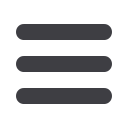

INFORMS Nashville – 2016
458
2 - Detecting Node Propensity Changes In Dynamic Degree-
corrected Stochastic Block Model
Lisha Yu, City University of Hong Kong, Hong Kong,
lishayu2-c@my.cityu.edu.hk,Kwok-Leung Tsui
Many real-world data can be represented as dynamic networks which are the
evolutionary networks with timestamps. Studying the evolution of node
propensity over time is significant to exploring and analyzing networks. In this
paper, we propose a multivariate surveillance plan to monitor node propensity in
dynamic social networks based on the degree-corrected stochastic block model
(DCSBM). Experiments on simulated and real social network streams
demonstrate that our surveillance strategies can efficiently detect different types
of node propensity change in dynamic DCSBM with different kinds of community
structure.
WD08
103A-MCC
Dynamic Prog/Control
Contributed Session
Chair: Xiaodong Luo, Sabre Holdings Inc, 3150 Sabre Drive,
Southlake, TX, 76092, United States,
Xiaodong.Luo@sabre.com1 - Adoptive Vehicle Cruise Control Using Real Time Data And A
Dynamic Programming Model: A Web Based Application
Mohammad Ali Alamdar Yazdi, PhD Student, Auburn University,
354 W Glenn Ave, Auburn, AL, 36830, United States,
mza0052@auburn.edu, Fadel Mounir Megahed
Recent investigations have included exploring the benefits of autonomous vehicle
systems in improving a vehicle’s miles per gallon (mpg) fuel-economy
performance. This talk examines a new direction where large amounts of online
data will be used to develop a fuel efficient cruise controller. More specifically, a
dynamic programming model is constructed to capitalize on existing fuel
consumption models and use real-time data collected from different Google APIs
to minimize fuel efficiency. The minimization is based on dynamically optimizing
the current speed and route based on forthcoming route conditions (traffic,
elevation, etc.).
2 - Modeling Quality Of Care In Hospice Operations
Leela Nageswaran, Tepper School of Business,
5000 Forbes Avenue, Pittsburgh, PA, 15213, United States,
lnageswa@andrew.cmu.edu,Alan Scheller-Wolf, Aliza R Heching
We study a hospice manager’s problem of controlling quality of care in light of
recent regulatory changes mandating reporting of quality metrics. To explore the
potential effects of such reporting, we develop an analytical Markov Decision
Process model that incorporates how staffing - a primary determinant of quality -
affects the rate at which patients join the hospice (due to quality reputation
effects) and depart the hospice (due to quality of care effects). This in turn affects
the hospice’s revenues and costs. We solve our model to obtain properties and
insights related to the optimal quality control policy.
3 - Decision Facing Ambiguity MDP POMDP And Beyond
Mohammad Rasouli, University of Michigan, 430 South Fourth
Ave, Ann Arbor, MI, 48104, United States,
rasouli@umich.eduWhile most of the decision making tools are developed for a Bayesian framework
where the decision maker knows full stochastic description of uncertainties in the
environment, decision facing ambiguity (model uncertainty and non-stochastic
uncertainty) is a better approach for modeling a lot of practical situations. We
discuss how decision making tools including MDP, POMDP, learning (e.g. Multi-
armed bandit) and team decision making can be extended for environments with
ambiguity. We discuss robustness and bounded rationality in this framework.
4 - Iterative Methods For Large Markov Decision Problems
Xiaodong Luo, Sabre Holdings Inc, 3150 Sabre Drive,
Southlake, TX, 76092, United States,
Xiaodong.Luo@sabre.comWe propose a new unified LP formulation for the Infinite Horizon Markov
Decision Problem (MDP), both with the total discounted reward criteria and the
long-run expected average reward criteria (assuming the gain is constant). We
embed a column generation scheme into a multiplier method to solve the new
formulation. Our algorithm can solve large randomly generated MDPs faster than
commercial solvers. It scales up linearly for MDPs with hundreds of millions of
nonzero. It uses much less memory than Barrier method, easy to warm start and
is highly parallelizable.
WD09
103B-MCC
Spatial Optimization and Conservation
Reserve Design
Sponsored: Energy, Natural Res & the Environment I
Environment & Sustainability
Sponsored Session
Chair: Bistra Dilkina, Georgia Institute of Technology, 266 Ferst Drive,
Klaus Bldg 1304, Atlanta, GA, 30332-0765, United States,
bdilkina@cc.gatech.edu1 - Density Based Design: A Spatial Optimization Model For
Protecting The Fisher
Richard Church, University of California, Santa Barbara,
church@geog.ucsb.eduWe discuss the necessary elements of home range core areas in supporting female
fishers during the key natal-maternal season. We propose an integer linear
programming model that schedules harvests and spatially tracks and meets
needed habitat elements over time and present preliminary results for an
industrial forest in California.
2 - Optimizing Conservation Designs With Home Range And
Connectivity Criteria
Bistra Dilkina, Georgia Institute of Technology, Atlanta, GA,
United States,
bdilkina@cc.gatech.eduWe develop a wildlife reserve design approach that takes into account both the
number of individuals supported and the accessibility of the whole design to those
individuals. In particular, a spatial capture-recapture model based on ecological
resistance distance gives rise to three estimated quantities of interest (density,
potential connectivity and density-weighted connectivity) that can be used to
evaluate the ability of a reserve design to support individuals. We formulate a set
of optimization problems to examine the use of these three quantities for
selecting land parcels for conservation.
3 - Delaying Invasive Spread: Is Effective Control Possible Without
Effective Prediction?
Gwen Spencer, Smith College,
gwenspencer@gmail.comSome (continuous) models of species spread yield impossibly-clean analytical
results. Productive mathematical exchange with ecologists must acknowledge and
attempt to capture disciplinary knowledge and critiques, even if this means
sacrificing analytical traction. We will discuss computational work motivated by
experimental and statistical papers in the invasive-species literature, making the
case for discrete methods that acknowledge landscape heterogeneity and
objectives that go beyond expected value.
4 - Avicaching: A Two Stage Game For Bias Reduction In
Citizen Science
Yexiang Xue, Cornell University,
yexiang@cs.cornell.eduThe data collected in citizen science projects are often biased, more aligned with
the citizens’ preferences rather than scientific objectives. We introduce a novel
game for reducing the data bias in which the organizer, a citizen-science program,
incentivizes the agents, the citizen scientists, to visit under-sampled areas. We
provide a novel way to encode this two-stage game as a single optimization
problem, cleverly folding the agents’ problems into the organizer’s problem. We
apply our methodology to eBird, a well-established citizen science program, as a
game called Avicaching. Since its deployment, Avicaching has been very
successful, surpassing the expectations of the eBird organizers.
WD11
104A-MCC
Various Aspects of Second Order Cone Optimization
Sponsored: Optimization, Linear and Conic Optimization
Sponsored Session
Chair: Sertalp Bilal Cay, Lehigh University, 200 W Packer Ave,
Bethlehem, PA, 18015, United States,
sertalpbilal@gmail.com1 - On Disjunctive Conic Cuts When They Exist When They Cut
Mohammad Shahabsafa, Lehigh University, 200 West Packer Ave,
Bethlehem, PA, Bethlehem, PA, 18015, United States,
mos313@lehigh.edu, Tamas Terlaky
The development of Disjunctive Conic Cuts (DCCs) for MISOCO problems has
recently gained significant interest in the optimization community. Identification
of cases when DCCs do not exist, or are not useful, saves computational time. In
this study, we explore cases where either the DCC methodology does not derive a
DCC which is cutting off the feasible region, or a DCC does not exist. Additionally,
we work on extending the DCCs to other conic optimization problems such as
Mixed Integer p-order Cone Optimization and Mixed Integer Semidefinite
Optimization.
WD08