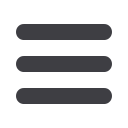

S234
ESTRO 36 2017
_______________________________________________________________________________________________
the EPDC constraint tolerance is adjusted gradually until
a desired PDC is met.
We have tested our probabilistic planning method based
on datasets containing multiple imaging for four cervical
cancer patients treated with VMAT (2 Gy, 23fx). The
datasets formed the basis for a statistical shape model
(SSM) that provided the scenario specific sampled
deformations. A set of 100 scenarios sampled from the SSM
was included in the probabilistic optimization. A final
iteration using 400 scenarios was performed to increase
the resulting precision. A set of 1000 independent
scenarios not part of the optimization was used to verify
that the requested PDC was met.
Results
For all patients in this work, the iterative process of
finding the EPDC tolerance to fulfil the requested PDC
converged in less than 10 iterations to within 0.1 Gy of the
requested PDC (95% of 46 Gy = 43.7 Gy), see figure 1. The
verification calculations showed that the requested PDC
was met within 1.3%, see table 1.
Figure 1.
The convergence of
D
98%,90%
per iteration towards
the requested indicated by the dashed line. A full
probabilistic optimization is performed per iteration.
Table 1.
The D
98%,90%
after optimization and verification
calculations.
Patient
1
PDC optimization
[Gy]
PDC verification
[Gy]
1
43.4
43.2
2
43.8
43.8
3
43.4
43.4
4
43.1
43.1
Conclusion
We proved that a probabilistic planning algorithm can be
formulated such that the dose planner can request a PDC
which the algorithm attempts to fulfil. Results for datasets
of four cervical cancer patients indicate that the
requested PDC was fulfilled within 1.3%.
OC-0446 A Fully Automated VMAT Planning System
with Site-Configurable Algorithm
M. Chu
1
, R. Maggs
1
, M. Smyth
1
, R. Holmes
1
, D.G. Lewis
1
,
J. Staffurth
2
, E. Spezi
3
, A.E. Millin
1
, P.A. Wheeler
1
1
Velindre Cancer Centre, Medical Physics, Cardiff,
United Kingdom
2
Cardiff University, School of Medicine, Cardiff, United
Kingdom
3
Cardiff University, School of Engineering, Cardiff,
United Kingdom
Purpose or Objective
One of the key benefits to automation of the treatment
planning process is that consistency in plan quality can be
maintained, regardless of user experience. To ensure that
the plans are fully optimal, however, the system should
allow incorporation of clinical experience and knowledge
of the oncologist. This work presents an automated
planning system that can be configured via a novel Pareto
navigation process. A retrospective study was performed
with thirty patients across three sites: Prostate & Seminal
Vesicles (PSV), Prostate & Pelvic Nodes (PPN) and Head &
Neck (HN).
Material and Methods
A fully automated VMAT planning system has been
developed using the scripting functionality of RayStation
(RaySearch Laboratories, Stockholm, Sweden). For each
treatment site, a set of clinical priorities is determined as
a list of constraints and ‘tradeoffs’. The system is designed
to ensure constraints are met while optimization of the
prioritized tradeoffs is guided by an
a priori
calibration
process. This process involves optimizing for the first
priority trade-off with a range of objective weights. A GUI
allows the user to navigate through these plans using the
DVH and dose-distribution to determine the optimal
weight. This weight is then stored and the process is
repeated for the next priority. When all trade-offs have
been optimized, the whole process is repeated to refine
the set of objective weights.
As the underlying automated-planning algorithm tailors
the base plan to individual patient anatomy, a single set
of configuration data can be used for all patients of a given
site and plan can be generated with no user interaction.
Ten patients of each configured site were planned using
the automated system and compared against the clinically
approved manual plan. Quantitative comparisons were
made using relevant DVH metrics and qualitative
comparisons of dose distributions.
Results
A selection of the DVH metrics, averaged across all
patients in each site, is listed in Table 1. A set of
representative dose distributions is provided in Figure 1.
The tabulated data shows that the automated plans tend
towards improved OAR sparing. For PSV and PPN, this was
at the expense of target coverage. For HN plans, the
automated plans improved coverage while also reducing
OAR doses.
Visual comparisons of dose distributions showed that, for
all three sites, the automated plans were of equal or
better quality relative to manually optimized plans. All
plans met local clinical DVH constraints and were deemed
to be clinically acceptable.