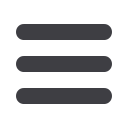

INFORMS Nashville – 2016
508
2 - Resource Optimization Based On Data Envelopment Analysis -
Dynamic Programming Method
Tao Du, Beijing Insititute and Technology, Beijing, China,
dutao0608@163.comAs the resource optimization is one of main approach to improve organization’s
efficiency, we propose a DEA-Dynamic Programming (DEA-DP) method which is
a resource optimization method combining with the DEA model for measuring
efficiency and the Dynamic Programming method for multistage decision. The
basic ideas of the method is to determine the optimal resource planning strategy
for improving the inter DMUs’ relative efficiency of organization through
Dynamic Programming method. Through a case study, we proves investment can
be saved by 11.23% using the method.
WE77
Legends E- Omni
Opt, Large Scale III
Contributed Session
Chair: Tao Jiang, Purdue University, 403 W. State Street, Krannert
School of Management, West Lafayette, IN, 47906, United States,
taujiang@purdue.edu1 - Robust Principal Component Analysis In Multiclass
Problem Structures
Sam Davanloo, Ohio State University, Columbus, OH,
United States,
sdt144@vt.edu, Xinwei Deng
Robust Principal Component Analysis (RPCA) is mainly used to decompose a data
matrix to a low rank and a sparse component, and has applications in face
recognition, video surveillance, latent semantic indexing, and etc. In this study,
we consider a multiclass structure in which classes share a common low rank
component, a class-specified low rank component, and a class-specific sparse
component. RPCA is then utilized to estimate these components. A first-order
optimization method is proposed to solve the problem in high-dimensional
settings. Numerical simulation results support the proposed methodology.
2 - Stability Of The Stochastic Gradient Method For Approximated
Large Scale Kernel Machine Using Random Fourier Features
Aven Samareh, PhD Student, University of Washington, 4324 8th
ave NE, D7, Seattle, WA, 98105, United States,
asamareh@uw.edu,
Mahshid Salemi Parizi
We measured the stability of stochastic gradient method (SGM) for learning an
approximated Fourier support vector machine. The stability of an algorithm is
considered by measuring the generalization error in terms of the absolute
difference between the test and the training error. Our problem is to learn an
approximated kernel function using random Fourier features for binary
classification data sets via online convex optimization settings. We showed that
with a high probability SGM generalize well for an approximated kernel under a
convex, Lipschitz continuous and smooth loss function given reasonable number
of iterations. We empirically verified the theoretical findings as well.
3 - Modeling Improvements And Refinements To The Fleet
Modernization Capability Portfolio Analysis Tool
Frank Muldoon, Sandia National Labs, 1525 Summit Hills Drive
NE, Albuquerque, NM, 87112, United States,
fmmuldo@sandia.gov,Matthew Hoffman, Stephen Henry,
Lucas Waddell, Peter Backlund
The Capability Portfolio Analysis Tool (2015 Edelman Finalist) is currently being
used to model both the fleet of ground combat systems under the U.S. Army PEO
Ground Combat Systems and the fleet of logistics and support systems under PEO
Combat Support & Combat Service Support to provide analytical capability in
support of modernization and investment decisions. This large-scale multi-phase
MILP has evolved over the last year to meet the challenges posed by both PEOs
including the incorporation of budgetary earmarks, system age, and modeling
techniques developed to mitigate its large size.
4 - An Iterative Rounding Algorithm And Almost Feasibility For
Nonconvex Optimization
Tao Jiang, Purdue University, 403 W. State Street, Krannert School
of Management, West Lafayette, IN, 47906, United States,
taujiang@purdue.edu, Thanh Nguyen, Mohit Tawarmalani
We consider a class of high-dimensional non-convex minimization problems for
which the objective is separable and constraints are linear. We solve a convex
relaxation to obtain a lower bound and to restrict the original problem to an
integer program. Then, we study the trade-off in approximation gap between
admitting solutions that violate some constraints slightly versus not allowing such
solutions. In particular, we show that if we admit solutions that “almost” satisfy
the constraints with small coefficients, the iterative rounding procedure can find a
solution much closer to the relaxation bound than if we disallow such solutions.
We discuss applications of this result in various settings.
WE78
Legends F- Omni
Opt, Linear Programing
Contributed Session
Chair: Joseph L Trask, North Carolina State University, 3500 Mill Tree
Rd, Apt B2, Raleigh, NC, 27612, United States,
jltrask@ncsu.edu1 - Optimal Assignment Of Inspection Stations In A Flowline
Md Shahriar Jahan Hossain, Louisiana State University, 2508
Patrick F. Taylor Hall, Baton Rouge, LA, 70803, United States,
msjhossain1@gmail.com,Bhaba R Sarker
The research deals with multiple in-line inspection stations that partition a
production flowline into multiple flexible lines. A unit cost function is developed
for determining the number and locations of in-line inspection stations along
with the alternative decisions on each type of defects: scrapping or reworking
(on/off-line). The problem is formulated as fractional mixed-integer nonlinear
programming problem to minimize the unit cost of production, and solved with
branch and bound method. A construction heuristic is also developed to
determine a sub-optimal solution for large instances.
2 - The Double Pivot Simplex Method
Fabio T Vitor, Graduate Teaching Assistant, Kansas State
University, 2061 Rathbone Hall, 1701B Platt St., Manhattan, KS,
66506, United States,
fabioftv@k-state.edu,Todd W Easton
The Simplex Method (SM) is considered one of the top 10 algorithms of the 20th
century. Each iteration of SM pivots between basic feasible solutions by
exchanging one nonbasic variable with a basic variable. This talk presents the
Double Pivot Simplex Method (DPSM), which can pivot on two variables. The
Slope Algorithm is a new method that replaces the ratio test of SM, and
guarantees the optimal basis at every iteration of DPSM. Furthermore, each
iteration of DPSM not only has the same theoretical running time as SM, but also
improves the objective value by at least as much as an iteration of SM.
Computational experiments demonstrate that DPSM is approximately 15% to
40% faster than SM.
3 - Inverse Optimization For Utility Measurement
Yu-Ching Lee, National Tsing Hua University, Hsinchu, Taiwan,
ylee77@illinois.edu,Yi-Hao Huang, Ciou Si-Jheng
Utility function has been prevalent to express one consumer’s preference
representing consumer’s demand. We formulate a mathematical program with
quadratic objective function and complementarity constraints as the inverse
problem that minimizes the error of the measured utility function. Our research
indicates that the program with complementary constraints will help us find a set
of more accurate parameters.
4 - Determining The Aggregate Plan: A Cross-functional Perspective
Kathleen Iacocca, Villanova University, Villanova, PA, United
States,
kathleen.iacocca@villanova.edu, Kingsley Gnanendran
The traditional aggregate plan is extended to include marketing and financial
aspects. On the marketing side, we determine the optimal price and demand for
each period, while on the financial side we include month-by-month collections,
taxes, interest on loans and/or return on surplus funds, depreciation, and
minimum cash balances in determining optimal production levels. The problem is
modeled as a linear program and implemented on a spreadsheet to demonstrate
ease of managerial applicability.
WE80
Broadway E- Omni
Retail Mgt II
Contributed Session
Chair: H. Sebastian Heese, EBS University, ISCM, Burgstr. 5,
Oestrich-Winkel, 65375, Germany,
sebastian.heese@ebs.edu1 - Consumer Perceptions Of Return Policies
Yue Cheng, Pennslyvania State University, 460A Business
Building, University Park, PA, 16802, United States,
yuc190@psu.edu, Daniel Guide, Margaret Meloy
This study investigates consumer perceptions of three types of return policies by
using survey data. The consumers responded to the amount of discounts and
premiums associated with different return policies and brand equity. We provide
managerial insights for firms to take advantages of using different return policies
strategically.
WE77