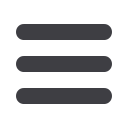

INFORMS Nashville – 2016
460
3 - A Study on Optimal Locations Of Public Facilities To Maximize
Social Benefit”
Hyunhong Choi, PhD Student, Seoul National University,
1 Gwanak-ro Gwanak-gu, Seoul, 08826, Korea, Republic of,
hongchoi@snu.ac.kr, Misuk Lee, Yoonmo Koo
Economic feasibility analysis on the public facility construction is widely being
studied. However, studies concerning the optimal location of these facilities has
not been fully explored yet. In this study, we estimated consumers’ willingness to
pay depending on the distance to non-market goods(i.e., arboretums) by using
the contingent valuation method. Then, we utilized nested partitions method, one
of the categorical optimization methods, to find optimal locations maximizing
social benefit among numerous alternatives.
4 - Location Determination Of A Milk Condensing Plant In Tennessee
David Mendez, Graduate Student, University of Tennessee,
2621 Morgan Circle, Knoxville, TN, 37996, United States,
dmendez@vols.utk.eduGiven the increasing popularity of local foods and the desire to reduce shipping
costs and carbon footprint, Tennessee-based dairy product processors are likely
interested in sourcing condensed milk from an in-state milk condensing plant.
Based on dairy farmer surveys, distances, and transportation costs, this study uses
a mixed integer linear programming model to determine the optimal location for
a condensing plant that minimizes transportation costs from farms to the
condensing plant and the condensing plant to further processors.
WD15
104E-MCC
Intelligent Information Systems
Sponsored: Artificial Intelligence
Sponsored Session
Chair: Victor Benjamin, University of Arizona, 1130 E Helen St, Tucson,
AZ, 85719, United States,
vabenji@email.arizona.edu1 - Using Big Data And Analytics To Enable Smart Mobility
Yun Wang, University of Arizona, Tucson, AZ, 85716, United
States,
yunw@email.arizona.edu, Faiz Currim, Sudha Ram
In this work we introduce a three-layer management system to support smart
urban mobility with an emphasis on bus transportation. In Layer-1, we apply
novel Big Data techniques to efficiently compute bus travel times and passenger
demands using universal data streams. Layer-2 contains two analytic components:
network analysis of passenger transit patterns and causal relationship analysis for
bus delays. The third layer provides interactive visualization tools for decision
support. Our system is developed in cooperation with the city of Fortaleza in
Brazil. The use of generally available urban transportation data makes our
methodology adaptable and customizable for other cities.
2 - Analyst Language In Quarterly Earnings Calls: Comparing
Interactions With Fraudulent And Non-fraudulent Managers
Lee Spitzley, University of Arizona,
lspitzley@email.arizona.eduCorporate financial fraud damages investors, the public, and the companies
involved. Fraudulent managers must convince investment analysts who study the
company that what they say is accurate and represents the true state of the
business. I will examine the content of analyst utterances when they are
interacting with the managers during earnings calls. If analysts suspect
abnormalities, they may modify their questioning strategies. This study will test
for differences in the topic composition of all analysts on a call, and for differences
within analysts who follow both fraudulent and non-fraudulent companies in the
same industry.
3 - An Empirical Study Of Venders’ Profit Under Different
Mechanisms On Online Crowdsourcing Platforms
Xiao Han, Shanghai Jiao Tong University, Shanghai, China,
hanxiao@sjtu.edu.cn,Pengzhu Zhang
Online crowdsourcing markets not only allow buyers of any size to tap into a
large talented pool of workforce but also expand the market reach for vendors.
Prior studies on crowdsourcing markets have mainly focused on buyers, and there
is a lack of understanding of how vendors survive and evolve in the
crowdsourcing markets. This paper aims to fill this gap by taking the perspective
of vendors and ask how vendors benifit in crowdsourcing markets under different
mechanisms.
4 - The Dark Side Of The Singularity: Can OR/MS Help?
John D Little, Massachusetts Institute of Technology, Sloan School
of Management, Room E62-534, Cambridge, MA, 02142,
United States,
jlittle@mit.eduThe “Singularity” is the point in time when artificial intelligence (AI) exceeds
human intelligence. This may occur by putting AI on computers, by biological
creation, or by a mixture of both. Some of the people writing about this or
developing advanced AI are Victor Vinge, Ray Kurzweil (the Singularity is Near),
Tom Malone, Ben Goertzel and Hugo de Garis. The dark side is that most people
in this room will be left far behind. Kurzweil notes that AI develops
exponentially, whereas most of us extrapolate linearly.
WD16
105A-MCC
Optimization and Learning in Urban Delivery
Sponsored: Optimization, Optimization Under Uncertainty
Sponsored Session
Chair: Lei Zhao, Tsinghua University, Qinghua West Road, Beijing,
100084, China,
lzhao@tsinghua.edu.cn1 - Robust Inventory Management Under Supply And
Demand Uncertainties
Jie Chu, McMaster University, Hamilton, ON, Canada,
chuj6@mcmaster.ca,Kai Huang
We simultaneously consider demand and supply uncertainties in a robust
optimization framework. We first consider a single-station case, then we extend
to a multi-echelon network case. The resulting robust counterpart of the network
case does not maintain the same difficulty as its nominal problem. Nonetheless,
we present an approximation and thus it can be solved more efficiently. We
demonstrate the effectiveness of proposed models numerically.
2 - Learning In Multi-stage Rollouts
Saul Toscano-Palmerin, Cornell University, Ithaca, NY,
United States,
st684@cornell.edu, Peter Frazier
We consider a transportation company choosing routes on which to offer service.
Each route has an unknown parametric demand distribution, and we wish to
choose routes subject to a budget constraint to maximize total demand. We
propose a two-stage optimal learning algorithm, where first we offer service on
some routes, and learn from observed demand about demand distributions on
other similar routes. Then in a second stage, we offer service on additional routes
suggested to be good by the first stage. We demonstrate that this two-stage
optimal learning approach captures more demand than a one-stage approach that
does not leverage the opportunity to learn.
3 - Optimal Learning In Urban Delivery Resource Allocation
Yixiao Huang, Tsinghua University, 530 Shunde Building, Beijing,
100084, China,
huangyx12@mails.tsinghua.edu.cn, Lei Zhao,
Warren B Powell, Ilya O Ryzhov
We study knowledge gradient (KG) based optimal learning methods to optimize
the urban delivery resource allocation decisions, when the evaluation of such
decisions is expansive.
WD17
105B-MCC
Nonlinear Optimization Algorithms II
Sponsored: Optimization, Nonlinear Programming
Sponsored Session
Chair: Daniel Robinson, Johns Hopkins University,
3400 N. Charles Street, Baltimore, MD, 21218, United States,
daniel.p.robinson@gmail.comCo-Chair: Frank Edward Curtis, Lehigh University, 27 Memorial Dr,
Bethlehem, PA, 18015, United States,
frank.e.curtis@gmail.comCo-Chair: Andreas Waechter, Northwestern University,
2145 Sheridan Road, Evanston, IL, 60208, United States,
waechter@iems.northwestern.edu1 - A Geometry Driven Active-set Method For
Elastic-net Minimization
Daniel Robinson, Johns Hopkins University,
daniel.p.robinson@gmail.comWe propose an efficient and provably correct active-set based algorithm for
solving the elastic net problem. The proposed algorithm exploits the fact that the
nonzero entries of the elastic net solution fall into an oracle region, which we use
to define and efficiently update an active set. The proposed update rule leads to
an iterative algorithm which is shown to converge to the optimal solution in a
finite number of iterations. We present experiments on computer vision datasets
that demonstrate the superiority of our method in terms of both clustering
accuracy and scalability.
WD15