a special case, we customize our framework to handle the
symmetric case with provable convergence guarantees. We test our algorithms on
various practical problems including matrix completion, and quantum
tomography.
4 - Projection Algorithms For Nonconvex Minimization With
Application To Sparse Principal Component Analysis
Dzung Phan, IBM Research,
phandu@us.ibm.com, William Hager,
Jiajie Zhu
We minimize a concave function over nonconvex sets, and propose a gradient
projection algorithm (GPA) and an approximate Newton algorithm (ANA).
Convergence results are established. In numerical experiments arising in sparse
principal component analysis, it is seen that the performance of GPA is very
similar to the fastest current methods. In some cases, ANA is substantially faster
than the other algorithms, and gives a better solution.
TD07
102B-MCC
Emerging Topics on Internet of Things (IoT)
and Data Analytics
Sponsored: Data Mining
Sponsored Session
Chair: Chen Kan, Pennsylvania State University, University Park, PA,
United States,
cjk5654@psu.edu1 - A Pomdp Approach For Optimal Alerting To Remotely Monitored
Asthma Patients Considering Alert Compliance Issue
Junbo Son, Assistant Professor, University of Delaware, Newark,
DE, United States,
sonjunbo@gmail.com,Shiyu Zhou
Driven by the IoT, a smart asthma management (SAM) system has been
implemented where rescue inhalers with a wireless connection record the inhaler
usage and transmit the data to a centralized server. Based on the diagnosis result,
the system may alert the patient for timely interventions. A crucial challenge is to
decide when to alert the patients considering the fact that the patient may not
comply the alert. This alert compliance issue complicates the decision process. In
this research, a novel partially observable Markov decision process considering
alert compliance is proposed and useful insights were found which would benefit
both the asthma patients and company who run the SAM system.
2 - Integration Of Data-level Fusion Model And Kernel Methods For
Degradation Modeling And Prognostic Analysis
Changyue Song, University of Wisconsin-Madison, Madison, WI,
United States,
csong39@wisc.edu,Kaibo Liu
Internet of things has enabled a data-rich environment with multiple sensors to
monitor the degradation process of a unit in real time. As each sensor signal often
contains partial and dependent information, data-level fusion methods have been
developed that aim to construct a health index via the combination of multiple
sensor signals. The existing data-level fusion methods are limited by only
considering a linear fusion function, which may be insufficient to accurately
characterize the complex relations of sensor signals in practice. This study fills the
literature gap by integrating the kernel methods with data-level fusion
approaches to incorporate nonlinear fusion functions.
TD07