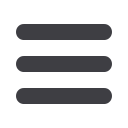

S233
ESTRO 36 2017
_______________________________________________________________________________________________
OC-0444 Pareto-optimal plans as ground truth to
validate a commercial knowledge-based DVH-
prediction system
E. Cagni
1
, A. Botti
1
, Y. Wang
2
, M. Iori
1
, S.F. Petit
2
, B.J.
Heijmen
2
1
Arcispedale S. Maria Nuova - IRCCS, Medical Physics
Unit, Reggio Emilia, Italy
2
Erasmus MC Cancer Institute, Department of Radiation
Oncology, Rotterdam, The Netherlands
Purpose or Objective
The purpose of the current study was two fold. First, to
evaluate the DVH prediction accuracy of RapidPlan (Varian
Medical Systems, Palo Alto) using a large database of
Pareto optimal treatment plans. These were consistently
generated with automated prioritized multi-criterial
treatment plan optimization, independent of RapidPlan,
and therefore can be considered as an unbiased, ground
truth of achievable plan quality. Second, to determine the
importance of the size/variability of the plan database on
the accuracy of the RapidPlan DVH predictions. Using the
automatically generated plans, the prediction accuracy of
RapidPlan could be investigated without an impact of
unavoidable plan quality variations related to manual
planning.
Material and Methods
A previously published database of 115 Pareto-optimal
prostate VMAT plans, consistently generated with
automatic prioritized planning, was used in this study
[Wang et al, PMB, 2016]. Separate Rapidplan prediction
models were generated for training groups consisting of
20, 30, 45, 55, or 114 randomly selected plans, the latter
using a leave-one-out technique. In a second experiment,
Model-20 was also built for 4 other groups of randomly
selected training patients. Prediction accuracy of all
models was assessed using a fixed, independent validation
group of 60 plans and comparing predicted dose
parameters (rectum Dmean, V65, and V75, anus Dmean,
and bladder Dmean) with the achieved values of the
Pareto optimal plans.
Results
For Model-114, the absolute (relative) prediction errors
(mean±SD), for rectum Dmean, V65, and V75 were
1.8±1.4Gy (6.9±5.6%), 1.0±0.9% (8.9±13.4%), and 1.6±1.4%
(36.3±62.2%), respectively. For anus and bladder Dmean,
these errors were 2.2±1.7Gy (18.3±21.3%) and 1.8±1.3Gy
(4.9±4.2%), respectively. For 63.3% of the validation plans,
Model-114 predicted a lower rectum V65 than could
actually be achieved. Because of the prioritized
optimization used for generating the input Pareto-optimal
plans, this can only be realized by underdosing the target.
In 36.7% of plans, the predicted V65 was higher than
obtained in the input plan, possibly losing an opportunity
for lower rectum dose.
Table 1
demonstrates equal
prediction accuracies for model-114 and the smaller
models (only first Model-20 included).
Table 2
compares
Model-114 with all 5 investigated Models-20, showing
significant differences in performance of the Models-20.
Conclusion
Rapidplan DVH prediction is a useful tool to guide
treatment plan generation, although significant prediction
inaccuracies may occur, even when the training database
consists of as many as 114 treatment plans. Since all plans
to train and validate RapidPlan DVH prediction were
Pareto optimal, prediction errors for a database of
manually optimized treatment plans are likely larger than
presented here. For models based on a small number of
plans (N=20), prediction performance depends strongly on
the selected training patients, therefore larger models are
recommended.
OC-0445 Probabilistic optimization of the dose
coverage – applied to treatment planning of cervical
cancer
D. Tilly
1,2
, A. Holm
2
, E. Grusell
1
, A. Ahnesjö
1
1
Uppsala University Hospital, Department of
Immunology- Pathology and Genetics, Uppsala, Sweden
2
Elekta, R&D, Stockholm, Sweden
Purpose or Objective
Probabilistic optimization is an alternative to margins for
handling geometrical uncertainties in treatment planning
of radiotherapy where the uncertainties are explicitly
incorporated into the plan optimization through sampling
of treatment scenarios and thereby better exploit patient
specific geometry. In this work, a probabilistic method is
presented based on statistical measures of dose coverage,
similar to the basis for margin based planning. The idea is
that the dose planner requests a dose coverage to a
specified probability, which the algorithm then fulfils.
Material and Methods
The van Herk margin recipe is designed to deliver
sufficient target dose coverage in 90% of the treatments.
The probability is however rarely specified. We generalize
this prescription approach to include the probability
explicitly through the concept of
Percentile Dose
Coverage
(PDC), i.e. the dose coverage that is at least
fulfilled to a specified probability. The PDC used in this
work for target minimum dose criterion is the probability
for the dose-volume criteria
D
98%
to be fulfilled with 90%
probability
which
we
denote
as
D
98%,90%
.
For optimization, we make use of the
Expected Percentile
Dose Coverage
(EPDC), defined as the average dose
coverage below a given PDC. The EPDC is, in contrast to
PDC, a convex measure which allows for standard
optimization techniques to be used for finding an optimal
treatment plan. We propose an iterative method where a
treatment optimization is performed at each iteration and